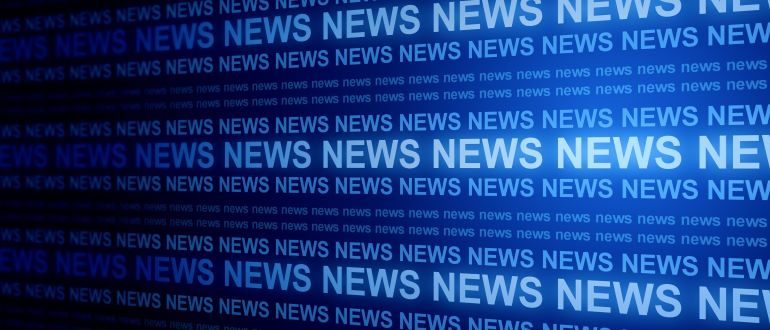
VAST Data this week during a Cosmos virtual event revealed it will, in collaboration with NVIDIA, deliver a platform specifically optimized for uses cases requiring regenerative augmented generation (RAG) early next year.
In addition, the company extended an existing alliance with Cisco to enable the VAST Data platform to natively run in Unified Compute Systems (UCS). Previously, the two companies integrated the VAST Data platform with the Cisco Nexus HyperFabric AI, which combines Cisco’s 800G Ethernet fabric software with NVIDIA AI Enterprise and NIM inference microservices to create a network of clusters running AI models across CPUs, graphical processor units (GPUs) and data processing units (DPUs) provided by NVIDIA.
Finally, VAST Data has launched an effort to build a community for artificial intelligence (AI) practitioners of all skills level.
Aaron Chaisson, vice president of product and solutions marketing at VAST Data, said the turnkey VAST InsightEngine platform that will be delivered next year will be able to extend large language models (LLMs) using massive amounts of data processed in real time. That approach will enable organizations to extend LLMs without compromising the overall user experience, he added.
The overall goal is to democratize RAG by eliminating the need for organizations to integrate disparate technologies themselves, noted Chaisson.
At the core of the VAST InsightEngine is the company’s existing VAST platform for processing data, including vector and graph embeddings, and the NIM microservices framework developed by NVIDIA.
A VAST DataBase that is included with the platform can process an ingestion of exabytes of file, object, table or streaming data in milliseconds, added Chaisson.
In addition, because all data indexing happens at the source, organizations do not have to additionally invest in extract, transform and load (ETL) processes and data lakes to normalize data before exposing it to an LLM, he noted.
Finally, the platform ensures that any file system or object storage data update is atomically synchronized with an embedded vector database and its indices to both ensure data consistency and secure data access management, said Chaisson.
It’s not clear how many organizations will need to process data in real time to extend LLMs versus relying on batch-oriented processing. However, most end users expect to be able to interactively engage with responses to prompts versus waiting, for example, multiple seconds for an LLM to process external data before responding. The challenge, of course, is making sure that relevant data is made available to LLMs as it is constantly being updated.
Undoubtedly, as more turnkey approaches to RAG become available, more responsibility for managing these platforms will shift toward IT operations teams. In the meantime, however, many organizations continue to create dedicated teams to manage all the platforms and processes required to manage RAG workflows.
Each organization will need to determine which approach makes the most sense for them, but the one thing that is certain is the variety and volume of data that organizations will need to manage is going to continue to exponentially increase in the age of AI.