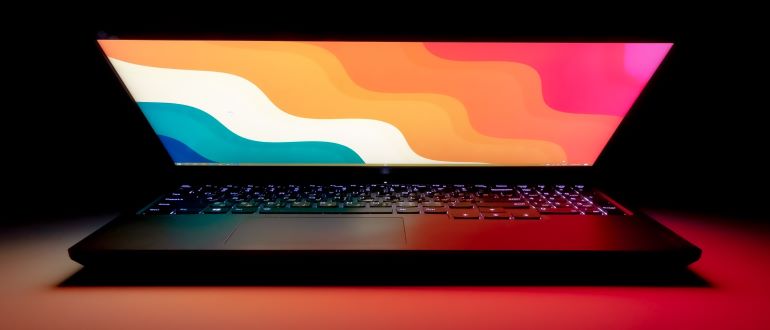
Lenovo today at its annual TechWorld conference previewed an ability to compress artificial intelligence (AI) models in a way that promises to make it feasible to securely run them either on a PC or local server.
Dubbed AI NOW, this capability promises to enable either individual end users or an organization to run personalized generative and private inferencing models based on local data in a way that preserves security and privacy, says Lenovo CTO Yong Rui. “It’s a hybrid framework,” adds Rui.
The Personal and Enterprise AI Twin frameworks ensure data that has been exposed to an AI model does not wind up being inadvertently used to train an AI model that is publicly accessible.
At the same time, Lenovo is expanding its alliance with NVIDIA to make available the Lenovo ThinkSystem SR675 V3 server and ThinkStation PX workstation, which make use of NVIDIA processors to run NVIDIA AI Enterprise software. The ThinkStation PX enables up to 4x NVIDIA RTX 6000 Ada graphical processor units to be configured in a workstation.
Additionally, Lenovo and NVIDIA have committed to build systems based on the NVIDIA MGX modular reference design along with VMware Private AI Foundation with NVIDIA, a framework for deploying NVIDIA software to build generative AI models on virtual machines from VMware.
Lenovo also touted its commitment to DRIVE Thor, an automotive-grade system-on-a-chip (SoC) based on CPU and GPU from NVIDIA capable of processing 2,000 teraflops of performance that will enable AI models to be deployed in vehicles as early as 2025.
Interest in customizing LLMs by exposing them to unstructured data residing in some type of vector database is rising sharply. Not many organizations have the data science expertise required to build, deploy and secure LLMs but they can customize them using frameworks such as Langchain. The challenge is making sure none of the data they expose to an LLM inadvertently makes its way into an AI model that is publicly accessible.
Lenovo, like most other providers of IT infrastructure in that rush to customize LLMs, will see a massive increase in spending on IT infrastructure to run databases and frameworks needed to train AI models.
It’s not known at what rate the customization of LLMs for various use cases is occurring but there is no doubt most organizations are experimenting with them. The challenge is in addition to requiring access to massive amounts of data residing both in the cloud and on-premises IT environments, organizations will also need to revisit how IT teams are structured. As AI models become more pervasively deployed, data engineering, machine learning operations (MLOps), DevOps and security operations (SecOps) are all going to increasingly converge.
The challenge will be to reduce that friction as much as possible as the rise of AI finally forces IT organizations to confront an issue that has historically conspired to increase both the cost and complexity of managing IT at the level of scale that will be routinely required by AI applications.