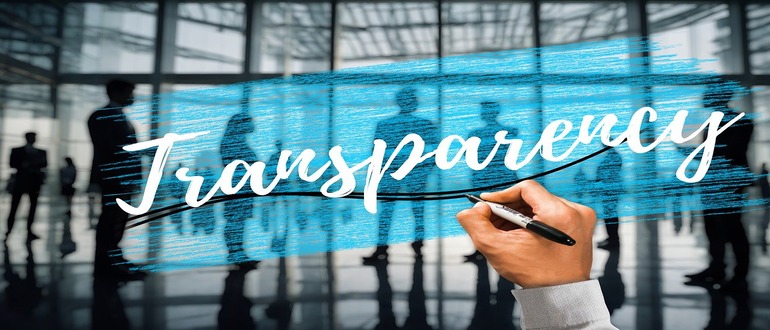
The rapid integration of Generative Artificial Intelligence (GenAI) into business and society presents an unprecedented opportunity for innovation, but it also demands heightened responsibility. In this context, transparency becomes one of the foundational elements of responsible AI, ensuring that we do so ethically and responsibly as we advance.
According to McKinsey’s 2024 survey on the state of AI, adoption has surged globally, with 72% of organizations now actively using AI—a significant jump from 50% in previous years. This widespread adoption amplifies the urgency for transparency to safeguard trust, compliance, and responsible innovation. Without understanding the “why” behind AI decisions, we risk deploying technology we can’t fully trust or explain.
Transparency is the foundation of responsible innovation and ethical AI, allowing us to see what an AI system does and why. This insight aligns AI with ethical standards and regulatory requirements, and builds trust among stakeholders. IBM research found that about 42% of enterprise-scale organizations (over 1,000 employees) actively use AI, with 59% planning to increase investment. As AI becomes integral to business operations, transparency is imperative to ensure rapid adoption doesn’t outpace responsible management.
Understanding the Multifaceted Nature of Transparency
Transparency in AI is not a single concept but a multi-dimensional imperative, encompassing both protection and explainability. In an era of increasingly stringent regulations, safeguarding sensitive information is non-negotiable. The consequences of failing to do so—whether through regulatory fines or erosion of trust—can be devastating.
Equally vital is the need for explainability, which ensures that AI decisions are not just outcomes but processes that can be understood and scrutinized. Many external or third-party models function as impenetrable black boxes, making it crucial for organizations to maintain control and visibility over how these systems reach their conclusions or use your data to further enhance their models.
Implementing Explainable AI Models
One significant challenge with external commercial AI models lies in their opaque decision-making processes. Without direct oversight, it becomes difficult to understand or explain how these models function, leading to a breakdown in trust and potential compliance risks. To address this, organizations should prioritize deploying AI models internally, within their firewalls, to maintain complete control over data and models. Leveraging open-source models like Llama and Mistral, customized with proprietary data, not only ensures data security but also strengthens regulatory compliance.
By integrating advanced techniques such as Retrieval-Augmented Generation (RAG), organizations can further enhance explainability by drawing clear connections between data sources and mitigating the risk of AI “hallucinations.” For example, in customer service applications, combining Large Language Models (LLMs) with RAG enables the AI to deliver accurate, contextually aware responses while referencing specific documents—significantly improving traceability and transparency in decision-making. The same is true for software development AI support, document management, and all sorts of use cases where information accuracy is a priority.
Maintaining Comprehensive and Accessible Documentation
Transparency extends far beyond the AI models themselves—it encompasses the entire ecosystem of processes that govern their use and your use of them. Comprehensive documentation is essential to fostering trust and accountability across an organization. However, it is critical to strike a thoughtful balance. Striving to document every data source, model choice, or potential bias can easily become an exhaustive exercise. Instead, organizations must prioritize meaningful documentation that captures the most critical elements of their AI systems, focusing on clarity and impact rather than volume.
Tools such as RAG models and knowledge graphs provide built-in traceability, automatically mapping where information is sourced and how data points are interconnected. This ensures decisions can be traced back to their origins, offering a clear audit trail.
Beyond the technical, policy documentation is equally critical. Every organization should establish robust, clear policies governing the use of AI and data protection. These policies must be overseen by cross-functional teams, including risk management bodies, legal departments, and AI ethics committees, to ensure that all dimensions—from technical to regulatory—are fully aligned. This holistic approach to governance ensures that transparency is not just a technical feature, but a foundational principle that permeates the entire organization.
Encouraging Open Communication and Stakeholder Engagement
Engaging stakeholders in AI transparency demands clear, strategic communication that resonates with their priorities. It is essential to move beyond technical jargon and present AI models in terms that highlight their business value—focusing on the quality of outcomes and measurable economic impacts. Stakeholders need to understand how AI initiatives align with critical concerns like data privacy, security, and regulatory compliance, particularly with data remaining securely within the organization’s firewall. By framing AI in the context of business objectives, leaders can foster trust, drive alignment, and ensure that AI efforts are seen as integral to long-term success.
Positioning AI as another employee can be effective—viewing AI as a team member to be managed and trained makes it more tangible. Existing data handling policies for employees can logically extend to AI systems, helping stakeholders grasp the importance of data protection and AI’s role within the organization.
Organizational Culture and Ethical AI
An organization’s culture significantly influences AI adoption and utilization, reflecting its values and actions. Some companies may avoid AI due to risk aversion, but this will most certainly hinder competitiveness in a rapidly evolving market.
Many once-prominent organizations have faced challenges over time due to difficulties in adapting to changing market demands. Even historically dominant brands, like those in mobile devices and photography, have sometimes found themselves struggling to remain relevant in a fast-evolving landscape.
Today, companies must proactively embrace ethical AI by developing strategies that balance innovation with transparency, data protection and compliance. Establishing a culture that prioritizes data privacy and transparency should be universal, while specific AI applications can be tailored to individual teams or departments. This balance promotes flexibility and encourages safe, rapid innovation.
Best Practices for Integrating Transparency Into AI Practices
To integrate explainable AI effectively into operations, focusing on areas where AI can deliver immediate value with minimal risk is crucial. Non-sensitive data applications are an ideal starting point. For instance, customer service centers provide a fertile ground for AI integration, enabling systems to manage complex interactions, deliver timely and accurate responses, and perform real-time sentiment analysis. Since these processes typically don’t involve sensitive data, they minimize privacy concerns while still showcasing AI’s capability.
Another promising application is entity extraction for document analysis. Organizations often manage vast amounts of unstructured data, and AI can help organize and interpret this information. When AI’s outputs are verifiable against original documents, it enhances both accuracy and traceability—two pillars of transparent AI.
An emerging best practice involves AI playing multiple roles within an organization, acting as a creator, checker and reviewer. This approach ensures not only the generation of outputs but also their evaluation against criteria like bias, accuracy and completeness. When AI is utilized to assess its own performance, it enhances reliability and fosters trust within the organization.
At this critical juncture, the future of AI within enterprises will be shaped by a commitment to transparency, ethical practice and responsible innovation. As adoption accelerates, embedding transparency into AI strategies is not just a compliance exercise—it is the key to building lasting trust, driving accountability and securing a competitive advantage.
Organizations that harmonize innovation with responsibility will not only thrive in the evolving tech landscape but will also contribute meaningfully to societal well-being. The challenge is clear, and the opportunity is immense: Lead with integrity and innovate with purpose.