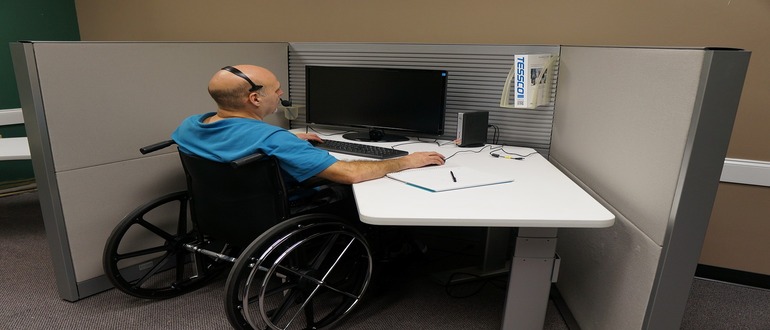
The U.S. Department of Labor released the AI and Inclusive Hiring Framework, a tool designed to support the equitable use of AI in hiring, and to benefit disabled job seekers.
Developed by the Partnership on Employment and Accessible Technology (PEAT), the framework aims to reduce the risk of unintentional discrimination and accessibility barriers when employers adopt AI-powered hiring technologies.
The framework highlights 10 key focus areas, providing employers with actionable goals and activities to integrate AI into disability-inclusive hiring.
It outlines how organizations can maximize the benefits of AI while managing potential risks for workers and job seekers, particularly those with disabilities.
The framework was funded by the Office of Disability Employment Policy (ODEP) and is based on the National Institute of Standards and Technology’s (NIST) AI Risk Management Framework.
The aim was to incorporate best practices for inclusive hiring, while input from disability advocates, AI experts and industry leaders shaped its development.
Stephen Kowski, Field CTO at SlashNext Email Security+, said employers could leverage the framework to enhance diversity and inclusion efforts by focusing on the 10 key areas it outlines, such as data quality and bias mitigation.
“They should prioritize accessibility and fairness in their AI-powered hiring tools, ensuring they don’t inadvertently discriminate against disabled candidates,” he said.
He added regular audits and assessments of AI systems can help identify and address potential biases, creating a more inclusive hiring process.
To integrate the framework effectively, Kowski recommended employers should start by mapping their current hiring processes and identifying areas where AI can be ethically implemented.
“Gradual implementation, coupled with thorough testing and employee training, can minimize disruption to existing systems,” he said. “Continuous monitoring and feedback loops will help refine the AI-driven processes over time, ensuring they align with both the framework and the company’s hiring goals.”
From Kowski’s perspective, striking the right balance between automation and human intervention in AI-driven hiring requires clear guidelines and oversight.
“Employers should establish checkpoints where human review is mandatory, especially for critical decisions or when dealing with edge cases,” he said.
He said he believes government frameworks like this can be valuable guides for businesses navigating the complex intersection of AI and inclusive hiring.
“While they may initially seem challenging to implement, they often lead to improved hiring practices and reduced legal risks in the long run,” he said. “The key is to view these frameworks as opportunities for innovation and improvement rather than as regulatory burdens.”
David Ly, CEO and founder of Iveda stressed the importance of proactive steps to address and reduce AI bias, urging leaders to implement comprehensive strategies throughout the AI lifecycle.
“Collecting diverse and representative datasets was crucial,” he said. “Data needed to include different demographic groups and perspectives to avoid excluding underrepresented or marginalized populations.”
Ly stressed rigorous data preprocessing as essential for fair AI outcomes.
“Leadership teams had to examine training data to actively identify and mitigate biases,” Ly added.
This included removing personally identifiable information, conducting statistical analyses and using data augmentation techniques to ensure balanced datasets.
Ongoing evaluation and transparency were also central to maintaining fairness.
“Regular monitoring could detect and rectify bias. Bias audits and transparency reports were valuable tools in this process,” Ly said.
He added that transparent AI processes built trust and accountability by allowing users to understand and challenge decisions, noting the role of diverse development teams was also critical.
“Inclusive teams brought diverse perspectives that helped identify biases early on,” Ly said.
He recommended interdisciplinary collaboration to address ethical concerns throughout AI development and advocated for regular bias testing.
“Bias testing throughout the development cycle helped ensure AI systems remained fair and unbiased over time,” he said. “These measures are necessary for fostering ethical, reliable and inclusive AI systems.”