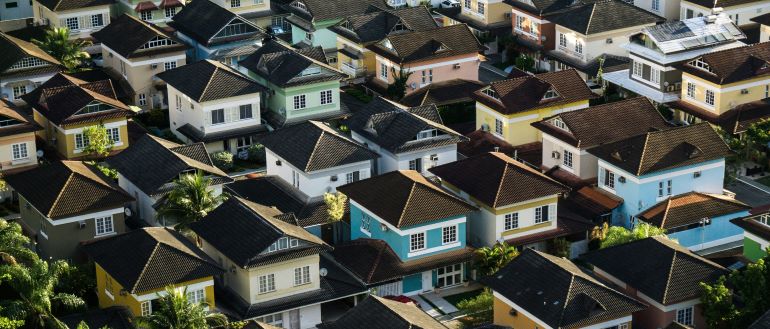
Real estate market crashes are infrequent on a national scale, yet they cause devastating local and regional effects when they occur. Such downturns manifest through property value decreases driven by speculation, economic uncertainty and policy changes.
The Great Depression from 1929 to 1939, the savings and loan crisis in the 1980s, and the 2008 housing bubble demonstrate that these events follow cyclic patterns and cause extensive destruction. The emergence of artificial intelligence (AI) and machine learning (ML) as predictive tools enables better identification of property market collapses through enhanced data analysis, offsetting the likelihood that such crashes will recur.Â
Understanding Real Estate Market Crashes
Real estate bubbles are periods when prices increase rapidly and unsustainably. It starts when investors buy properties aggressively because they predict continuous price growth.
The housing market experiences such behavior because of declining interest rates, which increase borrowing options and encourage builders to develop more properties in anticipation of sustained demand (Figure 1). Changes to regulatory policies through tax law modifications and monetary policy adjustments can disrupt this market balance.
Figure 1: Impact of interest rates and inflation on real estate prices 2010-2014. Image courtesy of Sarfarazurrehman Shaikh.
Specific historical patterns are detectable before market downturns. Rapid home price increases, rising speculative transactions and excessive construction activity create a high-risk environment.Â
The primary weakness of traditional forecasting approaches is their inability to detect these patterns during real-time operations. Using historical averages and linear assumptions in legacy models fails to detect dynamic market cycles and non-linear economic relationships that signal potential market collapses.Â
The Role of Macroeconomic Indicators in Market Instability
The integration of macroeconomic indicators creates accurate forecasting capabilities. Home price values and housing demand are directly affected by economic indicators, which include gross domestic product (GDP) growth, inflation, unemployment rates and interest rates (Figure 2).Â
Figure 2: Correlation between macroeconomic indicators and real estate prices. Image courtesy of Sarfarazurrehman Shaikh.
Slower economic growth reduces consumer confidence and purchasing power, leading to decreased home sales and property values. Foreign direct investment can stabilize markets through added liquidity or distort market conditions depending on the circumstances. The real value of these indicators emerges when ML systems analyze their combined data to detect hidden patterns between elements.
Advantages of AI and ML in Market Forecasting
The primary advantage of AI and ML models is how they handle large datasets and detect non-linear relationships while delivering fast and practical insights. Unlike traditional systems, these models quickly adapt to new data and market behavior.
AI demonstrates its most valuable benefit by creating economic simulation models. Through random forests and long short-term memory (LSTM) networks, analysts can evaluate past events, such as the 2008 housing crash, assess their investment strategies and forecast market shocks, an approach explored in economic modeling studies. Real-time analytics and anomaly detection extend these systems’ usefulness by providing early warnings that prove essential in unstable and overheated markets.
The ML Models Best Suited for Market Prediction
Various ML models have proven helpful for real estate market forecasting. The following are especially effective:Â
- Random forests:Â This model exhibits exceptional performance in detecting extreme events, combining decision trees to boost predictive accuracy. The system reveals which factors, including interest rate increases or declining GDP, have the most significant impact on warning of a market collapse.
- Support vector machines (SVM) and gradient boosting machines (GBM):Â These models are effective for classification and risk analysis.Â
- Recurrent neural networks (RNNs) with LSTM:Â The models achieve superior time-series forecasting by detecting long-term dependencies and patterns in housing data.
- Natural language processing (NLP) models:Â Sentiment analysis through NLP enables the identification of public and investor confidence shifts that usually signal market corrections.
- Hybrid models:Â These models unite economic principles with deep learning methods to enhance predictive strength and explainability using domain knowledge with data-based inference.
Applications and Strategic Benefits of AI-Driven Forecasting
The practical applications of these models extend across the real estate ecosystem. Predictive analytics helps investors identify growth areas and evaluate risks to adapt their strategies, as seen in deep learning studies analyzing California real estate trends. AI models offer transparency into whether growth stems from fundamentals or speculation. AI systems also assist financial institutions by detecting anomalies and evaluating systemic risks and benefit policymakers and regulatory agencies by delivering real-time economic intelligence at the local level.Â
From Data to Decision: Training the Models
AI models require diverse, reliable data sources to function properly. The typical dataset comprises property transaction records, mortgage interest rates, construction data, household debt statistics and economic metrics such as GDP and inflation.Â
Because ML models rely on massive datasets, training incorporates information from the past two decades, including the 2008 financial crisis, to help identify patterns that preceded major market downturns, as demonstrated in research exploring macroeconomic determinants of housing downturns. After deployment, the system continues to learn from new available data while improving its predictive accuracy.
AI systems use ensemble learning methods and time-series analysis techniques to minimize data uncertainties and volatility. AI achieves stability through output validation across various systems, reducing the likelihood of incorrect positive results. Normal market corrections differ from structural crashes, which AI models can effectively identify.
Challenges and the Road Ahead
Real estate forecasting with AI tools encounters multiple hurdles in its implementation process. The quality and accessibility of data present significant challenges because markets that lack standardized and transparent reporting face particular difficulties. Complex models limit usability, and integration requires financial investment and skilled personnel.
The complexity of data privacy regulations and ethical standards creates additional barriers for organizations. The accuracy of predictions depends on unbiased training data because any form of bias leads to incorrect predictions.
High initial costs and the industry’s natural resistance to change have resulted in delays in adopting new technology. Companies like Zillow and Redfin use AI to detect overvaluation and estimate property values to offset such implementation obstacles.
Despite these challenges, the future holds promise. AI’s predictive capabilities will likely grow through the integration of the internet of things (IoT) and blockchain technologies. ML models that receive data from IoT devices and sensors tracking building occupancy, energy consumption, foot traffic patterns and other factors, along with blockchain-based smart contracts, enable real-time assessments of asset performance and local demand. When combined with traditional economic indicators, these data sources significantly enhance forecast accuracy, delivering more detailed and timely predictions.