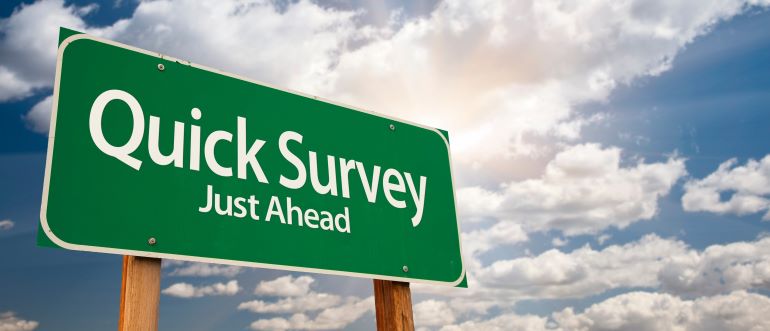
A survey of 1,000 senior technology executives with artificial intelligence (AI) expertise that are employed by mid to large enterprises finds that, on average, organizations have deployed 150 AI models in production environments.
Conducted by S&P Global Market Intelligence on behalf of Vultr, a provider of cloud hosting services, the survey also finds two-thirds (66%) are building or developing their own models or using open-source models. Almost half (47%) are relying on some type of third-party partner that is either a domain expert (25%), global systems integrator or technology provider (22%) or hyperscaler (15%).
More than two in five respondents (41% to 45%) said that AI made major contributions to market share, revenue, customer satisfaction, marketing improvements, and cost and risk reduction.
Overall, 87% of respondents said they expect to see a moderate or significant increase in AI spending, with 85% expecting to have achieved advanced usage of AI in the next two years.
While resources are limited, organizations today are not entirely certain which AI projects will provide the best return on investment (ROI), notes Eric Hanselman, chief analyst at S&P Global Market Intelligence.
As a result, many organizations are tending to lean toward funding a large number of proof-of-concepts (PoCs) to determine when and how to best apply AI, he adds. The challenge then becomes how to operationalize those PoCs, if and when, it is determined they add value, notes Hanselman. “They need to plan more for success after the PoC,” he says.
Challenges respondents are encountering, for example, include insufficient CPU or graphical processing unit (GPU) resources (65%), data locality issues (53%) and storage performance issues (50%).
Respondents are using a range of platforms to train AI models, with 38% conducting AI training in cloud and hybrid environments while 35% are using on-premises infrastructure. Security (35%) and open ecosystems (33%) were listed as concerns, followed closely by cost (29%).
A full 85% anticipate growing the amount of inference performed at the edge in the near future, the survey also finds.
Additionally, just under a third data governance (30%) and technical infrastructure constraints (29%) were shared as major challenges to reaching the next stage of AI maturity.
In general, it’s only a matter of time before AI becomes democratized to the point where IT operations teams are managing models, notes Hanselman. The immediate challenge is making sure the infrastructure deployed can run those AI models at an acceptable level of price/performance, he adds.
Ultimately, most organizations will, depending on the use case, be running a mix of generative, predictive and causal AI models. The challenge will be determining which types of AI models to employ in combination to ensure the best outcome. Generative AI models, for example, are not deterministic so, in effect, they are providing a best guess for an outcome based on the data exposed to a large language model (LLM). That approach, however, may not be applicable in use cases where the outcome needs to be much more deterministic, notes Hanselman.
Regardless of the type of AI model employed, the one thing that is certain is it will not be too long before AI models are pervasively deployed across the entire enterprise.