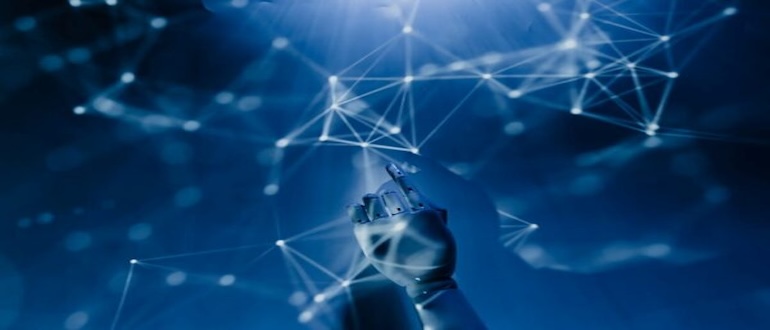
Enterprises that are investing heavily in AI to drive productivity and decision-making are under immense pressure from boards and shareholders to see these investments pay off. To realize max gains, they must answer one key question: Despite having access to the same foundational AI technologies as every company, how can they differentiate themselves and gain an edge?
The Challenge of Differentiation
Last year, the global spend on AI reached $166 billion, a figure that is projected to more than double reaching $423 billion by 2027, according to IDC. It is obvious that companies understand the urgency to make these investments, but only 43% of leaders say their organizations are good at delivering differentiated products and services.
To understand the challenge of differentiation, imagine you’re an auto maker who invests $1 million in a generative AI solution to help market vehicles precisely to potential customers. Your three biggest rivals build a similar solution. Maybe some people who hadn’t planned to buy a car would buy one, creating a marginal gain. But you’ve really each spent $1 million to arrive back at a level playing field. Now, the investment is important to keep up, but it doesn’t differentiate.
This is the reality with AI companies. A majority of enterprises today are using OpenAI’s GPT-4 as their foundational model, mostly through Microsoft’s Azure AI service. Anthropic, Mistral and Cohere are also popular but less widely used. The performance of these foundational LLMs doesn’t differ widely, which basically means they are all using the same technology. You could compare it to using an Oracle or IBM database – the technology is broadly similar and as a result, doesn’t offer significant differentiation by itself.
So how do they create a significant competitive advantage? There are two main vectors to think about here: one is technology differentiation – for example, the type of applications developed and the cost of training and operating those applications. The other is business differentiation – example, how AI is made available to employees, and how bold the company’s leadership is in developing its products and services. That last point can be the difference between a 10% improvement and a 10x improvement in business.
Differentiation at the Application-Level
While every business has access to the same models, they don’t have access to the same data. In many cases, companies can build applications that are better adapted to their particular industry by using proprietary data they have collected and acquired over the years. This may be customer data, operational data, financial data, security data, or something else.
But achieving differentiation requires more than just connecting this data to an AI model. Rather, it’s about applying the data to more advanced and predictive models, or using AI to create useful, structured data from messy maintenance or customer support logs to improve customer service. Leveraging proprietary data to build these deeply customized applications that address specific organizational needs is a powerful way to create unique competitive value.
Differentiation through Cost Efficiency
Generative AI (GenAI) is relatively less costly to implement, and presents opportunities to differentiate through cost efficiency. If an organization builds a similar GenAI solution to a competitor but operates it at 70% of the cost, that could be a significant gain.
Choosing the right model for the right application is key. Not every application requires a big foundational model like GPT-4. A smaller model that provides sufficient capabilities for the task at hand will reduce operating costs, or CPUs can be sufficient for certain tasks, particularly inference work, for certain applications.
The decision whether to train and operate AI applications in the cloud or use on-premises infrastructure can also make a big difference. Some enterprises are finding that the costs of data storage, network bandwidth and compute required for generative AI make self-hosting more economical.
Allow Pockets of Innovation and Micro-Revenue to Flourish
One of the promises of generative AI is that it allows employees to access and query data without specialized knowledge or skills. This could mean that at some point in the future, there will no more need for a dedicated data scientist or data engineer to build a dashboard or write SQL queries; the natural language interface will be sufficient to ask complex queries and get instant answers.
This is important because data professionals, while amazing, don’t know the business as well as the accountant sitting in the CFO’s office or the analyst who reports to the logistics manager. Businesses that make AI tools widely available to their employees to create the opportunity to uncover new insights or new workflows that improve business outcomes.
Inside large companies, innovative ideas die often because they’re too costly to develop, prototype, test and bring to market. Generative AI starts to reduce these costs, allowing micro innovations to blossom and create pockets of revenue that may eventually become much bigger.
The underlying need is to get AI tools in the hands of employees to see what they can make happen. This obviously needs to happen in a structured, organized way that ensures data governance and data privacy policies are maintained.
10% Gains Versus 10x Gains
The marketing example above may yield a marginal gain – a “10% improvement” – and that is happening across companies and industries. Enterprises are hard at work using AI to squeeze out supply chain efficiencies, improve customer service and decrease failure rates in manufacturing. But the ultimate differentiation comes in the form of a “10x improvement,” something that changes how an industry works entirely.
We’ve seen such transformative gains from other technologies as well. The cloud upended the software business when Marc Benioff created Salesforce. The smartphone upended the taxi business when Apple created the iPhone. History is littered with companies that were sidelined by new waves of technology — Kodak by digital cameras; Blockbuster by video streaming. The same will happen with generative AI.
These 10X gains provide the ultimate in differentiation, and we’re starting to see the first signs of them now. The restaurant chain Sweetgreen’s has used AI to create its first automated kitchens, which it says will reduce labor costs by 67% and increase salad production by 50%, with the potential to drive a seven-percentage-point margin expansion. DuoLingo credits generative AI for a 54% increase in paid subscribers.
These gains are significant, but they are still arguably improving existing business models rather than creating new ones. We don’t know yet where the biggest disruptions will come from, but we’re barely two years into this latest wave of AI innovation. The companies profiting the most today are providing the picks and shovels – the LLMs, GPUs and cloud infrastructure. The broader opportunity from AI lies in the new business models and product categories that will be created, and that requires a willingness to experiment and be bold.
Be a Disruptor
The wide availability of foundational AI models is a double-edged sword. Businesses have new opportunities to innovate, but so do their competitors. Capitalizing fully on this latest wave of AI development means deploying the technology in ways that are differentiated and create meaningful business outcomes. Like the cloud and mobile computing before it, AI has the potential to radically disrupt industries. The biggest gains will go to those who unleash the creativity of their employees and place bold, strategic bets on new use cases and business models.