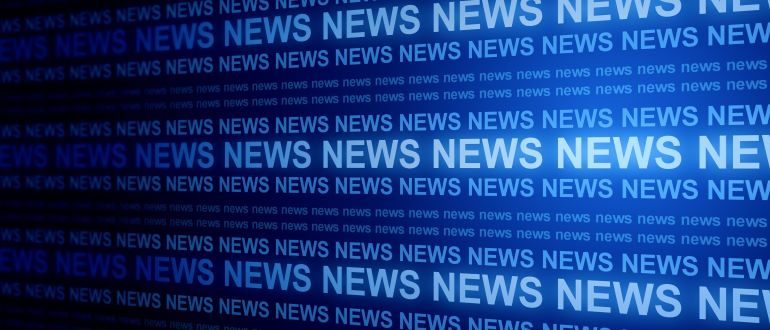
NetApp today extended its portfolio of infrastructure platforms for building and deploying artificial intelligence (AI) models by adding support for additional graphical processor units (GPUs) from NVIDIA along with software that optimizes the running of inference engines on NVIDIA GPUs.
A FlexPod for AI reference architecture has been extended to add support for NVIDIA AI Enterprise software platform running on distributions of Kubernetes from either Red Hat or SUSE. In addition, NetApp platforms have been validated to integrate with NVIDIA OVX, a platform for deploying inference engines using GPUs and data processing units (DPUs) developed by NVIDIA.
NetApp also announced that NetApp AIPod powered by NVIDIA DGX is now a certified NVIDIA solution. That platform is primarily used to train AI models in on-premises IT environments that are being used as an alternative to cloud computing environments that, because of compliance regulations, an organization might not be allowed to use.
While most organizations rely on GPUs to train AI models, there are multiple options for deploying inference engines. NVIDIA has been making a case for deploying GPUs to run inference engines, but as most organizations building AI models have already encountered – those GPUs are in short supply. As a result, many organizations continue to rely on processors from Intel and AMD to run inference engines, or processors based on a design created by Arm.
Regardless of approach, the cost of building and deploying AI models at scale remains considerable. That issue is forcing many organizations to limit the number of AI projects they might otherwise launch. In fact, one of the reasons to partner with a vendor such as NetApp is they typically have more access to GPUs supplied by their partners, noted Phil Brotherton, vice president of solutions for NetApp.
In addition, more organizations are also concerned about the level of sustainability that can be achieved and maintained when building AI and deploying AI models that increase carbon emissions.
It’s not clear to what degree the acquiring of IT infrastructure to train models is connected to the acquisition of the systems used to run the inference engines those models create. Data science teams typically exercise a lot of influence over what platforms or services to use to train an AI model, but the decision concerning what platforms should be used to deploy an AI model is most often made by IT operations teams. In theory, organizations that need to train AI models in an on-premises IT environment may be able to benefit from discounts if the acquisition of those systems is bundled with the platforms used to run the inference engine, but the number of organizations that might financially benefit from that approach is still comparatively limited.
Ultimately, there will one day be new classes of processors optimized specifically to run AI workloads at scale. In the meantime, IT teams will need to carefully navigate a range of options that are increasingly being modified to run AI workloads alongside a wide range of applications that today have very different attributes.