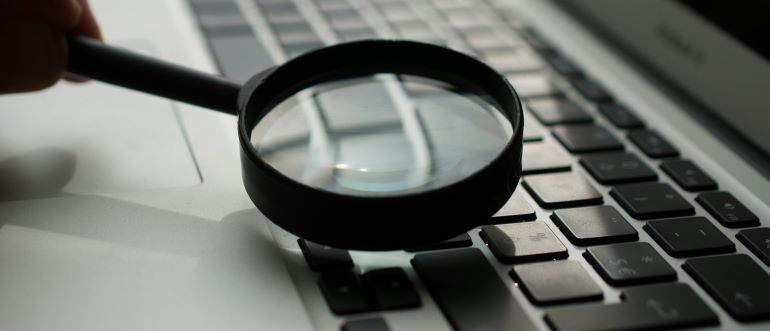
A survey of 1,367 artificial intelligence (AI) professionals published today finds 85% work for organizations that have delayed projects because of lack of access to graphical processing units (GPUs), with well over a third (39%) having delayed these projects anywhere from three to six months.
Conducted by Civo, a provider of cloud computing services, nearly all respondents (97%) cited high computational costs as a significant strain on their organization’s ability to capitalize on AI. A full 82% agreed that adequate GPU capacity is essential for maintaining long-term competitive advantage.
Unfortunately, gaining access to GPUs is likely to become even more challenging in the months ahead, says Josh Mesout, chief innovation officer for Civo.
As the next generation of GPUs become available, the servers are creating demand for energy that overwhelms the ability of existing data centers to provide. As a result, even more AI projects are likely to be delayed, says Mesout.
“People are getting ready for AI winter.”
The only way to mitigate those costs is to either rely on lower cost AI accelerators, or employ smaller AI models that require less computation resources, Mesout says.
Alternative AI accelerators, however, don’t provide the same level of processing capabilities as GPUs causing many AI teams to be reluctant to use them because of lower level of accuracy for AI outputs compared to a GPU.
The survey finds that 75% anticipate a growing need for GPU capacity over the next 12-24 months.
Alternatively, many organizations would be well advised to rely more on open-source AI models that don’t require a fee to invoke a commercial application programming interface (API), Mesout notes. Each time an input and output invokes those APIs, they increase the total cost of AI, and now many of the open-source models are able to achieve the same level of performance as a commercial large language model (LLM), he says.
Overall, the survey finds organizations are accessing GPUs from a range of data centers, including cloud-based resources (32%), hybrid cloud resources (32%) and on-premises infrastructure (14%).
The top challenges companies cited in scaling AI infrastructure are high costs associated with infrastructure (54%), limited access to skilled personnel (43%), and the high cost of inference (40%).
Most organizations as a result will need to narrow the number of AI projects they decide to fund as they move beyond experimentation, because of GPU costs. In fact, it may not be until well after 2030 before enough energy resources are available to make the next generation of GPUs widely accessible.
Ultimately, organizations are going to need to find ways to optimally consume GPU resources as efficiently as possible. That may prove challenging to achieve as more companies realize that many AI capabilities are rapidly becoming table stakes that they need to provide just to remain competitive versus actually providing a strategic advantage that justifies the cost of investment. It may not necessarily be what every business leader wants to hear, but it is rapidly becoming our new AI reality.