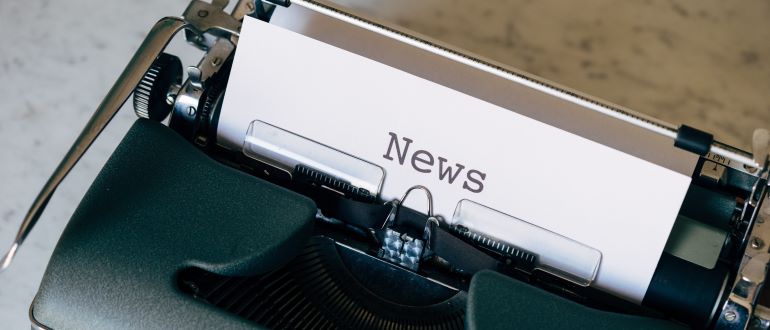
Anthropic this week revealed that its next generation large language models (LLMs) will be trained using processors from Amazon Web Services (AWS).
Announced at the AWS re:Invent 2024 conference, Project Rainier will make use of Trainium2 processors rather than graphical processor units (GPUs) to create a foundational model that could exceed a trillion parameters.
Anthropic’s chief compute officer, Tom Brown, told conference attendees Project Rainier will require access to hundreds of thousands of processors to build the next generation of the Claude series of LLMs. Previously, Anthropic has committed to making AWS its primary infrastructure partner following a $4 billion investment AWS made in the company.
Launched last year, Trainium2 processors are based on custom silicon that AWS specifically developed to optimally run deep learning algorithms. AWS makes those processors available via EC2 UltraClusters service that provides access to up to 100,000 processors.
That’s critical because the more access an LLM has to processors the more feasible it becomes to use large datasets to create more accurate models. “We’re building trustworthy AI that scales,” says Brown.
It’s not clear to what degree Tranium2 processors might reduce the current level of dependency on GPUs to train LLMs, but in time the next generation of Claude will be used to train a wide range of additional smaller models. AWS, of course, is hedging its bets by making available multiple classes of processors, including GPUs, via the Amazon Bedrock service, but the company has invested billions in developing custom silicon that is designed to run various classes of workloads more efficiently than general purpose processors.
Additionally, AWS has also invested in networking technologies such as a 10p10u switch and fiber optic cables along with AWS storage systems that are specifically optimized for AI workloads.
Peter Desantis, senior vice president for AWS Utility Computing, told conference attendees those investments make it possible to efficiently run AI workloads that tend to scale up much more than other classes of workloads that generally scale out across a wider range of distributed systems.
It’s not clear how long it might take to train the next generation of the Claude LLM but the AI arms race between Anthropic and its rivals is set to continue into 2025. Anthropic is seeking to unseat OpenAI as the the current dominant provider of foundational models that can be customized to drive a wide range of use cases involving AI agents that make use of the reasoning capabilities embedded in an LLM to automate tasks.
Of course, there is little doubt that other providers of cloud services are making similar investments in AI infrastructure services. The challenge, as always, is finding a way to train massive LLMs in the most efficient way possible. Up until recently, that generally meant relying on GPUs that make it possible to process data in parallel. However, those GPUs were not designed from the ground up to process AI workloads, which is now creating an opportunity for other approaches to more cost effectively train models using other classes of AI accelerators.