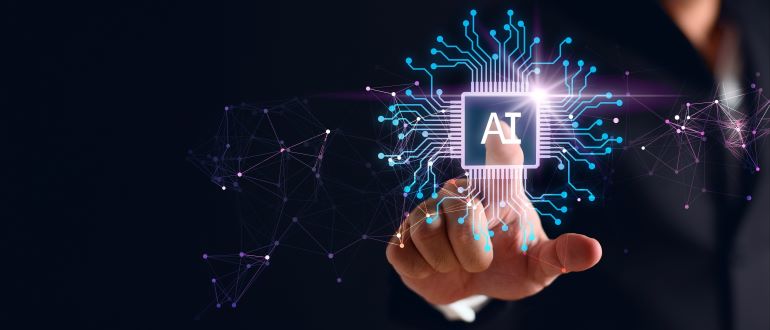
The artificial intelligence (AI) revolution has been on the horizon for decades but has arrived resoundingly in the past five years. Advancements in machine learning algorithms, added computing power and widespread availability of big data have contributed to AI’s impact in most areas of business and everyday life. Additionally, deep learning techniques create a favorable environment for natural language processing and speech recognition breakthroughs.
Applying these advances responsibly requires a growing emphasis on ethical considerations in AI. Further exploration into bias, fairness, transparency and accountability has led to the development of frameworks and guidelines for creating responsible AI.
Identifying Bias and Ensuring Fairness
Implementation of responsible AI requires close consideration of technical, social and ethical factors. From a technical perspective, algorithmic performance is the biggest factor in determining the AI engine’s accuracy, speed and scalability. Data quality requires impeccable model training to ensure accuracy and free the information from inherent biases. Privacy and security measures protect systems from cyberthreats that may compromise data integrity—or worse.
The most significant social consideration is AI’s impact on the workforce. Enhanced automation poses the threat of job displacement, so the emphasis becomes skill development for future employment—strategizing for upscaling and staying abreast of the latest trends in AI. Collaboration between humans and AI systems requires recognition of the strengths and weaknesses of both sides and developing strategies to work together.
Ethically, bias mitigation and detection are at the forefront to ensure fairness and prevent discrimination against any group. The key to maintaining transparency in development and deployment is creating a chain of command—ultimately, determining who is responsible for ensuring ideal outcomes. The ACM Code of Ethics and Professional Conduct and IEEE Ethically Aligned Design standards provide outstanding starting points for adhering to or establishing an ethical code.
Incorporating Human Oversight of AI
Data and model training development are robust, so it’s critical for the results to be analyzed equally to remove biases. Analysts must ask tough questions, such as whether any groups are over- or under-represented in the data training set. Bias detection tools are indispensable in projecting, assessing and improving fairness in numerous models through the detection of demographic disparities and equal opportunity.
Removal or mitigation of bias is a perfect example of a human’s role in working together with AI systems. Data pre-processing methods suggest the employment of bias mitigation via algorithmic adjustments, in addition to ensuring optimal diversity in the training data set through representation of each group, geographical region, and other demographic considerations.
A manual review and audit of ethical implementation is essential to ensuring ethical guidelines are followed. Just as handling sensitive data requires numerous legal and privacy reviews, it’s vital for AI models to assess fairness and implement any needed corrective measures.
Minimizing Potential Harm From AI
AI models are still new in many industries and elements of society, which again puts the onus on humans to ensure proper deployment. Enhancing awareness of ethical guidelines is paramount in the collection of information used to develop AI models. It’s critical for organizations to implement measures to obtain user consent for data collection and further usage while also prioritizing privacy throughout the life cycle.
The next step is to identify specific professionals involved in the audit. Data scientists and engineers can collaborate with domain-specific experts to thoroughly represent knowledge and implement AI. For example, AI implementation in healthcare requires technical expertise within model creation and professionals to fine-tune medication recommendations. Project managers can help identify goals and allocate people toward their accomplishment, while legal counsel can provide relevant guidance to ensure compliance.
Real-World Examples and Future Considerations
The chasm between leading innovators in AI implementation and those areas lagging is considerable, but no area or industry is immune to the impacts of unintentional biases. Image generation tools showed preferences or biases regarding occupation, with specialized roles displaying a disproportionate amount of older men while depicting younger women for most examples. Biases can also be subtle—for example, certain professions are displayed exclusively working in large buildings overlooking urban landscapes or skyscrapers, creating the perception that such jobs are only available in highly populated areas.
Companies, including Google and IBM, have created tools for bias mitigation and removal.
Just as humans work with AI to create these tools, it is imperative for users to develop trust in AI models for proper utilization. This starts with capturing consent anytime users feed data into these models to foster universal transparency and understanding of how the information is used, where the data goes, and other applicable considerations. The challenge is keeping consent statements concise so users will read and comprehend them rather than simply skipping to check off the box.
AI wields significant influence over society’s present and future, but ensuring that it happens in a responsible, ethical manner is a top priority. By properly implementing transparency and emphasis on continual learning, organizations can foster trust and dependability in AI.
Explaining the proper deployment of these models starts with user consent, which makes people aware of what data is used for which purposes. As AI advances, so will the need for human input and supervision of ethical considerations, especially with increased implications for sensitive topics such as education, health care, and climate change on the horizon.