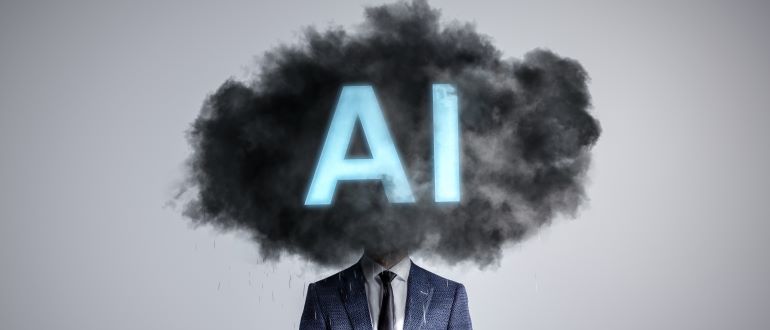
Today’s cybersecurity hinges on the ability to detect and respond to authentication anomalies swiftly and accurately. Artificial intelligence (AI) and machine learning (ML) have emerged as powerful tools for identifying unusual patterns in user behavior and flagging potential threats in real time. These technologies analyze vast amounts of authentication data, learning from previous attacks to better predict and mitigate future risks. By automating anomaly detection, AI and ML enhance the efficiency of security systems, reducing the need for human intervention while improving overall security. This approach allows companies to stay ahead of increasingly sophisticated cyberthreats and avoid potential breaches that can cause significant financial and reputational damage.
AI and ML as the Present and Future of Anomaly Detection
Anomaly detection flags suspicious attempts to access personal, financial, medical or other sensitive information and is the first line of defense for any cybersecurity threat. Traditionally, anomaly detection tracks abnormal user behaviors (like excessive login attempts, multiple password resets, or unknown devices) to identify malicious activity and protect user data. Third-party authentication, verification from backup accounts, account lockouts and fraud alerts are all standard first-line protections that rely on anomaly detection.
Traditional anomaly detection tools are becoming outdated as cyberthreats become increasingly common and complex. In fact, according to Microsoft’s 2024 Digital Defense Report, there has been a 79% increase in indicators of attack (IOA) since 2020 related to cyberattacks.
In response to this uptick in threats, major industry players have already shifted to AI- and ML-based anomaly detection software. Mastercard, for instance, developed an in-house anomaly detection system that uses AI to analyze billions of network transactions to detect, prevent and predict fraudulent transactions in real time. Many companies employ similar AI technologies, from Cisco’s zero-trust security model to Uber’s uVitals alert system. Even everyday users are becoming less reliant on traditional methods for anomaly detection. In a Delinea survey, 79% of participants agreed that traditional passwords are on track to “becoming obsolete” and rely on other methods to secure their data. These examples underscore the obvious need for organizations to get serious about how their anomaly detection utilizes AI and ML technologies and understand why the shift is necessary.
How AI and ML Improve Anomaly Detection
AI- and ML-based adaptive pattern recognition are at the heart of improving anomaly detection. Companies can use this cutting-edge, paradigm-shifting AI technology to help anomaly detection algorithms “learn” from past experiences and adapt to constantly shifting contexts and conditions. By using adaptive pattern recognition, AI, and ML, organizations can improve their anomaly detection systems significantly, including:
- Continuous learning. AI and ML technologies help anomaly detection systems constantly integrate new data based on user behavior. This approach allows security systems to more accurately and efficiently identify when a transaction is authentic, malicious or fraudulent by creating a more nuanced metric for anomaly scores.
- Enhanced biometrics. Biometrics, like fingerprint and iris scans, face recognition, and voice recognition, are already an integral part of cybersecurity, and the biometrics industry is forecasted to be valued at $150.58 billion by 2030. By integrating adaptive pattern recognition and biometrics, anomaly detection can enhance security by introducing new factors for authentication—including behavioral biometrics such as scrolling habits, typing speed, and login frequency.
- Increased response times. Using AI, ML and adaptive pattern recognition to automate security alerts and notifications facilitates faster notification of cyberattacks. Detection systems rooted in these technologies can immediately identify anomalies based on past user behaviors and generate custom protections and suggestions based on the anomaly’s surrounding context.
- Predictive threat detection. This is one of the most important cybersecurity developments. Adaptive pattern recognition can learn from the past and anticipate future threats with predictive analytics. Companies using adaptive pattern recognition can detect even the newest, most novel cyberthreats that would otherwise bypass detection systems.
These outcomes improve overall security and increase user confidence and ease of use. With such clear incentives for using AI and ML for anomaly detection, it’s crucial for companies to understand how and when to integrate these technologies into their systems.
Considerations for Integrating AI- and ML-Based Anomaly Detection
Before deciding to invest in AI and ML, company leaders need to determine clearly defined objectives for what they want these technologies to do for their business, as well as why meeting these objectives is essential. These answers will largely depend on a company’s scale, the sensitivity of its user data, and how prone it is to cyberattacks.
Company leaders can also assess their current protections on sensitive data and their current infrastructure’s compatibility with new AI and ML models. Attempting to integrate new AI and ML systems without understanding their compatibility could lead to serious, costly obstacles. When in doubt, it’s best to ask a cybersecurity expert to assess whether AI or ML is a necessary and advantageous investment.
A New Era for Anomaly Detection
With AI and ML actively reconfiguring the modern techscape of cybersecurity, it’s essential for companies to understand what these technologies offer regarding anomaly detection, data security and user confidence. Companies can leverage AI and ML’s continuous learning from past authentication anomalies and other important data sets to strengthen the security protocols around sensitive data, simultaneously speed up threat detection, increase user confidence, and save costs.
That said, it’s vital for any company to assess the need and use cases for AI and ML before integrating them into its cybersecurity systems and operations. While there are many benefits to AI and ML anomaly detection software, it’s critical for organizations to consider how they will adapt to the ever-evolving cybersecurity landscape.