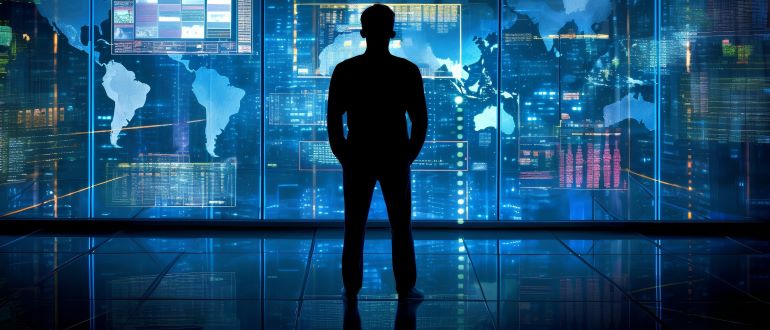
Generative AI has disrupted every industry in the world and turned them on their heads – GPT probably being the most recognizable model. Open AI’s GPT is a large language model (LLM), and these types of models are designed to accurately understand intent and respond with human- like conversations and output. These LLMs are extremely advanced, and we are just beginning to scratch the surface with their applications and their enhancement to business growth, especially in customer experience.
While LLMs are an incredible advancement in generative AI, there has been a disconnect between the revolutionary technology and its implementation with only 19% of businesses utilizing it. On the other hand, nine out of ten IT leaders consider generative AI to soon have a major role in their company.
Taking a closer look, there are some reasons for the skepticism and differing opinions, but there is also an often overlooked solution: Specialized-LLMs.
LLMs: As They Stand
In a simple explanation, LLMs are a type of AI that are trained on large amounts of data, covering patterns of words and phrases in order to generate new content. It should be noted that more specific requests will result in more accurate responses. These models have come a long way from when they were first introduced, and they are constantly being updated to mirror human-like conversation through training on language, syntax, and intention. However, LLMs that are trained on such massive amounts of general information and data sets often produce generalized results with hallucinations, leaving users dissatisfied and uninterested.
So how do companies leverage LLMs for a wide range of functions, including customer experience, marketing and sales, to reduce latency and hallucinations while increasing usage? By building models on domain- or use-case-specific information.
Specialized-LLMs
To maintain a competitive edge in harnessing generative technology as the leading frontier for productivity and efficiency, businesses must act swiftly. Many companies are already joining the generative AI trend, demonstrating the urgency. As per Gartner’s research, customer experience is the number one focus as businesses implement generative AI, and it will continue to be a top priority for years to come. As businesses are trying to manage consumer demands in real time and enhance user experience amidst skyrocketing expectations, they’re realizing the only way to keep up and remain competitive is to integrate the fastest, most effective solutions, which happens to be generative AI.
But, because no business is the same and it’s unrealistic to think that one generalized solution will work the same for every company, LLMs need to be trained on specific requirements that pertain directly to the business and its objectives.
Specialized enterprise LLMs are trained on industry-specific knowledge as well as domain or use- case-specific language and concepts. As hospitality, finance and human resources are all vastly different in their terminology as well as concepts, their solutions need to be just as diverse. The one-size-fits-all model fails to cater to the unique needs of each, resulting in hallucinations, latency, poor integrations and generic outputs.
By creating a specialized solution, companies can achieve 40% – 50% reduction in service interactions, increase customer satisfaction and respond with accurate and faster resolution times. With this hyper-productivity and augmented customer experience, companies could see massive growth and ROI (up to $80 billion by 2026).
How to Implement
Before integrating any sort of generative AI into a business, it’s important to have a plan. Here’s a few things to consider:
1. Start with Assessing Your Business’s Current Needs. Failure to consider the needs and uses of generative AI can end up costing companies huge amounts of resources and wasted time.
2. Clearly Define the Objectives of Deploying the Technology. Whatever the technology will be used for, there needs to be precise objectives implemented with ethical guidelines.
3. Decide Between In-House Automation or a Provider. While building an in-house solution can be an option, it can be very costly and would require a complex level of capabilities and expertise. Therefore, it is beneficial to partner with an automation vendor that is equipped to deliver on the benefits of specialized LLM solutions specific to your needs and use case.
4. Integrate the Technology in Phases. Continually monitor and maintain the new automation to ensure optimal performance. Creating a feedback loop will keep your system up to date and constantly improving.
5. Establish a Strong Foundation of Data Security. Be sure to meet compliance in accordance with industry standards and rules, identifying vulnerabilities and patching up your systems.
6. Scale Up. After some time of positive results, companies can begin to scale up their use of generative AI. It should be noted that scaling up too quickly can result in performance issues and loss of resources.
In 10 years (maybe three at this rate), this will be old news, and the idea of running a business without generative AI or AI in general will be like trying to work without a computer.
The moment is opportune, and enterprises must act promptly by incorporating specialized LLMs tailored to their unique requirements. This integration paves the way for a unique experience that translates into business expansion and limitless potential.