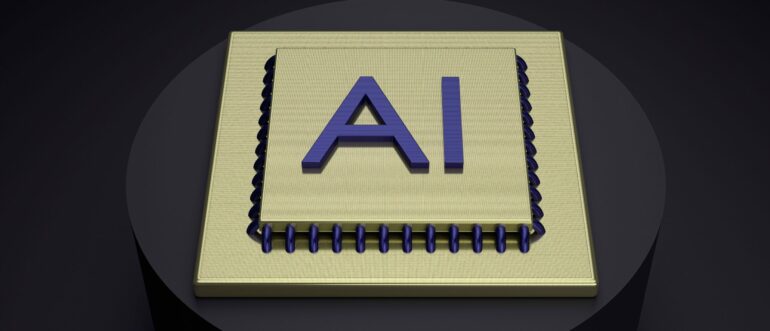
The launch of ChatGPT in 2022 marked a significant turning point in the AI landscape. Last year’s developments, from generative AI to multi-model AIs, have laid the foundation for substantial advancements in the AI space over the forthcoming years.
As we look ahead, it’s crystal clear that AI’s influence will only continue to grow. This year’s trends reflect a significant integration of AI across cloud and edge development and deployment strategies, and that’s what I will share with you today. Let’s get started!
Top 3 AI Trends in 2024
This year is exciting, challenging and demanding for AI businesses worldwide. Below are key trends that will shape the future of the cloud in the coming days.
Multi-Model AI
The next wave of AI promises richer customer experiences and intuitive interactions with the advent of Multi-Model AI. These models go beyond single-mode data processing to accommodate multiple input types, such as text, voice and images, a significant step forward toward human like thinking to process diverse sensory information.
Multimodal AI offers significant benefits, including cross-modal retrieval for better information discovery, multimodal sentiment analysis for deeper customer insights, integrated learning systems for improved accuracy in various sectors, and enhanced human-computer interactions. This leap in AI capabilities can potentially transform business operations and customer interactions, driving significant adoption of multi-model AI across industries.
Implications for Businesses:
- Multi-model AI integrating diverse data sources for comprehensive insights, allowing businesses to make more informed and strategic decisions.
- Combining multiple models to reduce errors and increase reliability, leading to more precise outcomes and predictions.
- Easily adapting to various tasks and industries with flexible model integration, ensuring businesses can grow and evolve without overhauling their AI systems.
AI Integration Across Edge and Cloud
One of the most exciting developments today is the convergence of AI with edge and cloud computing. In the coming years, AI workloads will move between the edge and the cloud. The cloud will be used for training complex AI models, while the edge infrastructure will handle real-time inferencing for fast responses.
Implications for Businesses:
- AI combining the cloud’s computational power for training with the edge’s low latency for real-time tasks, resulting in optimized performance.
- Automatically scaling workload and deploying models across new edge devices without significant infrastructure changes.
- Processing sensitive data locally at the edge, minimizing transmission risks and meeting stringent compliance requirements.
- Staying ahead by leveraging the latest edge and cloud technologies, fostering innovation and quick deployment of AI solutions.
AIOps
AIOps means using big data, analytics, machine learning and AI to improve IT operations. At its foundation, AIOps involves ingesting rich data from multiple sources, like metrics, events, and logs. Data is then processed with the help of AI and ML algorithms to detect patterns and predict potential issues before they occur. By automating routine tasks and incident responses, AIOps significantly improves operational efficiency, gaining significant momentum over the next few years.
Implications for Organizations:
- Automating routine tasks and processes, freeing up IT staff to focus on more strategic initiatives.
- Using advanced analytics to predict and prevent potential IT issues, ensuring smoother operations.
- Optimizing resource allocation and reducing operational costs through intelligent analysis and automation.
- Continuously monitoring and tuning IT systems for peak performance, minimizing downtime and disruptions.
A Transformative Era
The trends outlined in the article point to an exciting and transformative era in AI focused on enhancing efficiency and experience for both businesses and end-users. It will be interesting to see what’s next.