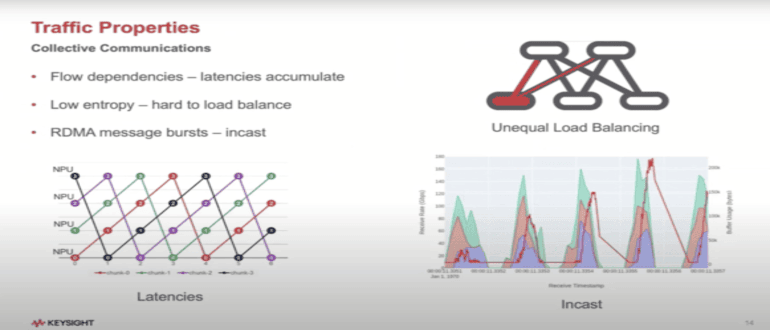
Keysight Technologies has officially joined the AI zeitgeist. In March, the electronics design and test solutions provider announced Keysight AI Data Center Test Platform to help enterprises benchmark and evaluate new AI network infrastructure fabrics in-house without using GPUs.
“AI is getting deployed everywhere for the last three years, since ChatGPT came out,” said Ankur Sheth, director of AI test research and development, while showcasing the new product at the AI Field Day event in Silicon Valley last week. “And people who have tons and tons of GPUs are deploying it. They need to test the infrastructure that is getting built at scale.”
This is the market Keysight hopes to tap into in a year when AI adoption reaches a record high among large companies. A lineup of top companies driven by Microsoft, Google and Meta have loaded up on computing power as they brace for the competition.
Keysight positions the new AI Data Center Test Platform as a solution tailored for large hyperscale operators, network equipment manufacturers, and ASIC and accelerator vendors that use a large concentration of GPUs and AI hardware.
Ideally, performance numbers of AI cluster fabrics are measured and tested on compute systems having GPUs and NICs. But these systems come with lofty price tags. Setting up a lab with dozens of such expensive and scarcely available appliances for testing is cost-prohibitive. Additionally, the benchmarking process requires deep skill and adds months to the production cycle. Few customers would be happy about the additional wait time.
“Compute capacity is not something you want to spare for testing,” said Alex Bortok, senior product manager, while demonstrating the platform.
When AI workloads are run on physical systems, the metrics you get characterize the performances of the elements. However, the information is not correlated, and you never know what caused a degradation, he said.
The Keysight AI Data Center Test Platform offers a workaround with which fabrics can be tested with realistic workloads, but without using actual GPUs.
“We emulate hardware, the network cards using our hardware traffic generator and test the network and provide deep networking insights,” said Bortok.
The platform benchmarks by emulating high-scale AI workloads and tracking the collective communication performance of GPUs in response to those.
The platform uses high-density traffic load appliances supporting over 256 100-800GE ports to simulate realistic traffic patterns. Prepackaged applications specialized in measuring and analyzing AI infrastructure run on the platform measuring things like the bandwidth available to AI collectives, and the rate of fabric utilization. They gather the data and metrics and analyze them using Keysight’s backend AI engines.
The platform, Bortok explained, taps into two AI engines, the AI Data Center Test Platform Software, and the widely deployed network test hardware – AresONE-M 800GE and AresONE-A 400GE.
The application called Collective Communications Benchmark provides comparative values of the observed bandwidth versus the theoretical maximum. The second application available to users, Workload Replay, measures GPUs response to synthetic workloads at different moments with different sizes of datasets to help operators benchmark the network accordingly.
Considerable Accuracy
The reports tell operators the estimated job completion time with considerable accuracy. The performance analytics and insights allow users to improve the performance of AI cluster fabrics while accelerating the pace of AI/ML innovation.
“Fifty percent of the time, GPUs are idle, waiting for data which is not optimal,” says Bortok. A medley of failures obstructs GPU performances in AI clusters. Among others, network problems account for 20% of the failures, leading to shockingly low GPU utilization.
“And as you scale, the component failures accelerate exponentially,” he added. “We need to shrink that time in order to accelerate the training.”
At the minimum, AI workloads require a high-bandwidth, high-throughput, low-latency scalable network that does not exhibit frequent inflections or congestions.
Quoting Andrej Karpathy, former OpenAI researcher, Bortok said, “If you want to run AI workloads on a multi-node distributed cluster, you better benchmark your network.”
“Co-tuning the software stack on the servers with the network is critical to get to the performance because the developers of that stack typically test it in an environment that is radically different from what any particular operator will have in production.”
Keysight was divested from HPE after 70 years, becoming an independent electronics testing and measurement company in 2014.
“Name any piece of equipment in the market, whether it is the iPhone or a laptop, some component has been tested using Keysight,” said Sheth.
Learn more about Keysight AI Data Center Test Platform by watching the deep-dive presentations from AI Field Day at Techfieldday.com, or head over to keysight.com for the solutions brief.