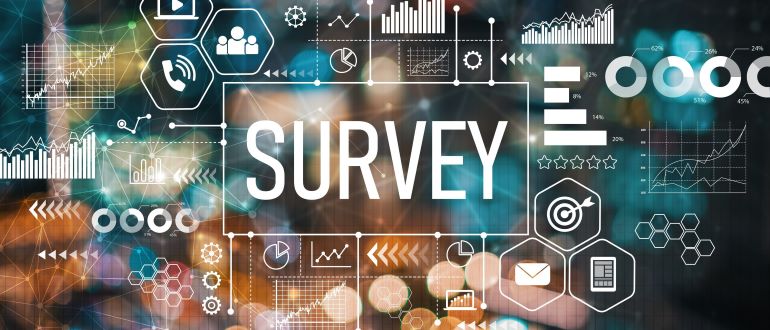
A survey of 220 IT and business professionals working for organizations in North America with more than 1,000 employees finds ensuring data readiness and quality for artificial intelligence (AI) ranks fourth (34%) behind improving data quality (42%), security (40%) and analytics (40%) as a top driver of data governance initiatives.
Conducted by Enterprise Strategy Group (ESG) on behalf of Quest Software, the survey also identifies the top three data management challenges organizations are encountering as understanding the quality of source data (38%), finding, identifying and harvesting data assets (33%) and evolving data governance for the needs of the AI era.
Specific AI challenges include ensuring regulatory compliance (40%), ensuring data quality remains high (38%) and the readiness of data for AI models (35%). Technical challenges include governing AI models and supporting data (31%), metadata harvesting and classification (23%), data quality monitoring (22%) and data quality remediation (22%).
The highest data intelligence priorities are ensuring robust usage of data (38%), increasing data quality (38%) and laying the foundations and governance for AI use tomorrow (34%).
The survey makes it clear organizations are increasingly viewing data as an asset that AI makes it easier for them to exploit, says Bharath Vasudevan, vice president of product management for Quest Software.
In fact, many organizations are now bringing AI initiatives under the purview of CIOs that already manage and govern the data used to drive those AI models, he adds. “They are providing the guardrails,” he says.
The survey also suggests organizations are becoming more adept at leveraging data, with 95% of respondents either planning to create or already having a self-service data marketplace. A full 84% of organizations are already delivering data products, with 86% planning to invest in data products in the next 12 to 24 months, the survey finds. A total of 61% claimed their organization has a clearly articulated data intelligence strategy in comparison to last year.
The data governance tasks identified as providing the most benefits to the business include metadata harvesting, classification and curation (31%), impact analysis (30%) and data policies and controls (28%).
A full 86% are also modeling their data, with 71% viewing data modeling as crucial or transformative for enhancing data product delivery and fostering collaboration.
Only 15% of respondents said their organization is coping with a data governance skills gap, even as the overall amount of data that needs to be managed and governed continues to rise, the survey finds.
Precisely how generative AI will be applied to business workflows will naturally vary from one organization to the next. Many IT teams are still experimenting with generative AI models that provide probabilistic rather than deterministic outputs that are consistently the same every time, notes Vasudevan. Each organization will need to determine to what degree those outputs are relevant within the context of a specific use case.
Regardless of approach, however, the quality of the outputs is only going to be as good as the data that was used to train and customize the AI model. More garbage in today is only even more garbage out. As such, the need for sound data management and governance practices has never been greater than it is now.