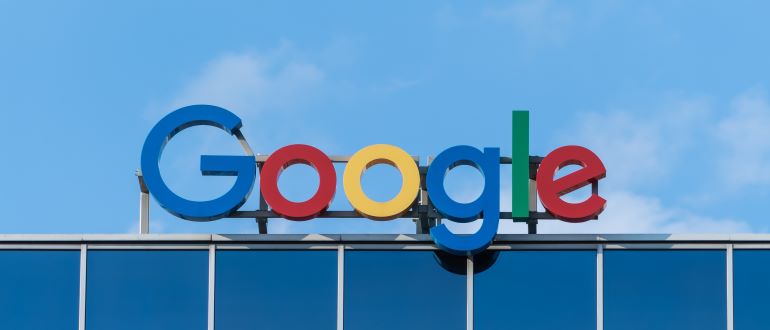
Google today, as part of a raft of updates to its cloud services, made available a preview of an update to its Looker business intelligence (BI) application that leverages Gemini generative artificial intelligence capabilities to enable end users to employ natural language to query data and generate reports with much fewer constraints.
Additionally, Gemini can be used within Google Slides to now generate charts and summaries in seconds without having to move data, and has added a Formula Assistant that can create calculated fields based on the metrics and dimensions exposed within any data set.
These capabilities in effect remove the limitations imposed by BI dashboards that could only be used to iteratively interrogate a narrow range of data that had been previously loaded into Looker, says Gerrit Kazmaier, general manager and vice president of data analytics for Google Cloud. “You can now have free-form questions,” he says.
Over time, as much as 70% of BI tasks will likely be automated by generative AI, adds Kazmaier.
Announced at a Google Cloud Next Tokyo 24 event, these updates to Looker are being made alongside a raft of other updates to Google Cloud services that include an Analytics Hub for processing streaming data and adding Gemini generative AI capabilities to its BigQuery data warehouse to automate data engineering tasks. BigQuery for the first time now also supports SQL.
In addition, Google is making available instances of open-source Apache Spark and Apache Kafka platforms on serverless computing platforms. End users can now as a result analyze structured, unstructured, and open-format data using SQL, Spark and AI models accessed via the Google Vertex service.
Google is also now making available Microsoft SQL server databases on its cloud service and is expanding in the processing capabilities and full-text search to its Spanner graph engine.
Google Also Adds Data Migration Services
Finally, Google is adding data migration services that promise to accelerate time to value while also reducing data egress costs.
As generative AI continues to evolve many of the silos that previously made querying data might soon give way to AI models that in effect are normalizing data in a way that makes it simpler to analyze. That capability may not eliminate the need for traditional programming tools such as SQL but over time more data will be interrogated using prompts that are increasingly being embedded into multiple platforms.
Additionally, AI agents are also being added to automate the management of specific tasks that previously required manual effort. Rather than performing those tasks, the IT teams that manage these platforms will be supervising tasks assigned to multiple AI agents that have been trained to optimally manage various classes of data.
It may be a little while before the way data is processed, analyzed and managed fundamentally changes in the AI era, but it’s already apparent that surfacing actionable insights from all the data collected by an organization is going to become simpler. The challenge now will be teaching end users how to get the most out of a set of new interfaces that fundamentally change the way they interact with data.