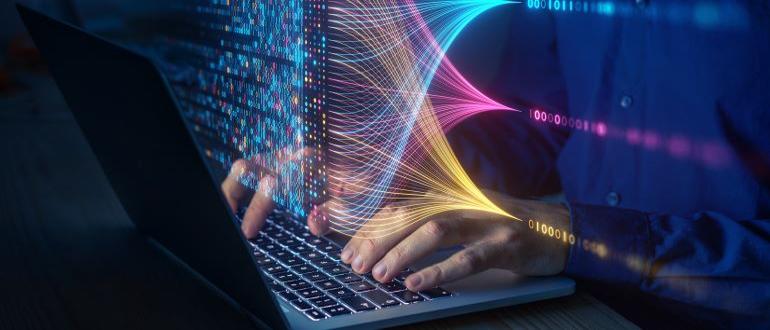
Artificial intelligence (AI) is ready to transform many industries, but most organizations find it difficult to scale their AI initiatives because of constraints with their data infrastructure.
Traditional data environments for structured data and conventional analytics simply don’t deliver the scalability, efficiency or integration capabilities that modern AI applications require. Organizations need to reimagine how they manage and optimize their data ecosystems to realize the full promise of AI.
The Limitations of Traditional Data Environments
One of the issues with current data environments is they’re built using traditional approaches, such as feature stores, which are mostly relevant for structured data. These setups don’t provide the foundation needed for AI applications like generative AI, natural language processing (NLP) and recommendation systems. AI requires much more: It needs a system that can analyze complex, unstructured data and contextual relationships.
Graph and vector data models break away from these rigid structures. They are better at capturing the complex relations between pieces of information — an essential task for AI. However, switching to this type of AI data infrastructure creates challenges. Designing a graph schema requires an upfront investment of time and effort to ensure it effectively surfaces the right linkage structures for a given use case. Embedding data in vectors is an intensive process that consumes a lot of memory and processing power. Scaling these systems is not easy. Organizations need to carefully balance performance demands and cost management to maintain efficiency without draining resources.
Many organizations also face hard choices when deciding whether to go with general-purpose AI models or specialized ones built for specific jobs. General-purpose models (such as OpenAI’s GPT-4) are versatile, but there are trade-offs in cost, accuracy and security. These models are generally trained on publicly-available data, potentially limiting their capacity to deliver accurate answers to domain-specific or highly-specialized queries. They also tend to “hallucinate” — where the model creates answers that sound legitimate but are wrong because they lack the right data.
Specialized models perform much better in their specific domains — whether it’s finance, healthcare or law. While they don’t allow for as much flexibility, these models give much more control over data privacy, accuracy and cost efficiency. The catch is that they need upfront investment and specialized know-how to build and maintain. For many organizations, determining which approach is right can be difficult, especially since the long-term costs and trade-offs aren’t always clear upfront.
Streamlining Data Integration for AI Systems
To build data infrastructure that’s ready for AI, organizations need to overhaul their entire data management strategy. One of the key areas to focus on is breaking down data silos within the organization. For AI to function effectively, data needs to flow freely between different departments and systems — nothing can afford to be isolated or locked away.
This is a necessary step to build data pipelines that continually update data for AI systems. AI models need data to be organized to work their best and return useful insights. Organizations should invest in advanced monitoring and profiling technologies to help optimize data quality.
Ensuring Security and Vendor Transparency
As organizations embrace AI solutions, it’s important they pay attention to how the vendors they partner with manage security. Large-scale AI models require access to vast amounts of data, which raises questions about how the data is handled, protected and used. They need to make sure their AI providers are transparent about their data practices, especially with sensitive information. An important question organizations should ask is how they handle training data — specifically, if they use private data in their general-purpose models. By carefully vetting AI providers and their security standards, organizations will minimize exposure to data breaches and other security incidents.
It’s also helpful for organizations to adopt AI in phases to remove some of the complexity and reduce risk. They should start with projects that are smaller in scope where they can afford the time to experiment with varying strategies. As they become more experienced, they build the confidence needed to take on bigger projects without worrying about the business impact of failing at a larger scale.
Measuring Success in AI Data Environments
Once AI solutions are in place, organizations need to monitor their performance. Specific metrics that support technical and business objectives should be tracked to see how adequate their AI data infrastructure is.
- Scalability: How well the system can handle growing amounts of vector data and queries without a drop in performance.
- Throughput: The number of queries processed per second indicates how the infrastructure handles a large real-time application workload.
- Cost-effectiveness: Assessing the overall cost of AI infrastructure ownership versus the value derived.
- Business impact: Improved areas of operation (better customer experience, efficiency and data-driven decision-making).
By balancing technical performance with measurable business outcomes, organizations can ensure their AI capabilities provide meaningful value and align with strategic goals.
AI initiatives depend on having a scalable, cost-effective and strong data environment. Organizations will achieve long-term success with AI by addressing current limitations, using modern data management best practices, and setting clear metrics, as outlined above. As AI accelerates, it’s time for IT and data leaders to rethink their data infrastructure to ensure they are ready for the future.