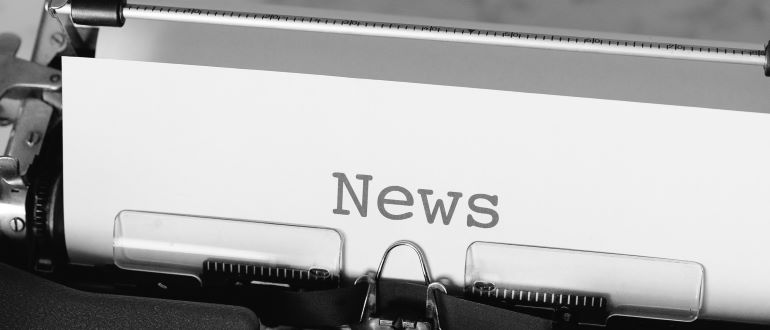
Voxel51 today made available version 1.0 of an open source tool that makes it simpler to normalize and validate visual data that is being used to train artificial intelligence (AI) models.
FiftyOne provides data science teams with a tool that make uses of algorithms to streamline the collection of, for example, 3D and LiDAR data, says Voxel51 CEO Brian Moore. “It can be used with any type of visual data collected from various types of sensors,” he says.
That capability improves the productivity of data science teams by reducing the overall amount of manual effort that would otherwise be required, adds Moore. Rather than rely on a proprietary tool to achieve that goal, an open source approach also enables data science teams to collect and normalize that data in a more transparent manner that helps make it simpler to explain how an AI model was constructed, notes Moore. That’s especially critical whenever an AI application needs to comply with regulatory mandates, he adds.
FiftyOne is based on a FiftyOne Brain platform that Voxel 51 built to apply machine learning algorithms to collecting and normalizing visual data. The company also makes available a commercial FiftyOne Teams edition of the tool that is designed to foster greater collaboration between data engineers. In fact, Voxel51 claims the teams edition of the platform increases productivity by up to 50% while improving model accuracy by as much as 30%. Existing customers include LG Electronics, Berkshire Grey and Precision Planting.
As organizations look to deploy AI models based on visual data across a wider array of use cases, they are discovering that collecting, organizing and normalizing that volume of data requires a massive amount of time and effort. FiftyOne provides those teams with a tool that makes it simpler to create an AI model that, for example, would be used to build a computer vision application.
Voxel51 has also created an extensible framework that makes it easier to use Python to create custom dashboards in addition to building interactive data applications or trigger actions across a set of workflows. Additionally, Voxel51 provides native vector search integration with the Elasticsearch platform to make it simpler to customize AI models.
It’s not clear just how many computer vision applications organizations are looking to build and deploy, but the number of potential use cases involving everything from robotics to self-driving vehicles is enormous. Most of those use cases will involve some type of AI application running on an embedded system running at the network edge. Ultimately, there could be many more of these types of AI applications than there are those running in a local data center or on a public cloud.
The challenge, of course, goes beyond training the AI models needed and building the applications that invoke them. AI models deployed at the edge will regularly need to be updated using the latest data available. As such, organizations will need to eventually marry machine learning operations (MLOps) and DevOps workflows in a way that unifies the efforts of data science and application development teams that today typically have vastly different cultures.