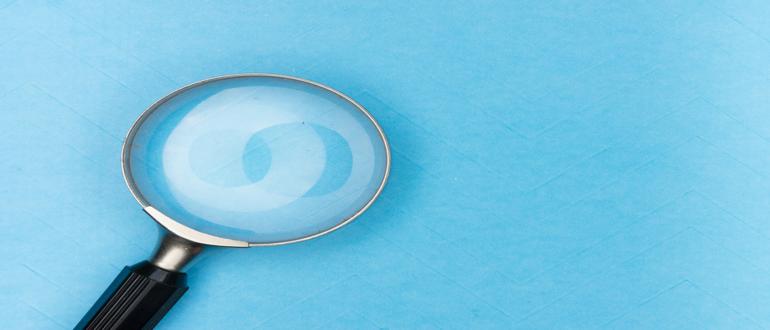
Imagine a search that learns. Not generally, not in the abstract—but about the world you work in; the information that drives your business; and the knowledge that allows you to address both the challenges and the opportunities. This is the promise of generative artificial intelligence (AI) and search.
Let’s explore what this could mean for business employees and customers, and their approach to adopting AI — especially tools capable of learning and delivering better user experience.
Generative AI Investments and Use Cases
AI, including the generative AI tools we use today, is on track to receive another $656 billion in 2025—on top of the trillion-plus dollars invested in it since 2020. Additionally, it is expected to deliver $6.1 trillion to $7.9 trillion of value annually by 2030, even with the 80% of AI projects that “fail.”
AI don’t fail in the traditional sense. We are still in the experimental era, and much like Edison discussing the development of the light bulb when he made his legendary quip, “I have not failed. I’ve just found 10,000 ways that won’t work,” businesses and organizations are still discovering the myriad ways AI doesn’t work to prove the ones that do. Only instead of one light bulb, we end up with countless innovative AI applications in those 20% of projects that do work.
According to new data from Ernst & Young LLP, 97% of senior business leaders whose organizations are investing in AI report positive ROI from their AI investments.
To realize the full benefits, investment decisions must be grounded in clear understanding of the technology and the challenges that organizations must overcome. This means asking the right questions, like, “What architectural and design choices do we make to ensure the AI we deploy not only gives us the answer but that the answer is correct, trustworthy, cost-effective and scalable?”
We know generative AI has its downsides. It hallucinates, makes mistakes, confidently invents incorrect answers that erode trust, and mistakenly attributes a greater level of fault towards the technology. Retrieval Augmented Generation (RAG) offers a proven architecture for delivering generative AI results for the most demanding of use cases with better levels of accuracy.
A more advanced RAG approach involves adding human intelligence to the data and its knowledge models so that the data used always has an expert lens applied. Not only does this help mitigate the challenge around the trustworthiness of results, but it also offers users a new way to engage with and discover their data.
One of the key features of this architecture is the ability to provide subject matter experts with a look into the data and how users search for it. After extracting the concepts found in the user’s query, the SME can compare those concepts to the knowledge graph of their data. If it doesn’t exist in the knowledge graph but should, the SME can add it. Using a reverse query function, the SME could then inform the user that their query can now be answered. This provides a knowledge graph that learns more as users use it and gives the statically trained AI this new data. The approach provides organizations and knowledge workers a search experience that improves, learns and adapts as it’s used—an applicable knowledge discovery experience tailored to the user, answering questions they might even forget they asked.
This is just one way of architecting an AI solution that combines the latest form of RAG-enhanced generative AI, knowledge graphs and human expertise. Some are calling this neuro-symbolic AI. Integrating human intelligence and expert knowledge into AI ensures that the system continuously evolves and adapts to new information, offering users a dynamic and intuitive search experience. As a result, businesses can leverage this advanced technology to deliver more personalized and insightful interactions, ultimately driving greater engagement and satisfaction among users.