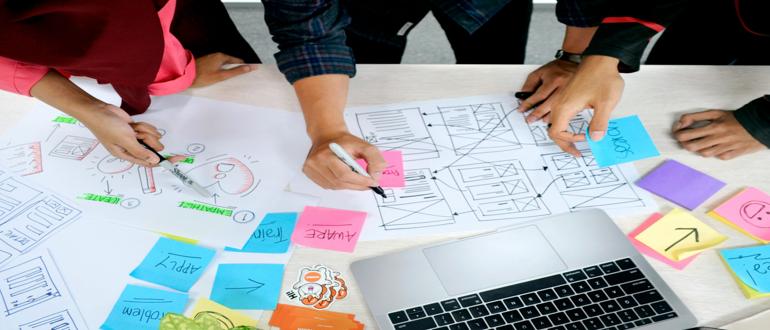
Generative artificial intelligence (AI) has seen significant growth over the past few years. What began as questions and answers have evolved into intelligent processes capable of providing deep information that enriches both engineering and design.
These benefits aren’t just theoretical. According to recent research, 58% of companies say that GenAI has prompted innovation, enhanced customer relationships and increased revenue.
But where exactly does GenAI fit in the design and engineering process? What practical benefits does implementation offer, and how do efforts translate into real-life applications? Let’s find out.
Democratizing the Design Process
The primary driver of GenAI adoption is ease of use. Designers and engineers can pose questions in plain language and get answers. This makes it possible to quickly and easily iterate new design ideas.
Historically, designers and engineers had to rely on complex and highly technical software that required in-depth training. This software was often resource-intensive, meaning it required significant computing power to deliver results.
Using available data, GenAI tools can produce output similar to those of highly technical tools, but without the need for high-level software knowledge and often in a significantly shorter time, thus democratizing the design process.
Benefits of GenAI Implementation in Design and Engineering
Implementing GenAI in design and engineering offers multiple advantages, including:
Task Automation
While generative AI can’t “think” like human beings, it can break larger tasks down into smaller steps and then execute those steps in logical sequences. This makes it ideal for task automation, especially in the fields of engineering and design.
Consider an auto manufacturer looking to reduce drag and improve performance on its vehicles. Traditional design technologies allow users to test the impact of specific factors on designs, but require significant human interaction, effectively creating a manual process within a digital one.
Generative AI, meanwhile, can carry out tests autonomously within a set of given parameters. Engineers and designers can provide a specific prompt, describe their expectations, and then let AI do the work. Once complete, staff can review the results and determine if further work is required.
Design Optimization
There’s no such thing as a “perfect” design. Designs evolve as new processes, materials and strategic goals are identified.
Optimization over time helps ensure that specifications meet business needs. GenAI streamlines this process.; as goals and metrics change, engineers and designers can update AI models to evaluate current performance against ideal results, and then use GenAI to suggest design changes that could help achieve these goals.
Development Acceleration
Finally, GenAI helps accelerate the development process. One example is safety. For automotive manufacturers or industrial design firms, user safety is both a reputational and regulatory issue. It takes a considerable amount of time to ensure new products or components meet (or exceed) safety standards.
Using generative AI with access to all applicable safety standards and materials specifications, enterprises can prioritize safety without sacrificing speed, allowing them to focus on the big picture while AI handles the details.
Exploring the Process
What does the GenAI design and engineering process look like in practice?
It starts with an idea. Engineers or designers ask AI to create a new product design based on a new concept or one that improves on an existing design. Here, data makes the difference. The more data GenAI can access and the more detailed this data is, the better.
Consider an engineer looking to enhance the performance of high-volume industrial machinery by improving its design. By providing GenAI access to key data, including current machine specifications and performance data along with more granular data such as temperature gradients and liquid circulation measured by clamp-on ultrasonic flow meters, engineers can specifically address and resolve the limitations of current designs.
Once GenAI has produced an initial design, it can be used to evaluate this design against different criteria. For example, engineers could ask AI to simulate a typical workload for the device and see how it performs. They could also ask AI to extrapolate what would happen if the new design was exposed to sudden changes in temperature, pressure, or other conditions.
Generative AI is also useful in post-production to help evaluate the performance of new machinery against baseline expectations, especially since real-world applications often differ from theoretical frameworks. Using this operational data, GenAI can help engineers and designers further refine and improve outputs.
The first generation of AI tools helped optimize design. Generative AI takes this process a step further. By using a combination of historical data, information collected by IoT devices, and data-driven trends prediction, GenAI can help designers and engineers innovate on existing frameworks to optimize design, improve performance and accelerate development.