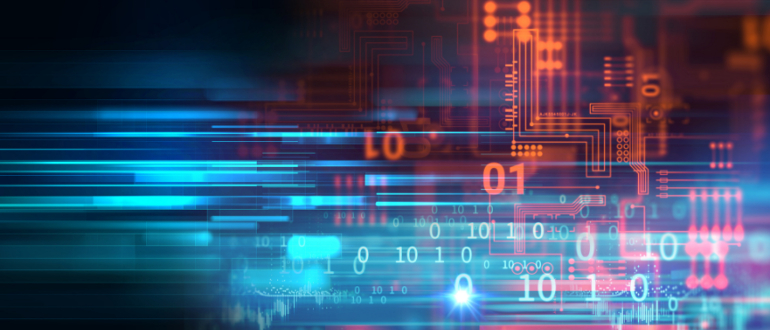
Organizations embarking on artificial intelligence (AI) projects cannot have long-running data centralization projects. They need to be able to integrate high-quality data as quickly and as efficiently as possible, from anywhere into their AI tools to deliver contextually-accurate outputs.
For many organizations, this movement of data is in full swing from cloud-based storage to on-prem systems. But data repatriation is neither cheap, nor easy. Typically, it is accompanied by a hefty cost, thanks to the volume of data organizations create and transact now and the growing pains of data management. Even at a few cents per gigabyte, the costs add up quickly. Bill Burnham, CTO for U.S. Public Sector at Hewlett Packard Enterprise notes that costs can grow “astronomically” as organizations move into processing petabytes of data.
In AI applications, particularly, where new data is used to refine and update outputs, hauling data back to on-prem storage makes economic sense. And from an operational standpoint, it is ideal to place data as close as possible to where it will be used. It is also critical that one has access to the most recent, accurate data for model training.
Safeguarding AI Data and Outcomes
A Gartner research suggests that cloud service misconfigurations are a significant cause for exposure of sensitive data to unauthorized AI models. Just as end-users are cautioned that the queries they submit to public generative AI (GenAI) services can be used, this scenario applies to any data that is exposed. On-prem systems are not immune from data breaches, but the risk of an unauthorized AI model accessing corporate data resulting in an intellectual property leak can be mitigated.
Inaccurate results remains another significant issue. Recent cases where Google’s AI suggested that cooks use glue to make cheese stay on a pizza and eating a rock each day as source of vitamins and minerals, reflect how important it is to populate LLMs with appropriate and contextual data. By using internal data and making it available quickly and cost-effectively to the models, the risk of misleading or erroneous results can be greatly reduced.
Similarly, the importance of contextual information cannot be understated. The best data an organization can use for AI tools is data that specifically pertains to what you do. For example, an apparel retailer needs to plug in data about the demographics they serve. A clothing outlet that focuses on females age 16 to 25 needs different inputs than one that sell suits to men in the range of 35 to 50 yease. AI models that ingest general data and do not understand the specific needs of the business only deliver outputs that lead to poor decisions. Case in point Google AI’s suggestions.
Bringing Your AI On-Prem
Putting data as close as possible to where it will be used for AI reduces complexity and costs. AI projects are highly dependent on the data used to train the models. Having high-quality, data close at hand is more valuable than hiring more data scientists. Accordingly, organizations must prioritize data and accessibility over less important things.
Managing this data for AI applications typically involves copying sets from the source. But when the best data is distributed across multiple platforms like cloud-based CRM, on-prem finance platform and online productivity tools, it can be challenging to assimilate information, often resulting in choosing the most accessible datasets over the others.
The question AI teams need to ask is how they can access all the data they need without having to wait for costly and time-consuming data repatriation processes.
An easy way to access disparate data in multiple locations with the ability to redirect queries that access that data as data is moved could solve this problem. Data preparation tools can condition data for AI activation while minimizing disruption during repatriation. These new state-of-the-art approaches can turbocharge AI projects without the need to significantly re-engineer systems or wait for data migration. Training data can be plugged into AI models and LLMs as it’s created in near real-time.
Spiraling costs, concerns of IP leakage and need for greater agility in development are driving the shift from cloud-based platforms to on-prem. AI and LLMs demand access to high-quality, contextual and timely data to ensure they deliver the best results for users. An AI data hub that is the single, central workbench and governance zone for all AI and data integration projects enables acceleration of AI projects in parallel with cloud repatriation projects so organizations can rapidly leverage their data, and at the same time continue to give business users better customer insights and advanced analytics to boost revenues and beat competitors in an extremely competitive business environment.