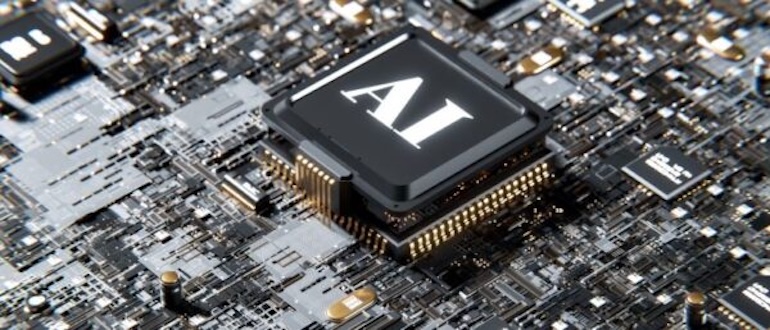
Business leaders are seeing unprecedented growth in data volumes that is driving up cloud storage demand pushing organizations to significantly expand their cloud-based storage estate.
A global Seagate and Recon Analytics survey of more than 1,000 business decision-makers found that 61% of respondents whose organizations primarily rely on cloud storage anticipate their storage needs to grow by more than 100% over the next three years. According to the survey, 72% of the respondents already use AI in their operations which underscores AI’s widespread adoption.
Storage, Security and Data Retention in AI Infrastructure
Among AI’s top infrastructure needs, security comes first, followed by storage, data management, network capacity, compute power, regulatory considerations, large language model viability, and energy efficiency.
The Seagate and Recon survey results indicated that AI is reshaping data retention practices: Among businesses using AI, 90% believe that retaining data for longer periods improves AI outcomes, while 88% emphasized that trustworthy AI requires increased data storage over extended periods.
“The only way these models compete is with cost-effective, data lake-type infrastructure that can scale to breathe life back into data that may previously have been on a shelf collecting dust,” said Jason Feist, senior vice president of global marketing at Seagate.
On top of that, organizations are collecting more insights through sensors for richer content.
Effectively managing data at scale will be a powerful differentiator, regardless of industry or geography. “Whether managed or deployed as a SaaS model, any data storage solution should be able to scale with the needs of the business as value from data evolves,” Feist said.
Best practices such as data tiering for appropriate architectural requirements to maximize capital investments, data governance for retention and compliance, and data security and encryption all help to extract value from the data stored and ensure continuous optimization.
Best Practices for Secure and Scalable AI
Feist added that proving provenance of AI-generated inference requires robust data security and encryption from data capture, formatting and tiering, computation, and eventually storage.
“Whether deploying a model as-a-service or developing a private, domain-specific solution, IT leaders should integrate data provenance and related retention models into their data governance processes,” he urged.
Guido Bartels, managing director, cloud at Xebia, recommended that organizations adopt secure-by-design cloud architectures, utilizing tools from leading cloud providers such as AWS, Azure, and Google Cloud to automate compliance, monitor anomalies, and secure data at rest and in transit.
“By prioritizing security alongside storage scalability, businesses can unlock the full potential of AI while safeguarding their data assets,” he said.
He further added that long-term data retention is vital for enhancing AI outcomes but requires a thoughtful approach to managing costs. Businesses must leverage AI-driven storage optimization tools to identify high-value datasets and automate tiering to cost-efficient cold storage when appropriate.
“Techniques such as deduplication and compression can further minimize redundancy and storage costs,” he explained.
With 88% of AI users emphasizing the need for trustworthy AI, Feist said developers can effectively demonstrate traceability, bias neutrality, and inference accountability by retaining sources of truth and model variation long-term.
“Think about how easy it is for humans to jump to conclusions based on limited information,” he said. “Often those assumptions based on limited information can lead to unintentionally poor outcomes. The more data you store, the more robust and trustworthy the outcome.”