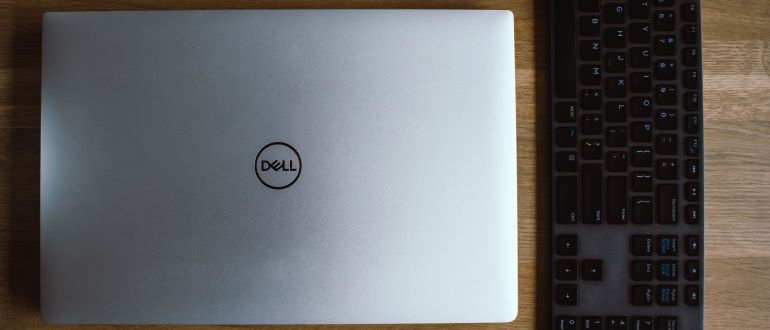
A frenzy among providers of IT infrastructure providers is growing in intensity as enterprise IT organizations look to build and deploy artificial intelligence (AI) models.
Dell this week, for example, touted its plans to add support for graphical processor units (GPUs) from AMD to train large language models (LLMs) for Dell PowerEdge XE9680 servers. The goal is to provide IT teams with a less costly alternative to GPUs from NVIDIA.
At the same time, Dell is also continuing to support NVIDIA, most recently in the form of an instance of Dell storage arrays that have been validated to run with the NVIDIA DGX SuperPOD AI infrastructure. Dell is also rolling our all-Flash instance of its Dell PowerScale storage system that is designed to scale out in a way that optimizes throughput when using GPUs to train AI models.
Dell, in effect, is making a case of an infrastructure stack that is specifically optimized to run multiple types of LLMs that can be extended using, for example, vector database capabilities provided by software partners such as Databricks, says Martin Glynn, senior director of product management for Dell Unstructured Storage. “We’re taking an AI first approach,” he says.
It’s not clear to what degree AI models will be trained in on-premises IT environments but given concerns about data privacy and the rise of data sovereignty regulations more organizations than ever are looking at this option as an alternative to moving data into a cloud service. Many of the LLMs that an enterprise IT organization is likely to either customize or build will be domain specific. As such, they are likely to be measured in terabytes rather than the multiple petabytes of data required to train a foundational LLM that was built by OpenAI or Amazon Web Services (AWS).
In addition, enterprise IT organizations lack clarity into how usage of LLMs will be priced. Most providers of these services are providing organizations with tokens that can be used for a specific cost. The issue is that a token is required for each input and output of an AI model. In effect, every interaction will cost two tokens. Over time, the cost of using LLMs running on a cloud service might prove cost prohibitive.
Of course, not every organization is as AI ready as they might like. Much of the data needed to train or customize an AI model is strewn across the enterprise. Much of that data is either conflicting or simply wrong. Arguably, the most important thing any organization can do to get ready for AI is to get their data management house in order.
The challenge is many business leaders now see all things related to AI as a strategic imperative. In fact, conversations about the need to bring some discipline to data management before embracing AI can be downright awkward. Too many business leaders assume that IT teams have been optimally managing data when it fact most of them have been focusing on processing and storing it as cost effectively as possible. The quality of the data is the responsibility of the business units that created it in the first place. Unfortunately, not nearly enough business units have, for longer than many care to admit, been taking enough responsibility for the quality of that data as they should.