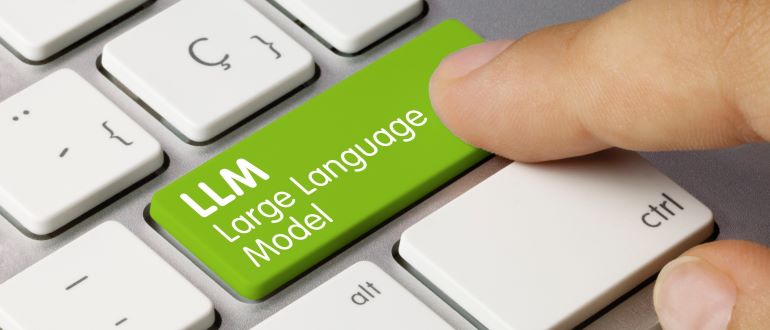
The newly released Falcon 180B, an open large language model (LLM) from the United Arab Emirates-based Technology Innovation Institute (TII), part of the Abu Dhabi government’s Advanced Technology Research Council, shows competitors will likely continue for some time to rival LLMs released by Anthropic, Google, Meta, OpenAI and others.
“There will always be a race to larger models,” says Mike Gualtieri, VP and principal analyst at Forrester Research. “For enterprises, this means models will cover more topics, be more accurate, and articulate.”
However, the value of those gains doesn’t extend to all use cases, notes Gualtieri, who says such gains are most useful when using the LLM for general AI prompts, and such power does come with trade-offs. “A model that big won’t help for more domain-focused use cases such as health care and law where you don’t need a model trained on Shakespeare’s sonnets or AC/DC lyrics. The larger the model, the more costly to inference and [the bigger] increase in latency,” he says.
Falcon 180B is trained in 180 billion parameters on 3.5 trillion tokens and claims to have four times the compute resources of Meta’s LLaMA 2 and is comparable to Google’s Bard while just behind OpenAI’s ChatGPT 4.0. Falcon 180B sits at the top of Hugging Face’s leaderboard for open-access LLMs. Falcon 180B is open access for researchers and commercial users with many restrictions within its license.
While TII hopes Falcon 180 B’s open-access model will make huge LLMs widely available, others question whether the improvements it brings are worth the added expense of powering such a large model. “We envision a future where the transformative power of AI is within everyone’s reach. We are committed to democratizing access to advanced AI, as our privacy and the potential impact of AI on humanity should not be controlled by a select few,” said H.E. Faisal Al Bannai, secretary general of the Advanced Technology Research Council, in a statement.
The LLM race and open vs. closed models raise interesting questions, as does when the models’ sizes become a challenge for many enterprises.
“The main issue with Falcon 180B is the marginal improvement over LLAMA2 and Falcon 40B,” says developer David Barton, CEO at OneIT, based in Perth, Australia. “It doesn’t quite justify the significantly increased hardware cost. If Falcon 180B is more amendable to future fine-tuning, that could make it worth the cost in certain use cases. Out of the box the performance improvement is so marginal that it’s hard to justify the tripling of running costs,” Barton adds.
In Barton’s experience, most enterprises repeatedly run specific use cases rather than solving ad-hoc reasoning or knowledge problems. “Examples like Orca and Phi-1 indicate that smaller models can perform excellently in that niche with good training data, and fine-tuning is a reasonable substitute. I think more emphasis needs to be on fine-tuning and better training rather than increasing parameters,” he says.
Forrester’s Gualtieri explains that there are ways to mitigate expenses associated with the model size. “Numerous techniques can be applied when analyzing a prompt to execute only against parts of a model that are relevant. Training costs will go up, but the value of the model will increase too,” he says.
Gualtieri also sees the proliferation of powerful LLMs as good news for those who seek choice. “There will be tens of thousands of LLMs focusing on domains and possessing different personalities. Open source communities such as Hugging Face will allow millions of developers around the world to experiment and use open source models, including using them directly for fine-tuning and prompt tuning,” he says.