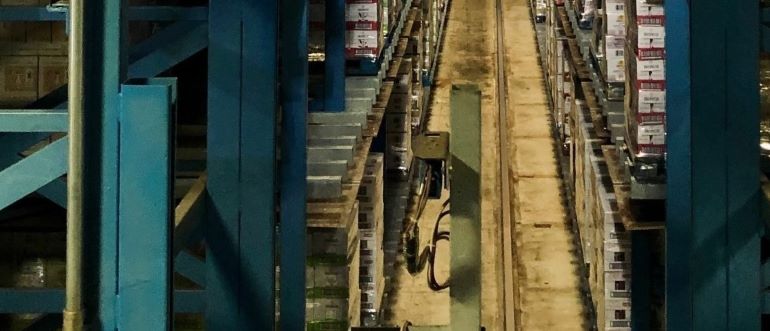
Early adopters of artificial intelligence (AI) are already seeing positive impact on revenue and profits as manufacturers look to automation to reduce costs, according to Aptean’s Discrete and Process Manufacturing 2024 Trends and Outlook for North America report.
The study surveyed North American manufacturers, encompassing sectors like electrical equipment, fabricated metals, machinery, cosmetics, chemicals and pharmaceuticals, highlighting key industry challenges and their influence on emerging trends.
Of note, more than half of surveyed manufacturers identified rising costs and supply chain disruptions as top concerns. The report emphasizes the critical role of digital transformation and automation in addressing these challenges, showing that manufacturers who embraced automation experienced an average growth rate 30% higher than those reliant on manual processes in 2022.
Supply chain digitization also emerged as a game-changer, with businesses achieving over 1.6% greater revenue growth in 2022 compared to their non-digitized counterparts, a significant leap from the 0.7% growth difference in 2021.
The research, conducted in partnership with B2B International in August 2023, gathered insights from 200 decision-makers in the North American manufacturing sector, highlighting the increasing importance of AI and automation in the industry.
Infosys executive vice president and global head of manufacturing Jasmeet Singh explains if applied effectively, AI can enable manufacturing leaders to drive cost reduction through four levers.
He explains the first lever is about labor cost reduction through mechanisms, such as enhanced automation and scheduling optimization, while the second is material cost reduction by controlling scrap and rework.
“The third is the cost of capital, which can be lowered by increasing equipment uptime while reducing the inventory carrying cost,” he says. “And, finally, there’s energy cost which can be reduced by optimizing energy usage.”
Each of these are enabled by AI’s capability to predict and pre-empt events, and together, these levers enhance operational efficiency by positively impacting KPIs such as overall equipment efficiency, throughput and labor productivity.
“The foundational capabilities enabled by AI such as predictive maintenance, computer vision and robotic process automation are equally suited for both discrete and process manufacturing,” Singh adds. “The difference lies in the quantum of value which a certain use case can deliver.”
For example, discrete manufacturing often involves significant amount of material handling as material flows from work center to work center or across production lines.
“There’s a relatively bigger play for AI enabled autonomous mobile robots in discrete manufacturing,” he notes.
On the other hand, predictive maintenance has a bigger impact in process manufacturing because the machinery tends to be much more complex, and the cost of a breakdown is highly disruptive and costly.
“There’s an opportunity for discrete and process manufacturers to learn from each other as the problem complexity can be vastly different even if the base use case is the same,” he says. “If they can learn how a harder problem encountered in the other industry has been solved, it can be easier for them to then apply the learning in addressing a relatively easier problem in their industry.”
Gartner analyst Pedro Pacheco points out the “billion dollar question” is how leadership at manufacturing firms develop and implement AI integration roadmaps into their business operations strategies.
“It’s important to focus on the biggest bang for the buck, meaning a focus on areas where AI adoption will bring you a big financial benefit,” he says. “That also builds a strong case for adoption overall–if you present a major financial benefit, it’s harder to ignore the call for investment.”
Pacheco lists automation, energy savings, process and quality control and predictive maintenance as some core areas where AI integration could hold major financial and operational benefits for manufacturing companies.
“Use of AI could also improve some manufacturing processes where there is what’s called a ‘ramp up period’, when you have a stamping machine in an automotive factory for example,” he says.
Once that machine is set up and starts producing, organizations are unable to reach full capacity straightaway due to a ramp up period in which the amounts of parts it can produce per unit of time is progressively increased.
“In some cases, by using AI you can reduce that ramp up time to achieve a high cadence in a shorter time period, which once again means cost reduction,” he explains.