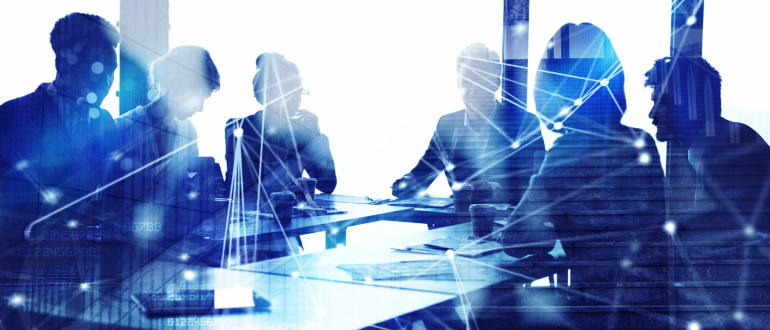
A few years ago, substantial enterprises or organizations within traditionally data-driven industries were the only organizations practicing anything close to MLOps. Few organizations even had the data scientists and machine learning (ML) engineers necessary for an MLOps organization on staff – let alone DevOps and IT teams having the tools or knowledge to work successfully with MLOps organizations.
The 2022 University of Cambridge paper, Challenges in Deploying Machine Learning: A Survey of Case Studies, notes that lacking necessary expertise is one of the top reasons AI/ML projects fail. That’s no surprise; the technology and processes are being newly applied to MLOps and AI, and MLOps is a new and rapidly evolving field.
What’s state of the art today may be superseded by something much better next week, month or year. And for MLOps to be successful, all of the relevant enterprise disciplines need to be brought up to speed, says Miroslav Klivansky, global practice leader of analytics and AI at Pure Storage. “In the enterprise, it is more likely that staff will come from a traditional operations or analytics background. Some baseline training is required to participate in new AI initiatives and take advantage of MLOps tools,” Klivansky says. “Then there is the question of domain expertise and how staff taking on new projects need to familiarize themselves with the areas their AI will support,” he says.
Staff training is critically important for successful enterprise MLOps adoption and implementation. Below are key explanations and methods for ensuring MLOps training success:
Upskill all Relevant Teams
MLOps is multi-disciplinary and spans data science, software development, operations and security. To work effectively, each of these teams must understand the needs and motivations of other teams, the MLOps fundamental principles, and the tools used. “Great people are curious and ambitious to learn, so providing opportunities for staff to ramp up on MLOps-specific training is a no-brainer,” says Lou Flynn, senior manager for AI at SAS. “This extends to stakeholders outside of data scientists and MLOps engineers. Cross-functional teams need to know how to work with one another and understand how to tackle the natural tension and seamlines in the MLOps space,” he adds.
Bridge Teams
Just as the operations and development worlds needed to converge with DevOps, the model development (data scientists) and operations must converge with MLOps. “Training initiatives can include cross-disciplinary learning to promote a deeper understanding of the business context and encourage a holistic approach to machine learning projects,” says Olga Kupriyanova, principal consultant with global technology research and advisory firm ISG. “Therefore, ongoing staff training is not just an investment in individual competency, but a strategic imperative that drives the overall success and sustainability of MLOps efforts within an organization,” Kupriyanova adds.
Enable Cultural and Process Changes
Adopting MLOps requires significant cultural and process changes; training programs can help govern this transformation. “Ongoing training is vital for ensuring the proper governance of all analytic deployments and trust around them. This encompasses everything from the actions people must take to the processes they must follow and the technology that supports them throughout their lifecycle. Additionally, it’s important to implement upskilling for people as their roles shift from managing to supervising AL and MLOps,” says Hillary Ashton, chief product officer at Teradata.
Reduce or Avoid Technical Debt
Without the proper training across teams, problems are bound to mount. These can include too many manual processes, lack of model monitoring and rogue models. Keeping teams cross-trained and individual skills sharp can go a long way toward improving MLOps practices and governance and avoiding creating problems that will have to be undone later.
Last year, ClearML released a survey highlighting how challenging it is for businesses to attain business value from their ML deployments, in which 86% said they struggled. It’s clear successful MLOps training will help go a long way toward improving proficiencies, collaboration and model production.