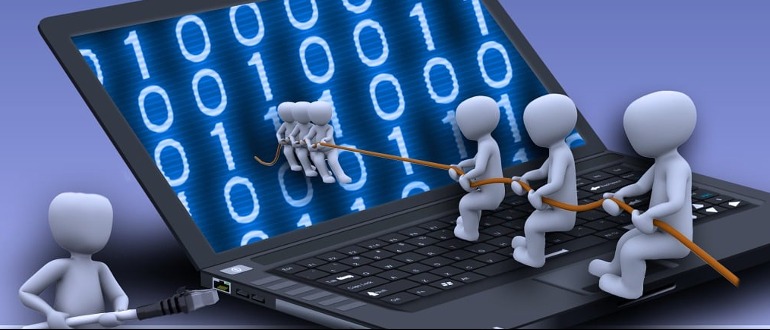
Zilliz today updated a self-hosted edition of its open source Milvus vector database that makes it possible for organizations to rely on a managed service to deploy it.
Previously, an internal IT team would have been required to deploy and manage an edition of the Zilliz Cloud Bring Your Own Cloud (BYOC) offering for each platform they deployed it on.
That approach enables organizations to securely deploy the Milvus vector database in IT environments where they control how data is managed without having to also hire an IT professional that has vector database management expertise, says Zilliz CEO Charles Xie.
Many organizations are reluctant to rely on a managed cloud service because of regulatory requirements that prevent them from storing sensitive data outside of an IT environment they don’t directly control. “The data doesn’t leave their environment,” says Xie.
In effect, Zilliz is now providing organizations with all the benefits of a managed service without having to be concerned about who outside their organization might be able to view their data, he adds.
That’s especially critical for organizations that, for compliance purposes, would otherwise have to vet every person who can access their data, Xie notes.
Zilliz Cloud BYOC additionally provides support for fine-grained permission settings, private link support between the control plane and data plane, and communication via outbound port 443 to ensure that data is only transferred via the HTTPS protocol.
The Milvus vector database is based on Sparse-BM25 algorithms for converting text into embeddings that can be consumed by artificial intelligence (AI) models. Zilliz claims version 2.5 of the Milvus platform can process one million vectors in six milliseconds in a way that also eliminates the need to deploy and manage a separate keyword search platform.
That capability makes it feasible to use Milvus in a wider range of real-time AI applications running on a range of embedded systems, including mobile phones and autonomous vehicles. It also streamlines the number of calls to application programming interfaces (APIs) that otherwise would need to be made.
Regardless of how organizations decide to expose additional data to a large language model (LLM), making certain the right data shows up in the right place at the right time requires a significant amount of data engineering expertise. Arguably, the less time data engineers have to spend on configuring databases, the more time they will have to focus on ensuring the right output is generated by AI models that are only as accurate as the data that they have been exposed to by the organizations invoking them.
In the meantime, as more organizations look to operationalize AI models, the amount of appreciation for data engineering will only increase. The challenge, right now, is finding enough of that expertise to enable data science teams to deploy an AI model in an actual production environment versus simply becoming another experiment that ultimately became yet another wasted research effort that C-level executives by the day are clearly becoming less interested in funding.