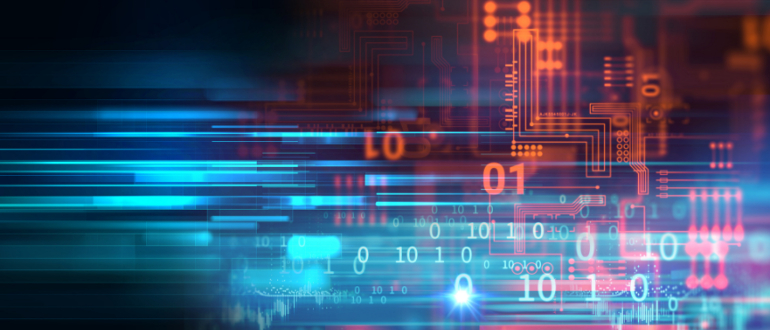
Generative AI (GenAI) has matured significantly over the past several years, supported by a growing workforce that is gaining a better understanding of the tools. This amounts to a maturation of the basic platform.
This platform extends beyond well-known large language models (LLMs) like GPT-4, Claude, Cohere, Gemini, and Llama that we know of. The models, besides excelling at understanding language and images and extrapolating insights, are now complemented by small language models (SLMs) that enhance the platform’s capabilities.
With the adoption of techniques like Retrieval-Augmented Generation (RAG) which leverages data stored as vectors that can be submitted to the LLM for more precise responses, the platform has evolved and improved in reliability to deal with hallucinations or incorrect responses. RAG and knowledge graphs have contributed to this by providing additional context and clarity through representation of real-world relationships and guiding answers within relevant frameworks.
Today GenAI powers many capable chatbots that are known to deliver cost savings and enhanced customer satisfaction. But is that the full extent of its potential?
Enterprises and investors are putting an enormous amount of capital behind expanding the capabilities of the base LLMs, and training new SLMs on specific industry domains. But the ringing question from investors and the C-suite executives is: When will we see transformative efficiencies delivered at scale?
Here are the predictions for how GenAI platforms will evolve in 2025.
Retaining Extensive Data Sets Will Become Fundamental
GenAI is fueled by a diversity of information: structured, unstructured, inter- and intra-enterprise data. Its potential depends on the data ecosystem that supports training, fine-tuning, and RAG processes. For models to be industry- or company-specific, organizations must retain massive amounts of data over long time periods.
But in a fast-changing world, determining relevant data becomes clear only in hindsight. For instance, consider a scenario where your company is adapting to a new geopolitical environment and if you had kept data from three years ago that would have made it much simpler. This highlights an important point: Retaining and managing extensive datasets isn’t an option—it’s fundamental. By integrating historical data with real-time insights, organizations can transform AI from an experimental tool into a driver of value at enterprise scale.
Over the next several years, we’ll see how the platform evolves to meet these challenges and deliver on its promise.
Filling Visibility Gaps Will Drive the Next Wave of GenAI Data Platform Deployment
While the technology for a GenAI data ecosystem exists, its deployment remains spotty. Real-time visibility into transactional, IoT, and customer behavior data is often siloed.
As enterprises ramp up from proof of concepts to trials and full AI deployment, their existing systems will be taxed. While initial trials may have been easy, unlocking GenAI’s internet-scale advantages demands data platforms that can support massive parallel access while simultaneously providing high-volume ingestion capabilities.
In 2025, enterprises will focus on filling these gaps by enhancing their data platforms to support vector data, similarity searches, knowledge graphs, and raw data stores for many types of data.
Achieving this will require balancing the ability to control data with accessibility while understanding its deployment across various uses and models. This shift marks a departure from simple chatbot usage toward truly incorporating GenAI into core enterprise systems to gain new insights and controls.
Enterprises Will Augment GenAI with Real-Time Data
The real value of GenAI materializes when enterprises begin deploying the first sets of applications across core systems at scale. In the past several years, companies have cautiously experimented with these tools. 2025 will mark a turning point, as the struggle to launch and scale GenAI across systems like customer support, manufacturing, supply chain, and finance, continues.
This will require tools to manage the deployment, and tracking data and GenAI models so that visibility into how and where data is used can be ensured.
One lesson learned over the last several years is that providing broad, generic answers is flawed. To make GenAI effective, it must be augmented with specific real-time data in the right forms of vectors and graphs. In 2025, we will start to see major vendors like Oracle, SAP, and Workday, as well as smaller ones, release enterprise applications that leverage these advancements. And only organizations that successfully navigate the challenges of data management and platform integration will be positioned to lead in AI.