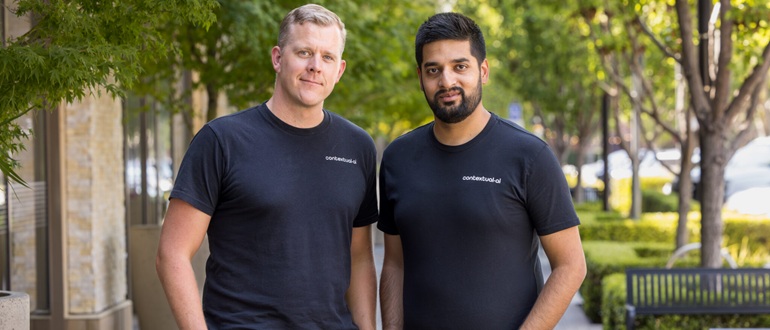
RAG is tightening. A distillation and narrowing of dataset analysis for custom-aligned artificial intelligence functions designed to serve organizations’ business models in specific context, retrieval augmented generation (RAG) is now being channelled into more specialized RAG agents to support expert knowledge work.
An effort is being carried out at Contextual AI to provide even more raggy RAG (not an industry term yet, but watch this space) with its eponymously named Contextual AI Platform. The platform now features what the company calls production-grade accuracy to support specialized knowledge tasks and boost expert productivity.
The Contextual AI Platform includes capabilities needed to build, evaluate and deploy specialized RAG agents, which is intended to be a route to taking higher-value AI initiatives from pilot to production in the face of vast amounts of noisy (aka unstructured, multi-form factor, non-deduplicated or normalized) enterprise data.
What Are Specialized RAG Agents?
While there is broad consensus that general-purpose AI agents are poised to streamline many generic tasks, Contextual AI believes that specialized RAG agents will now be required to transform a certain breed of high-value business tasks.
We could also argue that all RAG is inherently “specialized” by definition (i.e. it is obviously an extension mechanism for Large Language Models to be able to draw upon company-specific datasets in one form or another), the term in this context refers to specialized RAG agents that have been engineered for high-value domain-specific knowledge work. In other words, RAG is specialized – but essentially still general purpose when compared to specialized RAG agents that operate in advanced RAG workflows.
For subject-matter experts in any organization, this means having AI tools that match their level of expertise and can be trusted to address complex or technical problems with confidence.
Contextual AI now has released a set of public benchmark results in which its model CLM (Contextual Language Model) is benchmarked against Anthropic’s Claude 3.5 Sonnet and OpenAI’s GPT-4o across diverse enterprise domains.
“Enterprise AI has reached a critical turning point,” said Douwe Kiela, CEO of Contextual AI. “AI agents will soon be available to every employee at every company. However, the specialized work of subject-matter experts remains largely underserved. Specialized RAG agents built on the Contextual AI Platform bridge this gap, enabling [users] to boost their productivity with AI that truly understands their domain.”
Qualcomm (QCOM), a global semiconductor leader, chose Contextual AI after finding other RAG solutions inadequate for its highly technical customer engineering needs.
Conversational Context
Data engineering teams can now use the Contextual AI Platform to create specialized RAG agents that orchestrate both retrieval and generation based on conversational context. This mechanism is built to deliver accurate responses for complex knowledge tasks across large corpora of structured and unstructured enterprise data.
The platform is powered by Contextual AI’s own RAG 2.0 specification (an internally branded technology, not an industry-standard iteration point), which works by “jointly optimizing” the retriever and the generator in the RAG system to deliver accuracy and groundedness at scale.
Contextual AI says that previous generations of RAG have existed by stitching together frozen models, vector databases and poor-quality embeddings. The new RAG 2.0 works with a set of CLMs designed to outperform strong RAG baselines based on GPT-4 and the best open-source models by a large margin (the suggestion is based on the company’s research and its customers).
The platform provides streamlined deployment and tuning, reduced maintenance overhead, and a simplified AI tech stack i.e with development, evaluation, tuning and deployment in a single system optimized for RAG.
Benchmarking the RAG Pipeline
As a part of the platform’s GA launch, Contextual AI has released a set of public benchmarks comparing its platform to RAG architectures built using other AI companies. These benchmarks follow other research publications from Contextual AI, including LMUnit, a new paradigm for AI evaluation.
The company claims that the Contextual AI Platform was found to outperform other solutions across every major component (e.g. document understanding, reranking, groundedness) as well as in terms of end-to-end accuracy what is now solidifying and becoming known as the RAG pipeline.