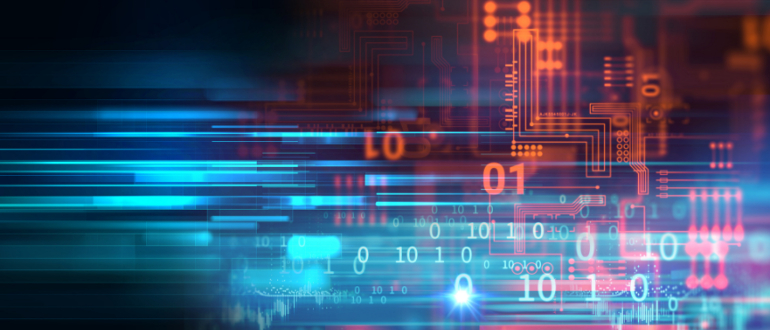
If “data is the new oil,” artificial intelligence (AI) is the new internal combustion engine. When Clive Humby coined the now-infamous phrase back in 2006, generative AI (GenAI) as we know it today was barely creating sparks. Now, discourse around AI is exploding, but it’s just another chapter in a bigger story: digital transformation.
AI’s potential is big and exciting for nearly every industry. The hype is understandable – but before “lights out and away we go” on AI, organizations need to pump the breaks. AI models are only as good as the data behind them. This data must always be available so that businesses can operate no matter what happens, be it a natural disaster, broken line of code, or cyberattack.
Hence, the right processes must be in place to ensure the data flowing in and out of AI models is not only available and accurate, but also protected and intelligent. Data resilience is the key to helping businesses run smoothly in the age of AI.
Seize Control before Shadow Sprawl Creeps in
A company’s data is ever-changing. With the extensive amount of data within an organization, it’s easier and better to manage it with training and controls early on, rather than trying to unscramble the eggs after something goes wrong.
According to McKinsey’s Global Survey on AI, 65% of respondents said that their organization already utilizes GenAI on a regular basis – that’s double from the number just 10 months ago. It’s unsurprising given all the hype AI is receiving, but that same survey found something else that should give IT and security leaders a pause: nearly half said that they are ‘heavily customizing’ or developing their own AI models.
This is what we call ‘shadow IT.’ Shadow IT refers to the unsanctioned, or unknown use of software or systems across an organization. Keeping track of all the tools – whether sanctioned or not – that teams and business units across an organization utilize is already a challenge, especially for large organizations. Building or adapting large language models (LLMs) will make it much harder for IT to manage and track the movement of data and the level of risk.
Having complete control over this is an impossible feat, but it’s still important to put processes and training in place around data privacy, data stewardship and IP. At the very least, these measures can make the company much more defendable if and when something does go wrong.
Risk Management
AI is a valuable tool that organizations can get enormous value out of when used ethically and securely. It’s not about being the progress police – it’s about understanding and managing the risk of said progress. As AI becomes a more integral part of the tech stack, it’s essential the tools fall within the organization’s already established data governance and protection principles. When it comes to AI tools, organizations need to focus on mitigating the operational risk of the data within them.
Generally, there are three main risk factors when it comes to AI:
- Security: What if an outside party can access or steal the data?
- Availability: What if we lose access to the data, even for a short period of time?
- Accuracy: What if the data we’re basing this on is wrong?
When we outline these risks, we can see that data resiliency is crucial. As AI penetrates organizations further, ensuring visibility, governance, and protection across the entire ‘data landscape’ is paramount. I like to think of the relatively old-school CIA triad of confidentiality, integrity, and availability. Organizations need to secure all three when it comes to data. As more employees turn to AI models, more gaps will appear. LLMs and other AI tools need to be covered by data resiliency guidelines.
It’s important to understand business-critical data and where it lives. Good data governance and resilience may be achievable now, but if the right training isn’t in place during the age of AI, use of these emerging tools could cause issues. Worse yet, most organizations may not even know about them until it’s too late.
Building (and Maintaining) Data Resilience
The entire organization needs to be responsible for ensuring data resiliency. With the speed at which AI is evolving, this can’t be a one-and-done, set-it-and-forget task. Data resilience covers identity management, device and network security, and data protection principles like backup and recovery. It’s not just about protection, it’s a massive de-risking project. Visibility and senior buy-in are a must. Data resilience must start in the boardroom. Without the executive understanding and buy-in, projects fall flat, and funding constraints can limit how much can be done. The “not my problem” attitude that has long-plagued organizations can’t fly anymore.
Ensuring the data resiliency of AI models can feel daunting, but it shouldn’t stop organizations from starting. In the end, doing something now is infinitely better than doing nothing and suffering the consequences later. It’s easier to start now, rather than waiting a year when AI tools are more mature and more LLMs have sprung up across the organization. Don’t fall into the trap many organizations did with cloud migration all those years ago – going all-in on new tech with little forward-thinking planning.
Test resiliency through practice drills, and plan for realistic worst-case scenarios. Have a back-up plan and have a back-up plan for that back-up plan and so on. It doesn’t matter how organizations plan – the most important thing is to start.