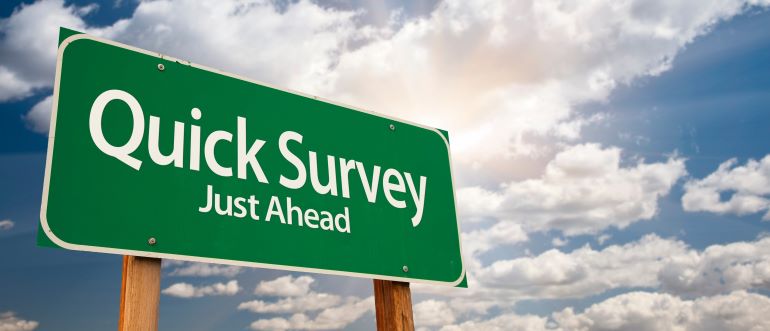
A survey of 550 IT and business professionals across the U.S., United Kingdom, Ireland, France and Germany from organizations with 500 or more employees suggests organizations are putting too much faith in the output of artificial intelligence (AI) models despite knowing how incomplete or flawed the data used to train them might be.
Conducted by the market research firm Vanson Bourne on behalf of Fivetran, a provider of tools for moving data, the survey finds 81% of organizations trust AI/ML outputs. Just under a quarter (24%) said that they have reached an advanced stage of AI adoption, where they rely on AI with little to no human intervention.
However, more than two-thirds of organizations are struggling to both access all the data needed to run AI applications (69%) and convert the data into a usable format (68%).
The report finds underperforming AI models result in organizations losing on average 6% of their global annual revenues, or $406 million, based on average global annual revenue of $5.6 billion.
Not surprisingly, nearly every respondent (97%) said their organizations are investing in generative AI in the next one to two years. However, 42% said their organization has experienced data hallucinations. Maintaining data governance and financial risk due to the sensitivity of data are cited as being the top generative AI concerns at 37% each.
Rather than immediately trying to operationalize AI across their entire business, it is clear businesses need a more prescriptive approach that starts with improving data quality, says Fivetran CTO Mark Van de Wiel. “It needs to be more deliberate,” he says.
Less clear is the pace organizations will be building and deploying AI models, but as application development continues to evolve, the bulk of most applications will include one or more AI models. The amount of data required to train all those AI models is nothing short of massive. The simple truth is most organizations today don’t have the robust processes in place required to manage data at that level of scale.
Data management barriers to AI adoption cited by survey respondents include disorganized or siloed data (43%), lack of support from senior leaders (42%), current IT infrastructure is outdated (42%), lack of internal skills (41%), access to data (40% and low-quality data (35%). Overall, nearly every respondent (96%) said they are encountering at least one barrier to adoption.
In general, data science teams hired to construct AI models wind up spending a significant amount of their time on basic data management tasks. More than two-thirds of respondents (67%) said data scientists spend most of their time preparing data, rather than building AI models.
More than two-thirds of respondents (67%) did note their organization plans to deploy new technology to strengthen basic data movement, governance and security functions. That may be critical, because even before the current AI boom there was a critical shortage of data engineering expertise.
At this rate, it may be a while before organizations truly operationalize AI. In the meantime, the amount of attention being made to data management issues that have often been long ignored is only going to increase.