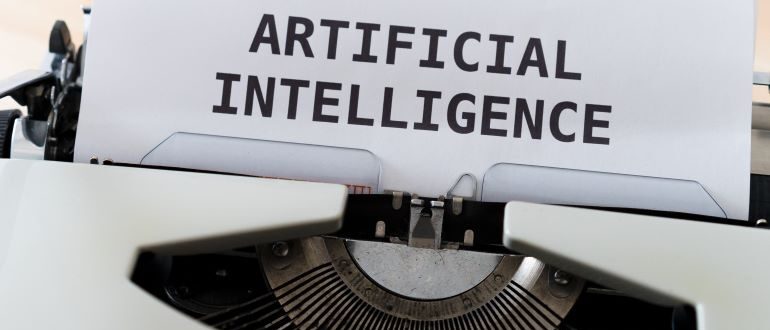
Competitive pressure to adopt and implement artificial intelligence (AI) technology is higher than ever before, with AI on everybody’s mind and modern platforms making it easier than ever to build and use AI models.
An Altair survey revealed high rates of adoption and implementation of organizational AI strategies globally. However, the survey also revealed that project successes suffer due to three main types of friction: Organizational, technological and financial.
“When it comes to technical friction there is a set of very diverse points the respondents make, explains Ingo Mierswa, senior vice president of product development at Altair. “One huge technical friction point is the lack of powerful compute infrastructures.”
He adds that while access to GPUs is currently limited, the good news is that for many successful AI models you actually do not need those.
“But this brings me to another identified friction point which is as much technical as organizational: The lack of skills and talent,” he says. “Personally, I think this is often a result of not enough upskilling and using the wrong tools for the job.”
From his perspective, organizations need to invest in their people to allow them to solve analytical tasks efficiently–but organizations also need to provide the right tools.
“Not every data science project requires coding; in fact only very few truly do,” Mierswa says. “If you are not using the right tools for the job, any work will feel harder than necessary.”
The lack of technical resources, skilled talent, and the use of inefficient tools are also core reasons for financial friction points.
About 25% of respondents confirm that financial friction holds them back, but those who overcome the other problems also report significant ROIs making it worth the efforts and investments for sure.
Jonathan Bruce, vice president of product management for Alation, noted 28% of respondents said leadership is too focused on the strategies’ upfront costs to understand how investing in AI and machine learning would benefit their organization.
“This is highly dependent on the vertical and the degree of data culture in that vertical,” he explains. “Banking, insurance, financial services, and the investment arena, each of whom has long sought to be more data-driven, are well ahead of this curve.”
He adds developing AI/ML models remains the purview of a high-code capable workforce.
“Until the arrival of generative AI, such as ChatGPT, the accessibility of AI/ML in a low-code form was largely inaccessible,” Bruce says. “Now, non-technical users can understand and extract value from AI/ML models, simply with clicks and no-code.”
In an enterprise capacity, however, these models are untrustworthy, as the underlying data processing governance and controls are nonexistent.
“There is too high a risk that generative AI models will produce a hallucination,” he cautions.
From Bruce’s perspective, the fluency and flexibility – and allure – of GenAI stems from its access to society’s collective intelligence.
“But the breadth of the public internet also introduces the risk of hallucination and inaccuracy,” he adds. “Constrain the training dataset and the bot will be less risky, but also less capable and less fluent.”
He says the beauty and allure of the technology is its impressive ability to sound smart, which is dangerous in a business situation.
“You could be unknowingly introducing inaccuracies into decision-making,” he notes. “To be effective, generative models should be fine-tuned on domain-specific data catalogs and their output should be reviewed by humans. They can accelerate human work but not yet safely perform it solo.”
Bruce explains CDOs, CTOs, or CIOs all play a role in implementing an AI adoption strategy, noting stakeholders include all functions.
“We are likely to see movement first in the CMO function, which is far more data-driven and ideally positioned as a function of the multi-channel customer-driven journeys that emerged over the past 20 years,” he says.
Randy Leblanc, vice president of data analytics with Altair, says in many organizations, the same teams who were responsible for data analytics initiatives focused on enterprise data, data visualization, data exploration, and data automation are now focused on bringing in AI.
“Sometimes these initiatives are IT lead, either because business stakeholders have already identified the need and tasked IT with fielding a solution, or because IT knows and is providing a capability in front of demand,” he says.
In several other organizations those tasked with AI adoption are people with innovation titles, or heads of digital transformation for key business processes (Head of Manufacturing 4.0, Head of Digital Transformation) who are driving the initiatives inside key business lines, where other business lines will follow.
Bruce adds that while it’s a cliché to say that software has captivated the world, AI is likely to do the same for software.
Exercised correctly – fed with trusted, governed data and overseen by data analysts – it can provide considerable benefits to all aspects of the lifecycle of innovation.
“AI can deliver a degree of customer loyalty and productivity that we’ve never seen before, from the conception and delivery of innovation to providing a deeply personal experience,” Bruce says.