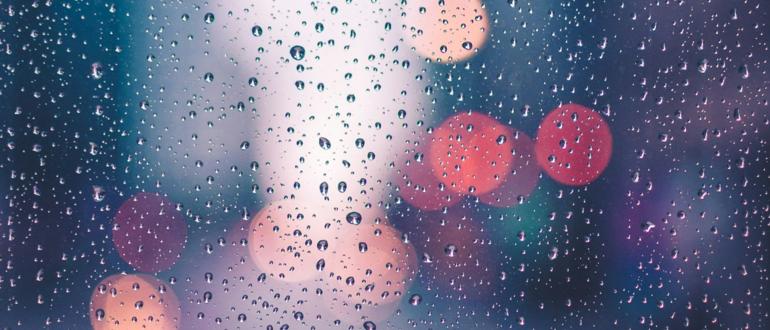
Let’s face it: Climate change is the ultimate party crasher. It’s here, it’s real, and it’s not leaving anytime soon. But while we’re busy arguing about whose fault it is (spoiler: it’s all of us), the planet is heating up faster than a microwave burrito. Here comes artificial intelligence (AI), the tech world’s golden child, to save the day (or at least help us understand what’s going on in the trillions acres of our global backyard)!
Traditional climate models? Yeah, they’re fantastic and all, but they’re like trying to predict the weather with a Magic 8-Ball. They’ve been very functional but have remained largely foundational and have missed the mark. But advanced AI has rightly come to the party and absorbed the non-linearity present in the systems. It has donned the hat of Sherlock Holmes of climate science, piecing together real-time cues and clues from a massive volume of data streaming from the satellites and sensors, and historical records to solve the mystery of our planet’s future. From simulating atmospheric dynamics to creating hyper-localized forecasts, AI-powered climate tech is opening us to new possibilities for understanding and addressing climate change on an unprecedented scale.
So, grab your buttered popcorn (preferably not microwaveable, because emissions!), and let’s dive into how AI is revolutionizing climate modeling – of course on a lighter note because what’s ensuing is pretty mystical!
The Intricacies in Climate Systems: A Hot Mess of Chaos
Climate systems are a tangled web of natural processes, much like soap operas, which are dramatic and unpredictable. The atmosphere, oceans, land and ice are all characters in this never-ending saga, each influencing the others in ways that make your head spin. For example:
- Ocean currents shift, and suddenly you’ve got hurricanes throwing tantrums.
- CO₂ levels rise, plants get stressed and carbon absorption goes haywire, creating a vicious cycle.
AI, particularly machine learning (ML) and deep learning (DL), offers powerful tools to unlock these complex interactions. Unlike the traditional models that rely on rigid equations (think: your high school math teacher), AI learns directly from data, spotting patterns and relationships that even the smartest scientists might miss. For instance, deep learning models have been trained to predict temperature changes and rainfall patterns by analyzing historical data, uncovering insights that traditional models overlook. It’s like having a crystal ball – but one that actually works.
Data Preprocessing and Multimodal Fusion: Cleaning up the Climate Mess
Climate modeling relies on diverse data sources such as satellite imagery, IoT sensors and historical data. But here’s the catch: this data is often messy, noisy and incomplete. It’s like trying to bake a cake with half the ingredients missing and a recipe written in hieroglyphics. Thankfully, AI is here to clean up the mess.
AI excels at cleaning, standardizing and filling gaps in data. Take Google’s Earth Engine, for example. This AI-powered tool integrates and analyzes massive geospatial datasets, allowing researchers to monitor environmental changes in real time. There’s your super-organized friend who color-codes your closet and labels everything.
AI also enables multimodal fusion, which is a fancy way of saying it combines data from different sources to create more comprehensive climate models. A study published in Nature demonstrated how integrating satellite data, ocean temperature maps and wind patterns improved hurricane tracking, leading to more accurate forecasts. So, next time a hurricane hits, you can thank AI for the heads-up and maybe invest in some better shutters.
Enhancing Climate Simulations with AI: Faster, Smarter and Way Cooler
Simulating climate phenomena like cloud dynamics and ocean currents has traditionally been a computational nightmare. It’s like trying to run a marathon while solving a Rubik’s Cube! Very exhausting and time-consuming. AI tools like convolutional neural networks (CNNs) and generative adversarial networks (GANs) are changing the game:
- CNNs don’t just recognize cats and dogs, they also decode clouds! These deep learning models analyze high-resolution satellite images to track shifting cloud formations and predict precipitation patterns. NASA even put CNNs to work simulating atmospheric water vapor distribution, slashing computational time by a whopping 80%.
- GANs aren’t just creating deepfake celebrities, but the weather too! These AI models generate synthetic climate scenarios, including rare extreme weather events, giving disaster planners a sneak peek at Mother Nature’s cranky moods. They also fill in the gaps for regions with sparse data, helping local governments prepare for storms they’ve never seen but AI has imagined.
By enabling quicker and more accurate simulations, AI allows scientists to test climate interventions, like geoengineering solutions, in virtual environments before implementing them in the real world. Think of it as a climate change sandbox mode. Absolutely no consequences, but a lot of learning!
Improving Prediction and Managing Uncertainty: Because the Weather Loves to Mess!
Predicting climate patterns is inherently uncertain, thanks to the chaotic nature of climate systems. It’s like trying to predict your cat’s mood. Good luck with that. But AI is stepping up to the challenge.
- Ensemble Learning is like getting a weather forecast from a whole panel of AI experts instead of trusting that one overly optimistic meteorologist. By blending predictions from several models, it boosts accuracy and reliability. The European Centre for Medium-Range Weather Forecasts (ECMWF) takes this a step further, leveraging AI to fuse outputs from several traditional models, making 10-day forecasts less of a guessing game and more of a science. Because sometimes, one AI’s best guess isn’t enough to decide if you should pack sunscreen or a snorkel!
- AI-driven probabilistic models don’t just predict the weather but rather they predict how confident they are about predicting the weather. These models quantify uncertainty, offering confidence intervals that help policymakers assess risks and plan ahead. Think of it like a forecast that says, ‘There’s a 70% chance of rain… but we’re only 60% sure about that.’ Not exactly reassuring, but at least it’s candid!
Hyper-Localized Climate Modeling: Because Your City Is Special!
Global climate models (GCMs) often struggle to capture regional climate nuances due to their limited resolution. There’s no way trying to describe the taste of pizza by averaging every flavor in the world. Ain’t gonna work! AI overcomes this by enabling hyper-localized, high-resolution predictions:
- Your city will now get a personal trainer. Because cities create unique microclimates due to infrastructure, energy use and human activity. And AI models trained on urban sensor networks can predict hyper-localized heatwaves, guiding measures like expanding green spaces or installing reflective rooftops.
- AI systems like IBM’s Green Horizon use machine learning to forecast air quality levels and issue targeted warnings to at-risk communities. This approach was successfully tested in Beijing to combat and reduce air pollution. So, if you’ve ever been to Beijing lately and had breathing easier, you know who to thank.
Localized modeling is crucial for climate adaptation, giving regional authorities the precision they need to tackle specific challenges. So, it’s like custom-fit raincoat of climate forecasting instead of a one-size-fits-all poncho that leaves everyone at least half-soaked!
Ethical and Practical Challenges: Because Nothing Is Perfect
Despite its potential, AI-driven climate modeling isn’t without its challenges. It’s still the superhero that’s battling a few kryptonite weaknesses: Here’s where it struggles:
AI might not have perfected its climate superpowers yet, but the good news? Its weaknesses are fixable, but only if we engineer the right solutions.
- Data Biases: If an AI model trains mostly on Western climate data, expecting it to predict weather patterns in Southeast Asia is like asking a snowman to handle a heatwave. It probably won’t end well. Bias in datasets can lead to skewed predictions, leaving some regions underserved and ill-prepared.
- Energy Intensity: Training these massive AI models eats up computational resources and contributes to carbon emissions. Researchers are now working on low-energy solutions like edge computing and sparse models. It’s like trying to burn calories while eating cake. Tough, but not impossible!
- Explainability: Many AI models are still frustratingly black-boxed, making it hard to figure out how they reach their conclusions. It’s like trusting a weather app that just says “Rain. Maybe.” Transparency is key to ensuring policymakers and scientists actually trust AI-driven forecasts.
Addressing these challenges requires collaboration among multiple stakeholders including the policymakers, climate scientists and AI developers to ensure that solutions are transparent, sustainable and equitable. We’re trying to assemble the Avengers, but for climate change.
The Future of AI in Climate Modeling: Buckle Up, Wild Ride Ahead
Looking ahead, AI will continue to transform climate modeling in exciting ways:
- Quantum Computing: Combining AI with quantum algorithms could substantially speed up climate simulations, enabling real-time predictions for disaster response like upgrading a bicycle to a rocket ship.
- Real-Time Streaming Analytics: As IoT devices proliferate, AI systems will process real-time data streams to provide faster updates on weather conditions, improving preparedness for extreme events like hurricanes and wildfires.
- Global Collaboration: AI-powered platforms like ClimateAI are fostering international cooperation, allowing researchers worldwide to share a huge potluck of data and uncover insights to combat climate change holistically.
By integrating AI with emerging technologies like blockchain for carbon emission tracking or drones for real-time data gathering, we can make climate modeling a cornerstone of global sustainability efforts. That’ll make AI a high-tech fortress to protect the planet.
AI as a Catalyst for Climate Solutions
AI isn’t just forecasting the future but it’s also helping us rewrite it. From fine-tuning climate simulations to delivering hyper-local predictions that make umbrellas (almost) obsolete, AI has the power to revolutionize how we interpret, predict and fight climate change.
But let’s be real, AI isn’t a magic wand. It needs clean data, ethical oversight and real-world collaboration to ensure it works for everyone, not just the well-mapped parts of the world. Like any powerful tool, it’s not about whether AI can make a difference, but how smartly and swiftly we use it before the climate clock runs out.
So, our forecast here? With AI, there’s a strong chance of innovation, but that’s only if we bring the right conditions.