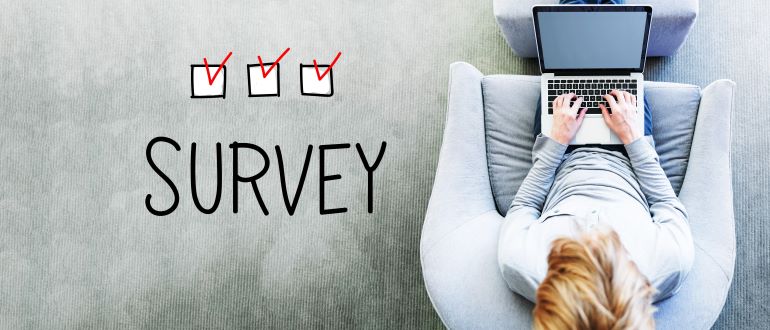
A survey of more than 276 executives finds increased efficiency and productivity (72%), increased market competitiveness (55%) and the need to drive better products and services (47%), followed distantly by increased revenue (30%) and reduced costs (24%), are the major drivers of investments in generative artificial intelligence (GenAI) applications.
Conducted by MIT Technology Review Insights on behalf of Snowflake, the survey, however, also finds only 22% of respondents believe their organization’s data foundation for building those applications is ready, compared to 53% that believe their organization is somewhat ready.
The top challenges organizations are encountering are data governance, security and privacy (59%), followed by data quality and timeliness of data (53%), costs (49%) and data silos (48%).
The survey makes it clear that more organizations are starting to realize how critical it is to have a strong data management foundation when building and deploying generative AI applications, says Prasanna Krishnan, head of collaboration and the Snowflake Horizon data catalogue. “The first step of the journey starts with a data foundation,” she says.
The challenge is that data typically has gravity in the sense that it is often challenging to move and aggregate. Organizations have historically created and stored a lot of data, but few are optimally managing much of it.
The second issue is the type of data needed to drive generative AI applications tends to be unstructured. Structured data is usually found in a database format that is relatively easy to aggregate, but most of the data needed to build or customize a large language model (LLM) is unstructured. It resides in everything from PDF files to spreadsheets that are typically stored everywhere from the cloud to a laptop. Aggregating all that data and converting it into a set of vectors that an LLM can consume is a major undertaking.
Finally, many organizations are starting to realize the use cases they want to apply generative AI to require instant responses from the LLM. That means data needs to be continuously fed to the LLM. Most IT teams today rely on batch-oriented processes to update applications. The percentage of IT teams that have the expertise required to manage near real-time data flows remains limited.
Less clear is to what degree organizations plan to bring AI models to where data already resides or attempt to unify their data using, for example, a data lake hosted in the cloud or elsewhere. Unifying data makes it simpler for organizations to democratize access across multiple business units, notes Krishnan. Eventually, generative AI will require organizations to unify multiple data sources, ideally using a cloud platform that also makes it possible to build and deploy AI models, she adds.
Depending on the use cases, most organizations are likely to pursue a combination of moving data and bringing AI models to where data already resides. The one thing that is certain is that data management challenges explain that, despite the current level of enthusiasm, many organizations are finding it will be 2025 before they can build and effectively deploy a custom GenAI application in a production environment.