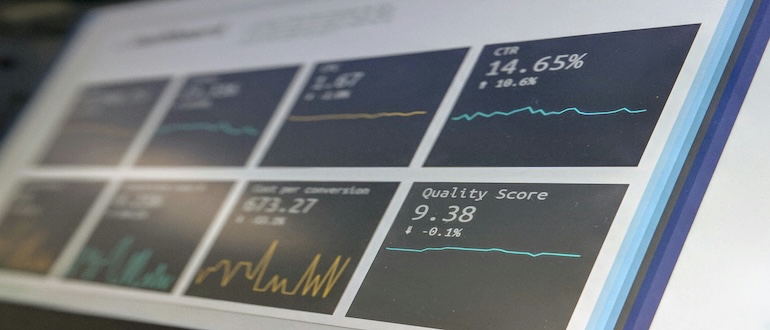
Generative artificial intelligence (AI) has emerged as one of most exciting and transformational pieces of AI – a field that has made progress in leaps and bounds in the past few years.
Powered by deep learning models, GenAI uses massive volumes of training data to create realistic text, graphics and audio content. The models can analyze reams of data in a heartbeat and make predictions and recommendations on any subject.
Here’s how it works: Leveraging deep learning, the models examine patterns in extensive datasets and replicate them to produce new data that resembles human-generated content. Typically the process begins with data collection and data cleaning where data is culled from various sources and prepped for model training. This data is then used to train and optimize the model. Through inferencing, the model generates new content, and the cycle of learning is repeated for continuous improvement.
Thanks to its growing smarts, today GenAI has penetrated almost every field, from content creation to life sciences, marketing to media, accounting and whatnot. But beyond data analysis, GenAI also has the capability to design and develop creative workflows from scratch. Within an organization, this can be leveraged to improve customer experience, speed up digital transformation and automate and streamline manual processes.
Key use cases currently include summarization, email generation, customer support, coding, 3D modeling and fraud detection.
In the recent years, it has also carved out a niche in cloud-native development. Cloud-native development deals with creating applications that are scalable, resilient and fault-tolerant that can run on the cloud. Generative AI makes the development process faster and easier by automating tasks like code testing and cybersecurity analysis while optimizing cloud-native observability, resource management and response processes.
As the technology evolves, we expect to see more automation within the cloud environments. However, there are some challenges and risks to using generative AI in cloud-native development. First and foremost, there are heavy security and privacy implications associated with intellectual property rights. The technology also poses a myriad of cybersecurity threats via phishing emails, deepfake videos and so on.
Environmental concerns because of the high computational need of the models is another big stumbling block. But an even bigger challenge is the models’ tendency to produce biased outputs that have led to spread of misinformation and reputation damage.
In Conclusion
There is no doubt that generative AI has incredible potential to create opportunities across industries. Recent development forecasts a future where AI will be an omnipresent in our day-to-day lives. With constant learning and self-improvement, the models will become more human-like in expertise and experience, and that will lead to enhanced creative output, greater automation, more personalized experiences and accelerated innovation. But the challenge lies in keeping the technology ethical and protecting our society while leveraging its transformational benefits. If we can keep the technology useful for the people instead of aiming to replace them with it, generative AI can make significantly impacts across industries and applications alike.