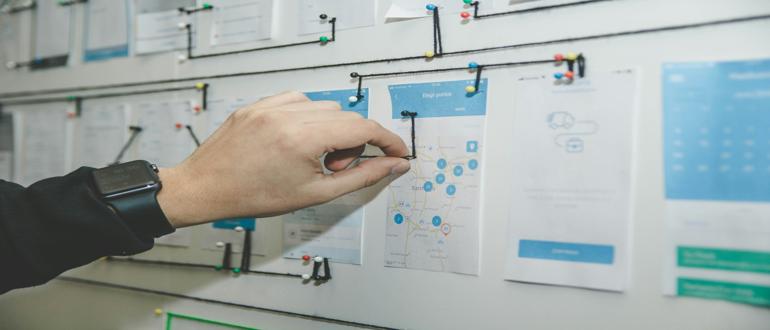
The promise of artificial intelligence (AI) is undeniable. However, the journey from concept to reality is fraught with challenges that require careful navigation.
Below are some essential steps that one may take to ensure effective implementation of AI into the enterprise.
Understanding the AI Landscape
The first step to AI implementation is understanding the broader AI ecosystem. As data becomes the lifeblood of AI, organizations must modernize their data infrastructure to support scalable and efficient AI workloads.
Traditional approaches to data management are no longer sufficient —they must evolve into intelligent data ecosystems capable of handling diverse and dynamic workloads.
Challenges such as data silos, integration issues and compliance hurdles often impede the progress. So, ensuring data quality and governance is paramount. 79% of global tech executives recognize unifying data as essential or very important for achieving top AI outcomes by 2025, underscoring the critical role of integrated data systems. Addressing these challenges upfront lays the foundation for successful AI adoption.
Building a Robust AI Strategy
AI implementation begins with clarity of purpose. Define specific, measurable objectives that align with your organizational goals. Are you looking to enhance customer experiences, streamline operations, or gain deeper insights into market trends? Identifying practical use cases provides direction and helps secure buy-in from stakeholders.
Equally important is assembling a diverse, cross-functional team. AI initiatives cannot succeed in isolation; they require collaboration between data scientists, IT professionals, business leaders, and domain experts. This team will not only drive technical execution but also ensure that the AI strategy aligns with business priorities.
A cohesive data strategy is another cornerstone. Treat data as a strategic asset by investing in robust governance frameworks and modern architectures. Scalable and secure data infrastructures enable organizations to support demanding AI applications effectively. According to the 2024 Data Complexity Report, approximately 65% of companies worldwide report that their data is either fully or mostly optimized for AI, indicating significant progress but also highlighting room for improvement.
Implementing AI: From Concept to Reality
The transition from conception to execution involves several key steps:
- Data Preparation and Integration: Data is the backbone of AI. So organizations must take extra care to collect, cleanse, and integrate data from various sources. This will create a unified dataset that AI models can effectively utilize.
- Infrastructure Readiness: Deploying AI requires high-performance infrastructure. Enterprises should optimize their storage and compute resources with AI workloads in mind, ensuring seamless scalability and operational efficiency.
- Model Development and Training: Developing AI models involves selecting the right algorithms and training them with high-quality data. Regular iteration and refinement are critical to achieving accurate and reliable outcomes.
- Testing and Validation: Before deployment, models must undergo rigorous testing to ensure they perform as intended under real-world conditions. This step minimizes the risk of errors and biases.
- Deployment and Integration: Once validated, AI models need to be integrated into existing workflows. This step often requires organizational change management to ensure seamless adoption.
Ensuring Security and Compliance
AI systems rely on vast amounts of data which raises significant security and privacy concerns. Organizations must implement robust data governance practices to protect sensitive information and comply with regulations.
Additionally, cyber resilience should be made a top priority. AI-driven solutions can play a key role in safeguarding critical infrastructure against emerging threats.
Scaling and Continuous Improvement
AI implementation is not a one-time effort—it is an ongoing journey. Monitoring the performance of AI systems is critical to identifying areas for improvement. Regularly revisiting and refining AI models ensures they remain aligned with evolving business needs and technological advancements.
Organizations must also remain agile, ready to adapt to new opportunities and challenges in the AI landscape. This might involve incorporating cutting-edge innovations or re-evaluating strategies to stay competitive.
Charting Your AI Journey
The journey to AI implementation requires more than technological investments; it demands strategic vision, organizational alignment, and a commitment to continuous improvement. By following a structured roadmap—from defining objectives and building a robust strategy to ensuring security effectively—organizations can unlock the transformative potential of AI.
And in the end, it is essential to remember that success lies not just in adopting AI but in embedding it into the fabric of the enterprise such that it can drive meaningful outcomes.