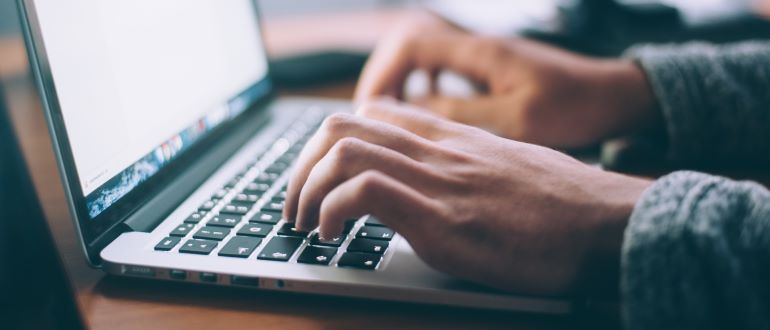
Integrating artificial intelligence (AI) and machine learning (ML) into financial forecasting represents a seismic shift for forward-thinking organizations seeking to navigate a complex marketplace by making faster, more effective, data-driven decisions with greater confidence.
By harnessing the power of advanced algorithms, companies can analyze vast datasets, forecast future financial scenarios and optimize resource allocation in real time. Organizations that adopt AI-driven forecasting tools effectively position themselves to anticipate change and drive strategic initiatives that foster long-term growth and profitability. Organizations worldwide, including Square, Canoe and Northwestern Mutual, leverage ML-based financial forecasting for their extensive benefits.
Key Advantages of AI-Driven Forecasting
AI and ML technologies redefine traditional financial planning through four main capabilities: enhanced forecasting accuracy, continuous and dynamic forecasting, market trend detection and prediction, and bottom-line metric enhancement. Each advantage adds tremendous value to a business’s financial operations.
Enhanced forecasting accuracy. AI and ML excel at interpreting time-series data to identify patterns and make more accurate predictions than manual methods. By mitigating human errors and accounting for internal and external variables, ML-powered models reduce forecasting inaccuracies by 20-50 percent, according to a report from IBM. This capability is extremely critical in high-stakes environments where precision is essential to informed decision-making.
Continuous and dynamic forecasting. Unlike manual quarterly projections, AI-enabled systems significantly improve the frequency of forecasting by analyzing data inputs in real time. This agility facilitates proactive adjustments to market trends. Over time, ML models can be retrained to identify hidden correlations and enable predictive analytics at unprecedented speed and scale.
Market trend detection and prediction. AI and ML enhance an organization’s ability to detect and proactively capitalize on marketplace trends. By incorporating seasonal patterns, economic indicators, consumer behavior, and global trends, these systems can project potential outcomes of varying scenarios, including supply chain disruptions and regulatory changes. This helps decision-makers refine their strategies proactively.
Bottom-line metrics. AI and ML improve bottom-line metrics by optimizing resource allocation and reducing operational costs. For instance, ML models can analyze historical expense data to highlight areas for cost containment while predicting revenue streams based on detailed financial drivers. AI-powered forecasting increases the accuracy of financial predictions, which minimizes risks and informs decision-making, such as scaling investments in high-return areas or mitigating losses in underperforming sectors. AI and ML tools help decision-makers make better-informed choices, leading to improved bottom-line metrics, like return on investment (ROI), net profit margin, and working capital.
Best Practices for Implementing AI and ML in Financial Planning
To maximize the success of AI/ML integration, companies can follow these best practices:
- Research and model selection. Choosing the appropriate algorithm or model is foundational to the success of any AI/ML initiative. Skilled ML data scientists and engineers offer the expertise necessary to customize or integrate turnkey solutions tailored to specific requirements and organizational objectives.
- Data integrity and security. The success of any AI initiative hinges on high-quality data. As such, it is essential to eliminate inconsistencies or biases that may impact accurate functionality. Periodic audits can identify potential biases, especially in historical datasets that may reflect outdated trends. Data privacy and security are also top concerns. Robust data governance practices, including encryption, secure storage, and regulatory compliance, are non-negotiable.
- Integration with existing systems. Although ML models can operate as standalone tools, they often deliver enhanced value when integrated into existing enterprise resource planning (ERP) or financial planning and analysis (FP&A) platforms. Third-party tools offer a flexible alternative for companies not ready to invest in internal talent. Plug-and-play platforms facilitate rapid deployment and immediate insights.
- Evaluation and analysis. Regular testing and validation are critical to ensuring model reliability. Techniques such as back-testing, cross-validation, and the use of metrics such as mean absolute percentage error (MAPE), the primary metric for measuring prediction accuracy by indicating the average percentage error in forecasts, can assess performance. It’s important to monitor prediction accuracy over time, comparing AI-generated forecasts to real-world results and then fine-tuning models as needed.
- Ongoing monitoring and adaptation. Because AI systems constantly evolve, continuous monitoring and reevaluation are essential to ensuring alignment with business goals. It’s crucial to allocate adequate resources for ongoing maintenance, including updating training datasets, to facilitate accurate, strategic decision-making.
The Role of Collaboration and Leadership
Implementing AI in financial forecasting and planning requires transparent responsibility allocation and strategic leadership. While FP&A teams typically drive forecasting and planning efforts, integrating AI requires collaboration with the IT department, which offers the data engineering support necessary to ensure datasets are accessible, clean and secure.
Leadership support is equally important for successful AI integration. A top-down approach, where executives align AI initiatives with overarching business goals, fosters companywide buy-in. Management allocates necessary resources, supports training, and fosters a culture that encourages innovation. In any AI/ML implementation plan, executives and decision-makers do well to remember that these technologies are best used to augment rather than replace humans, and people will continue performing reviews on produced data and model maintenance.
AI and ML: Strategic Imperatives for Success
In a global and digital business environment, adopting AI-powered financial forecasting tools is no longer optional—it’s a strategic imperative. By leveraging new and emerging technologies, companies enhance operational efficiency, decrease costs, mitigate risks and increase profitability.
Success, however, requires more than the adoption of AI and ML tools. It demands a strategic vision, expert execution and an unwavering commitment to transparent and ethical AI practices. Businesses that embrace this paradigm shift future-proof their enterprises in an era defined by data-driven insights and technological innovation.