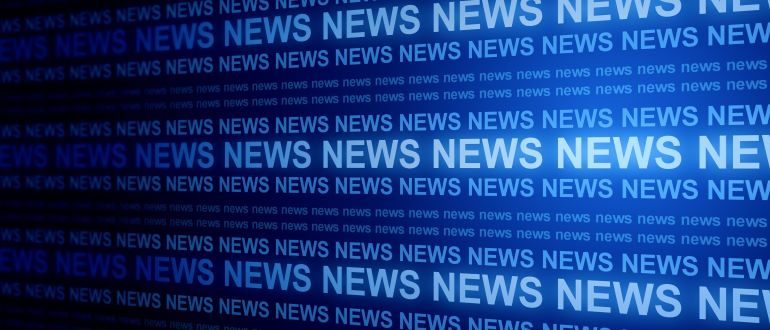
Securiti today extended its ability to govern and secure data by adding an offering that makes it simpler to build data pipelines for generative artificial intelligence (AI) applications.
Dubbed Gencore AI, the platform provides IT teams with a graphical interface and templates through which they can dynamically create and modify the data pipelines that are used to train AI models.
The overall goal is to accelerate the rate at which pipelines made up of both structured and unstructured data pulled from hundreds of sources can be created in a way that retains any original metadata that might be attached.
That capability makes it simpler to build complex data pipelines without necessarily requiring the expertise of a data engineer, notes Securiti CEO Rehan Jalil. “It reduces dependencies on specialists,” he says.
The core Securiti platform is based on graph technologies the company originally developed to secure data by making it simpler to apply controls at scale. Gencore AI leverages that capability to both automatically learn which controls, such as entitlements, to enforce and monitor the provenance of how data is being accessed across multiple large language models (LLMs) and vector databases.
Additionally, a natural language conversation-aware LLM Firewall capability protects user prompts, responses and data retrievals to both prevent data leaks and thwart prompt injection attacks and jailbreaking instructions.
As organizations look to operationalize AI, many are encountering a raft of data management challenges ranging from a lack of expertise to governance mandates that require them to be able to explain what data was used to create a specific AI model. Those issues are only going to become more challenging as organizations continue to train and replace AI models as more data sources become available, says Jalil.
Ultimately, organizations will need to find a more holistic approach to training and replacing AI models that existing IT teams can easily master, he adds.
It’s not clear how many organizations are struggling with data management issues at the dawn of the AI era. In theory, it would be better if they could simply deploy AI models where their data resides. In practice, the data needed to train AI models is strewn across the enterprise. Constructing the data pipelines needed to move data into some type of central repository that can be used to then train AI models is, in most organizations, going to be unavoidable. Right now, however, the tools required to create, manage and govern those pipelines are not often well integrated. More challenging still, much of the data needed is managed by disparate teams that often don’t have much of a history working with one another.
Data management has, of course, always been to one degree or another problematic, and many organizations only move data as a last resort. However, organizations are now finding that data is a lot more valuable when it is aggregated in a way that AI models can consume. The challenge is the demand for data engineering expertise required to aggregate massive amounts of data using, for example, best DataOps practices, at least for now, far exceeds the available supply.