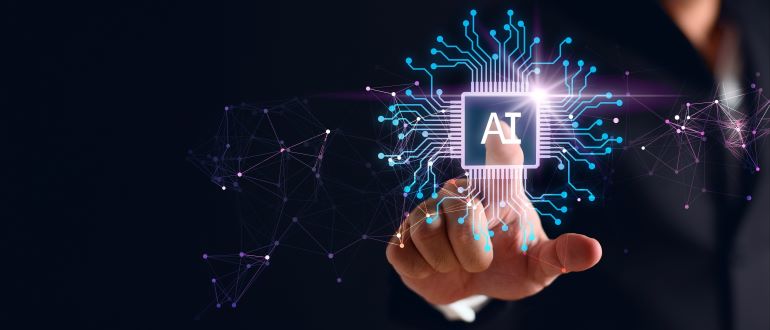
Protect AI today published a report identifying 32 vulnerabilities in the Triton Inference Server from NVIDIA and the Intel Neural Compressor that are both widely used to build and deploy artificial intelligence (AI) applications.
Discovered via a bug bounty research initiative that Protect AI invited more than 15,000 cybersecurity researchers to participate in, the vulnerabilities range from the fairly common, such as a SQL Injection attack, to flaws that allow unauthorized users to delete entire data sets, that primarily exist in the tools used to build these platforms.
Each vulnerability discovered was reported to the maintainers of the tools where the vulnerability originated 45 days prior to disclosure. However, in some cases the maintainers of the open source software that was used by the developer of Triton Inference Server and the Intel Neural Compressor platform did not respond, says Dan McInerney, lead AI Threat Researcher for Protect AI.
Many of the developers and data science teams building AI platforms and models have little to no cybersecurity expertise. All too often, they are simply unaware that the tools used to build AI platforms and artifacts have known vulnerabilities that cybercriminals already know how to exploit. In fact, cybercriminals have already launched campaigns to exploit known vulnerabilities in AI models, notes McInerney.
The issue when it comes to AI applications is there is a lot more at risk than a vulnerability that might be discovered in another application built using those same tools, he added. In theory, organizations might be able to remediate those vulnerabilities themselves, but unless they are accepted by the maintainer of that project, they will find themselves having to support a fork of that original project.
It’s not clear who is assuming responsibility for AI application security, but it generally falls to data science teams to apply any fixes made available by upgrading their tools and platforms within the context of a set of best security practices that should be added to machine learning operations (MLOps), says McInerney. “They need to embrace MLSecOps,” he says.
The challenge is that when a vulnerability is discovered in the tools and platform used by an MLOps team, they might also need to update the AI models that were constructed using the previous versions of those tools and platforms. In many cases, those AI models will need to be retrained because, unlike other software artifacts, an AI model doesn’t lend itself easily to applying a patch to remediate a vulnerability.
In general, vulnerabilities, when unaddressed, conspire to increase the overall cost of deploying applications because cybersecurity teams are then required to invest in zero-trust platforms that, hopefully, ensure only individuals with the right privileges are granted access to those applications, says McInerney. As a result, the overall cost of securely building and deploying AI applications tends to increase over time, he notes.
It’s not likely organizations will be able to avoid investing in those platforms simply because they embraced best MLSecOp practices, but the number of times they need to rely on them as a last line of defense could be substantially reduced if the AI applications themselves are a lot more secure than most of them are today.