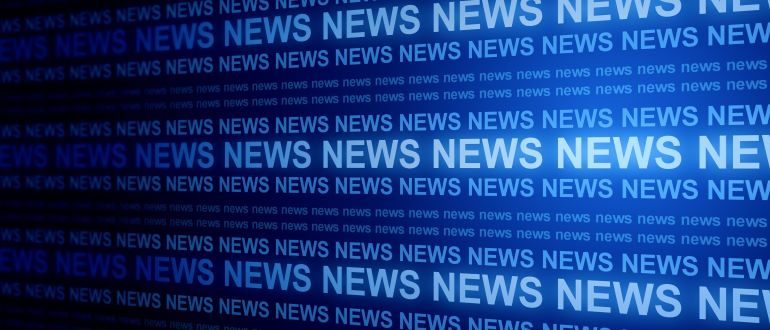
Vapor IO and Supermicro today launched a service designed to make it simpler to deploy applications infused with artificial intelligence (AI) models at the network edge.
The managed Zero Gap AI service will provide access to servers based on the NVIDIA MGX platform with the NVIDIA GH200 Grace Hopper Superchip running in more than 36 cities that can be accessed via either wired or 5G wireless networks. Initially, the service is being made available in Atlanta and Chicago using network services provided by Comcast.
Vapor IO already provides an edge computing service based on micro datacenters at the base of cell towers coupled with nearby wireless aggregation hubs. That service is now being expanded to include servers from Super Micro configured with NVIDIA GPUs to run the inferences engines that are at the core of most AI applications.
The challenge organizations face is GPUs, in addition to being scarce, are expensive. “It can take 52 weeks for GPUs to be delivered,” says Vapor IO CEO Cole Crawford. The Zero Gap AI service alternatively provides platforms connected via a mesh network at the edge for running AI applications that Vapor IO manages on behalf of organizations, adds Crawford.
That approach makes it possible to deploy inference engines to drive, for example, computer vision application closer to the point where data is being processed and consumed so that organizations can invoke either at a flat rate or based on consumption of infrastructure resources, he notes.
There’s little doubt that AI engines will be deployed everywhere from the network edge to the cloud. The challenge is to find a way to achieve that goal given the cost of GPUs and the need to be able to manage highly distributed computing environments. Vapor IO in collaboration with Super Micro is, in effect, making a case for a service that leverages IT teams from Vapor IO to centrally manage AI infrastructure deployed at the network edge.
Each organization will need to decide for themselves to what degree to rely on a managed service versus deploying and managing their own infrastructure, but a service should enable them to devote more resources to building and deploying software at a time when AI models are making it more challenging to build distributed applications.
In the meantime, it’s becoming apparent that a GPU shortage is holding back development of those applications. Many organizations are still determining how best to operationalize AI, but in the absence of GPUs it’s challenging to both train AI models and optimally run certain classes of inference engines.
Ultimately, there will be no shortage of managed services for deploying AI applications. The issue that IT teams will need to navigate is how many points-of-presence those services have in locations that will enable AI applications to be deployed in a way that minimizes at much as possible application latency.
One way or another, however, as AI models become ubiquitous at the edge, the laws of physics that govern networking will inevitably become a more pressing concern.