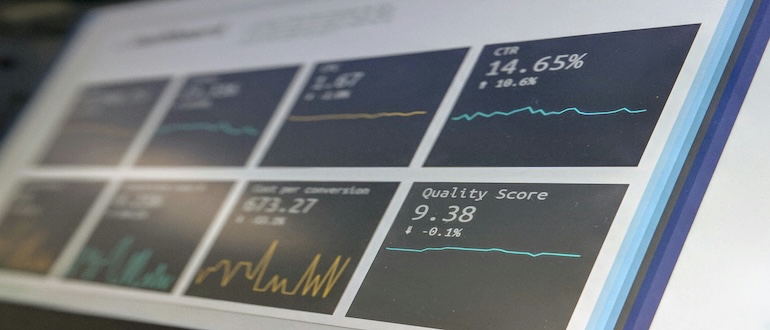
As artificial intelligence (AI) models grow more powerful, enterprises increasingly find their storage solutions unable to handle the data load – or bring data fast enough to the processors.
In 2022, Meta reported its infrastructure growth trends from the previous two years. According to the data, Meta’s AI infrastructure requirements had grown in leaps and bounds – principally driven by increases in the scale of data used to train its AI models. The growth, the report stated, was between 1.75 to 2x. Side by side, Meta’s data ingestion throughput requirement too had jumped up 3 to 4 times.
The MLPerf Storage benchmark, David Kanter, executive director of MLCommons, a non-profit AI safety work group that tests AI systems-under-tests, said, was born out of this trend.
“Storage is truly critical to big data models,” he emphasized.
But not enough people know about the outsize role storage systems play in the context of AI. As a result, companies despite willingly plowing money on specialized processors, often end up getting less-than-desired mileage out of them because the access to data is not fast enough.
At the AI Field Day event in Silicon Valley, January, Curtis Anderson, co-chair of MLCommons Storage Working Group, dove into the recently released MLPerf Storage benchmark v1.0 to explain why it’s critical for companies to look at the system results in comparison to their scale and speed requirements before getting into AI infrastructure investments.
“There’s an enormous amount of data and enormous number of accelerators and that means an enormous amount of bandwidth and capacity to manage for the storage system,” emphasized Anderson.
The reason data transfer speeds are critical in AI is because of something called random access pattern. AI models learn and work by deriving information from sources in a seemingly arbitrary order, almost like going through pages in a book.
“You can’t show all of your cat pictures and then all of your dog pictures because the neural network won’t learn that way. You have to intersperse them randomly,” said Anderson of model training while presenting the benchmark results.
“You go through one epic feeding the data through and then the next epic has to be a different pattern and so that defeats all of the normal caching techniques that storage systems tend to use,” he added.
A major disadvantage with traditional storage systems is that they were never built to support this level of randomization. “We never designed storage systems to be able to have twice as much I/O per unit volume as they were designed. We’ve never designed them to be able to handle this kind of randomization or parallel access,” said Stephen Foskett, president of Tech Field Day, a Futurum Group unit.
The version 1.0 benchmark which had 14 submitters including HPE, DDN, WEKA, Hammerspace and Nutanix and 130 submissions, digs into how efficiently the storage systems can support this high-speed and high-scale storage needs of AI workloads.
Using three kinds of workloads – 3D U-Net, ResNet-50 and CosmoFlow – that each stresses the systems differently – on emulated accelerators and with the end goal of keeping the GPUs 95% utilized, the benchmark offers verdicts on system performance for each solution.
The scores give customers a way to compare products across vendors based on real numbers.
“The thing we were trying to demonstrate is that storage is not going to slow down the computer…So if you have excess latency on some I/O operations that counts against you, too many of those and you don’t have a valid submission any longer. Keeping the thing busy is the core metric. Can you keep up with the demand?” said Anderson.
Users can make cross-system comparisons on the benchmark’s two key run divisions – Closed which MLCommons calls “apples-to-apples” comparison, and Open that offers certain tuning and optimization flexibilities.
The Closed results are a measure of how various systems fare with hitting the performance baseline with virtually identical workloads. Alternatively, the Open results show how the system configuration can be tuned to enhance that performance.
AI continues to push storage vendors to do better. But will it take them to step out of their comfort zone once and for all to push the envelope and endow storage systems with speeds and capacity sufficient to support cutting-edge AI workloads? Anderson thinks the movement is well underway.
“Storage is a slow-moving business. In terms of architectures, the quality requirements are so high [because] if you lose data, you’re toast. People don’t take large risks unless they need to and so that’s what happening with AI. The industry is being forced to take larger risks, do more innovation and increase the speed of innovation in order to meet the demand,” he said.