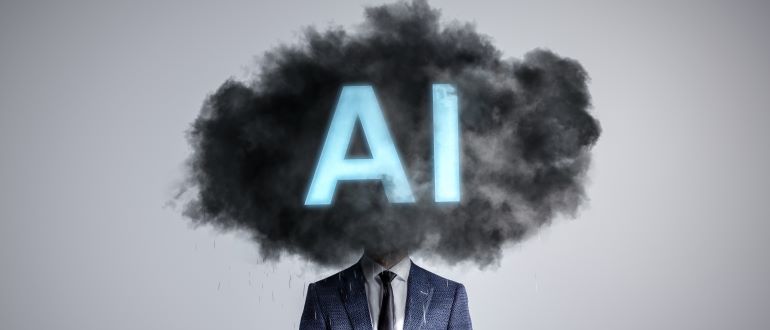
Fraud is a prominent concern in investment operations. Firms and individual investors alike must spot scams, forged documents or fraudulent transactions to avoid catastrophic losses, but that is often easier said than done. Artificial intelligence (AI) makes it easier.
Of the 93% of financial institutions planning on investing in AI, 63% cite fraud detection as their primary reason. That makes anti-fraud processes the most popular AI application in the financial sector. This shift is more than a passing trend. As more investment operations implement AI fraud detection, investment scams will become far less successful, saving money and boosting consumer trust.
How AI Fraud Detection Works
At its core, AI fraud detection works the same way conventional anti-fraud measures do. Organizations identify potential fraud by looking for details or unusual behaviors. However, automating this process through AI makes it more reliable and efficient.
Anti-fraud AI solutions typically employ clustering algorithms to enable outlier detection. Feeding these models examples of legitimate transactions, customer behavior or investment documents lets them group similar cases, with each cluster representing a different baseline for normal conditions. Then, any new data that does not fit into these clusters stands out as potential fraud.
Supervised machine learning methods can also be effective. Random forests can spot fraud with up to 96% accuracy, but the need for labeled data can hold them back. Clustering has an advantage in that it determines its own ways of classifying legitimate cases, which may reduce human error and improve real-world versatility.
Benefits of AI in Fraud Detection
Whichever machine learning technique a company uses, AI fraud detection has several advantages over conventional methods. First, it removes biases like overconfidence from the equation, resulting in a more objective analysis. This, paired with the ability to spot fraud signals humans may miss through clustering, results in higher accuracy.
Secondly, AI fraud detection works in real-time. As soon as factors fall outside normal parameters, the model recognizes it and alerts relevant stakeholders. That kind of speed is difficult — at times impossible — without automation, but it is crucial in avoiding mistakes in time-sensitive operations.
Similarly, automating fraud detection reduces the workload on investment firms’ staff. Unsupervised models take this benefit further by requiring less involvement during training. As a result, firms can focus their human efforts elsewhere to maintain productivity without sacrificing due diligence.
These benefits are particularly valuable when dealing with discretionary clients. Asset managers can execute trades without clearance for these clients, which improves efficiency but opens the door to costly mistakes. Automating fraud detection ensures investment experts can serve these clients safely and quickly, as the AI will handle fraud assessments to prevent human error.
How to Implement AI Fraud Detection Effectively
Investment firms should consider a few implementation best practices to use AI fraud detection to its full potential. Optimizing these models starts with algorithm selection. Clustering may yield more real-world reliability than structured methods but may be more prone to false positives, especially in K-means clustering.
The optimal model type depends on the organization’s data availability, machine learning experience and real-world expertise in fraud detection. Firms that opt for a supervised method should prefer random forests over decision trees or regression models to minimize overfitting.
All AI fraud detection models have a high risk of false positives, with rates as high as 30% to 70% in some cases. The first step to preventing these cases is to apply contextual filters to the model’s decision-making process. The more conditions each rule has, the less likely the algorithm is to produce false positives. Ongoing tuning is also necessary.
Finally, investment firms must take great caution in protecting their AI model’s data. That includes restricting access to the model and its databases to only those who need it for their jobs. Continuous monitoring solutions are also important.
Use AI to Avoid Fraudulent Investments Today
Fraud detection efforts must improve as new technologies make it harder to distinguish between the legitimate and the fraudulent. The best way to keep up with this trend is to implement AI.
AI has already shown impressive results in fraud detection within the finance industry. Embracing this technology now will help investment firms protect their clients and their business in the future.