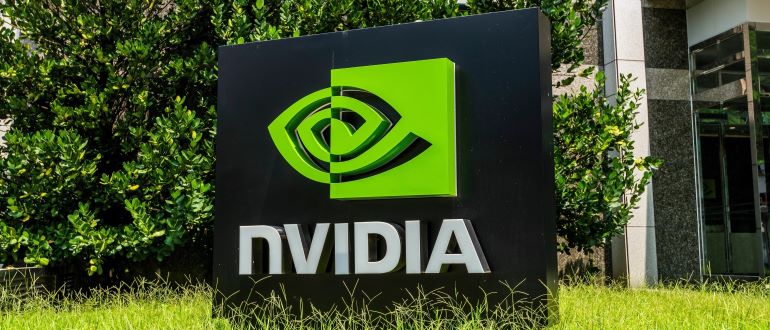
NVIDIA this week unfurled a bevy of extensions to its portfolio, including an instance of the NVIDIA DGX Cloud platform, that are all being made available on the Amazon Web Services (AWS) cloud service.
Announced at the AWS re:Invent 2024 conference, the two industry titans also revealed that the NVIDIA GB200 NVL72 server platform is now integrated with AWS network switches and storage systems.
NVIDIA and AWS are also optimizing data science and data analytics workloads with the RAPIDS Accelerator for Apache Spark, which accelerates analytics and machine learning workloads in a way that leads to 80% reduced processing costs. Quick Start notebooks for RAPIDS Accelerator for Apache Spark are now available on Amazon EMR, Amazon EC2 and Amazon EMR on EKS services.
In addition, the NVIDIA IGX Orin and Jetson Orin platforms are now integrated with AWS IoT Greengrass to streamline the deployment of AI models at the network edge.
NVIDIA is also adding an ability to run NVIDIA Isaac Sim reference framework on Amazon EC2 G6e, instances accelerated, based on NVIDIA L40S GPUs to enable developers to simulate and test AI-driven robots that will be deployed in a physical environment using a virtual environment.
Additionally, the NVIDIA BioNeMo NIM microservices and AI Blueprints, developed to advance drug discovery, are now integrated into AWS HealthOmics, a fully managed biological data compute and storage service.
Finally, NVIDIA CUDA-Q is now integrated with Amazon Braket processors to streamline quantum computing development. CUDA-Q users can use Amazon Braket’s quantum processors.
The latest extension of the NVIDIA alliance comes at a time when AWS is simultaneously making a case of using its Trainium processors as an alternative to graphical processor units (GPUs) to train and deploy AI applications.
However, platforms such as NVIDIA DGX Cloud are designed to run on multiple platforms, says Alexis Bjorlin, vice president of DGX Cloud at NVIDIA. That’s critical because many organizations want to be able to more easily train an AI model in the cloud, but deploy the inference engines created in an on-premises IT environment where their data already resides, she adds.
Organizations today are especially sensitive to being locked into specific platforms in the wake of recent changes made to VMware licensing terms, notes Bjorlin.
There will always be alternatives to GPUs to run AI applications given the current insatiable demand for GPUs, but organizations need to also consider the impact other classes of processors will have on AI accuracy, says Bjorlin.
In addition, the number of partnerships and alliances made by NVIDIA provides access to a richer set of technologies, notes Bjorlin. “The ecosystem matters,” she says.
As is the case with most providers of IT platforms, the relationship between AWS and NVIDIA is complicated. In addition to providing access to Trainium, AWS also makes available services based on NVIDIA GPUs, including next year the forthcoming Blackwell-class of GPUs that NVIDIA is expected to deliver next year.
In addition, the two companies provided AI Blueprints for deploying applications on GPUs and are working on Project Ceiba, an effort to build an AI supercomputer using GPUs hosted in the AWS cloud.
Each organization will need to decide to what degree to rely on one AI platform versus another, but the one thing that is certain is it’s likely to include a wide range of classes of processors that, in addition to serving different use cases better than others, might also be more readily available.