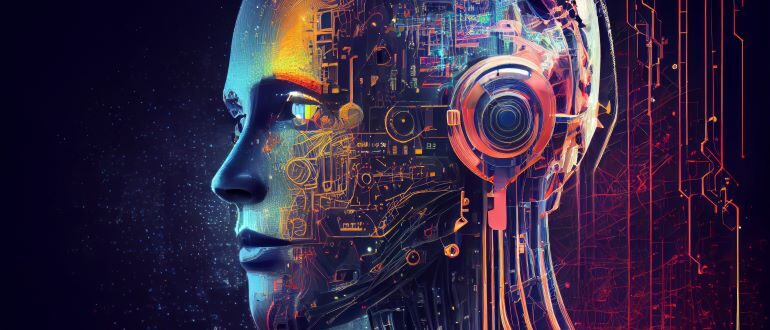
By 2027, 80% of the software engineering workforce will need to upskill in response to the rise of generative AI (GenAI), according to a Gartner study.
The research firm predicted that in the short term, AI tools would offer moderate productivity boosts by augmenting existing developer tasks, especially benefiting senior developers in mature engineering environments.
Slightly farther down the road, AI agents are expected to automate more processes, pushing the boundaries of development practices and leading to the era of AI-native software engineering, where most code will be AI-generated.
Long-term advances will further drive demand for specialized AI engineers with expertise in software, data science and machine learning, as organizations increasingly adopt AI-empowered solutions.
A previous Gartner survey of 300 U.S. and U.K. organizations reveals that 56% of software engineering leaders identified AI/machine learning (ML) engineers as the most in-demand role for 2024, with applying AI/ML to applications seen as the biggest skills gap.
Automating Tasks, Transforming Roles
Gartner senior principal analyst Philip Walsh said AI-native software engineering would transform existing roles by automating tasks and requiring new skills, particularly in AI orchestration and validation.
“While entirely new roles may not emerge immediately, the evolving responsibilities within current roles will be crucial in leveraging AI to drive innovation and productivity,” he said.
Walsh explained investing early in AI developer platforms offers several key benefits, significantly enhancing engineering teams’ productivity and capacity for innovation.
“One of the most immediate impacts is the improvement in both efficiency and value creation,” he said.
Michael Olaye, executive vice president and managing director of Hero Digital’s Western Region, said traditional engineer roles will become less about writing the actual code and more about prompting these AI applications to create the necessary code.
“For the next few years, the roles of low-level engineers are bound to reduce or even disappear entirely as AI takes on the mundane, repetitive, and low-level tasks directed by a more experienced engineer,” he said.
Still, with sophistication available through ML and more specialist tools becoming available within the development workflow, he said he fears many engineers’ roles in the future will look grim.
“We can expect the average size of a product, development, or QA team to be dramatically reduced to a handful of specialist support by their AI companion,” Olave said.
Aligning Siloed Workflows
Walsh explained by providing centralized tools and streamlined processes, AI platforms help developers respond more rapidly to business demands, allowing companies to create more innovative software leveraging AI.
“This drives efficiency while also unlocking greater business value,” he said.
He recommended organizations focus on aligning previously siloed workflows, such as DataOps, MLOps and DevOps.
By integrating these workflows, AI platforms promote better collaboration across teams, ultimately speeding up the development and deployment of AI-empowered applications.
“AI developer platforms help break down silos, improving both innovation and productivity,” Walsh said.
Finally, AI platforms unlock the full potential of AI engineering by establishing standardized, scalable processes for building and deploying AI-powered solutions.
“Early adopters of AI platforms can ensure their teams are equipped to deliver AI at scale,” Walsh noted, emphasizing the importance of these technologies for long-term success in the AI-driven landscape.
Keeping Humans in the Loop
Agustin Huerta, senior vice president of digital innovation for North America at Globant, said while AI can write code and accelerate coding tasks, human expertise will still be required to oversee strategic design and ensure AI-generated code aligns with business objectives.
“Software engineers need to focus on their ability to critically evaluate AI-generated code to identify issues and develop strong machine learning ML skills to fine-tune those outputs,” he said.
He explained creative problem-solving will not only help address issues like AI hallucinations, but also empower engineers to determine the right time for human intervention.
“In the long term, the engineering role will require more interdisciplinary skills, incorporating knowledge of AI ethics, system design and stronger business strategy for more efficient software development,” Huerta said.