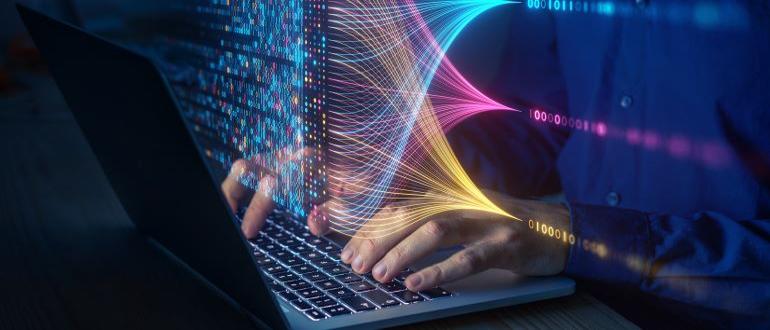
JFrog today unveiled a machine learning operations (MLOps) platform that accelerates the building and deployment of artificial intelligence (AI) applications via tight integrations with a DevOps platform.
Additionally, the JFrogML platform provides integrations with Hugging Face service for accessing open source artificial intelligence (AI) models, and tools and platform such as Amazon Web Services (AWS) Sagemaker, MLflow developed by Databricks, and the NVIDIA NIM microservices framework for building AI applications using containers.
Based on the MLOps platform that JFrog gained with the acquisition of QWAK.ai last year, the JFrogML platform is designed to make it simpler to incorporate AI models into existing DevOps workflows managed by the JFrog Artifactory platform that organizations rely on to build and deploy applications, says JFrog CTO Yoav Landman.
There is, of course, no shortage of MLOps platforms for building AI models but the level of integration between those platforms and the DevOps workflows that organizations use to manage software artifacts is comparatively limited, notes Landman. Integrations with the NVIDIA NIMs framework and the Hugging Face service also make it simpler to access multiple large language models (LLMs) as needed.
In addition to providing tools to manage data and observe AI models, the JFrogML platform streamlines the workflows used to build AI applications in a way that also serves to make it simpler to document which artifacts were used to construct them. “It provides organizations with a system of record for building these applications,” Landman says.
JFrog has also integrated its existing Xray software composition analysis (SCA) to enable organizations to apply best DevSecOp processes, including JFrog certified checkmarks, to those workflows, he adds.
Building and deploying AI applications require a level of collaboration between data scientists, data engineers, DevOps teams and cybersecurity professionals that can be difficult to achieve and maintain. Many of the existing AI models that organizations have built were created by small, dedicated teams that often have their own unique culture. Operationalizing AI at scale requires data science teams to become more integrated with the DevOps processes used to build and deploy modern applications. The JFrogML platform provides an integrated approach that enables organizations to more easily bring the diverse cultures that make up an IT organization together in a way that accelerates the deployment of AI applications, Landman says.
That’s critical because going forward, just about every application being developed is going to include one or more AI models. The challenge many organizations are already encountering is that models over time need to be replaced as new data sources are incorporated. They also tend to drift beyond the scope of their original design as they are exposed to additional data, which might also necessitate replacing them.
Finally, the pace of LLM innovation now occurs at a pace that many AI models previously built are quickly being made obsolete by LLMs that provide access to more advanced capabilities, such as the ability to reason across much larger sets of data.
It’s still relatively early days when it comes to integrating MLOps and DevOps workflows, but the one thing that is certain is that building, deploying, managing, securing and updating AI applications at scale is going to be all but impossible without it.