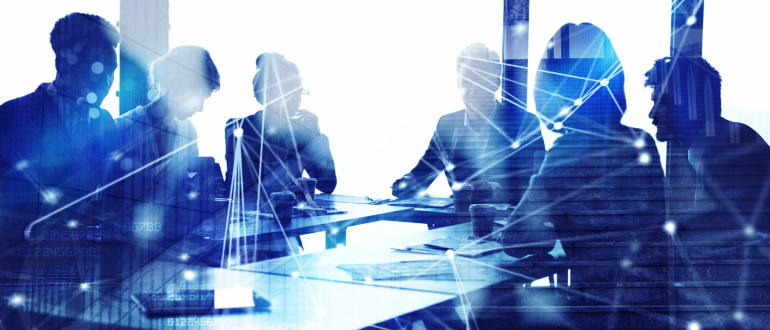
In IT Service Management (ITSM), the precision of artificial intelligence- (AI) driven insights is fundamentally anchored in the underlying data quality.
High-quality data ensures that AI models function optimally, leading to reliable decision-making processes. Conversely, poor data quality can result in misleading analyses and suboptimal outcomes.
Data quality encompasses accuracy, completeness, consistency, timeliness, validity and uniqueness. These dimensions are essential for AI models to produce trustworthy and actionable insights. In the context of ITSM — where decisions often have significant operational implications — ensuring data quality is paramount.Â
For instance, inaccurate or incomplete data can lead to erroneous predictions about system performance, potentially causing unnecessary downtime or misallocation of resources. Moreover, high-quality data strengthens user trust in AI systems, promotes transparency and supports compliance with industry regulations such as the General Data Protection Regulation (GDPR) and the Health Insurance Portability and Accountability Act, which emphasize data integrity and security.
Strategies for Implementing Robust Data Quality Frameworks
Building a strong data quality framework requires a series of deliberate actions designed to preserve data integrity at every stage of its life cycle. The following strategies are foundational:
- Define data quality dimensions: Clearly articulate what constitutes high-quality data within the organization by specifying dimensions such as accuracy, completeness and consistency.
- Establish data quality rules and guidelines: Develop and document rules that govern data entry, processing and maintenance to ensure uniform standards across all datasets.
- Implement data validation techniques: Utilize automated validation tools to detect and correct errors at the point of data entry, ensuring that data conforms to predefined formats and logical parameters.
- Adopt data cleansing protocols: Regularly perform data cleansing activities to identify and rectify inaccuracies, remove duplicates and fill in missing values, maintaining dataset reliability.
- Deploy continuous data monitoring systems: Implement real-time monitoring tools to continuously assess and report data quality metrics, allowing ITSM teams to detect anomalies and take corrective action promptly.
- Integrate data stewardship programs: Assign data stewards responsible for maintaining data quality standards across departments and facilitating collaboration between IT and business units.
Addressing Common Challenges in Maintaining Data Quality at Scale
Data quality management at scale is fraught with challenges, particularly when dealing with multiple data sources, real-time data streams and the integration of legacy systems with modern technologies. One significant challenge is ensuring data consistency across different sources and formats.Â
Data integration presents challenges such as ensuring consistency across various sources, reconciling legacy systems with modern structures, and maintaining data quality and accuracy throughout the process. These hurdles can introduce inconsistencies and errors, which — if not addressed — can negatively impact AI-driven decision-making and the insights derived from such data. To tackle these challenges, businesses should consider implementing the following strategies:
- Standardize multiple data sources: Organizations often integrate data from various systems, increasing the risk of inconsistencies. To mitigate this, teams should standardize data formats and use master data management platforms to create a single source of truth.
- Utilize real-time monitoring systems: This approach helps businesses stay on top of data quality, promptly identifying and correcting issues, which supports better demand management and reduces the likelihood of errors influencing decisions.
- Implement data validation techniques early in the data pipeline: Ensuring data consistency and accuracy from the start can help prevent errors from propagating downstream, ensuring reliable AI-driven insights.
- Establish robust governance frameworks: A strong data governance framework is essential for overseeing data integration, ensuring data quality is maintained throughout the process.
Prioritizing Data Quality for Reliable AI Insights
Data quality management is not a one-time initiative but a continuous commitment that underpins the success of AI-driven insights in ITSM.
By implementing robust frameworks that include validation, cleansing, monitoring and governance strategies, organizations can ensure their data remains accurate, consistent and trustworthy throughout its life cycle. Addressing challenges such as multiple data sources and real-time data streams requires proactive, scalable solutions aligned with ITSM best practices.Â
Ultimately, prioritizing data quality enables AI systems to drive more informed, reliable and impactful decisions, solidifying an organization’s competitive advantage in an increasingly data-driven world.