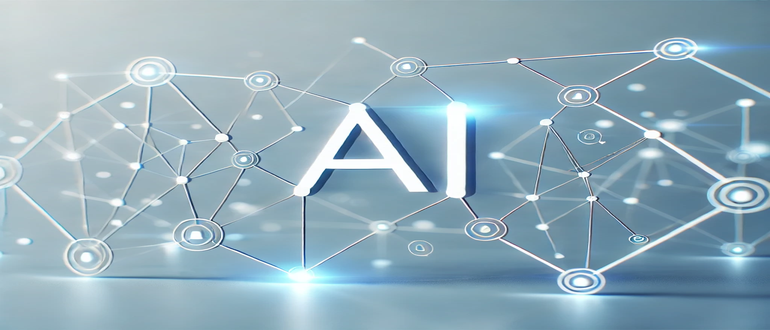
Businesses are increasingly turning to artificial intelligence (AI) as a transformative force. While some have already implemented it aggressively, others are still exploring ways to leverage its potential. However, nearly all recognize that AI will be pivotal in driving future successes.
When adopting the technology, companies often assess their readiness in terms of business strategy, safety, skills and product capabilities—all critical areas to address. Yet, one vital aspect is frequently overlooked: the network infrastructure.
AI adoption places significant new demands on enterprise networks. Without proper accommodations, AI workloads risk being slow, inefficient and costly, while exposing organizations to serious security threats. As a result, their substantial investments in AI may fail to deliver the anticipated return on investment.
To ensure their network is AI-ready, businesses must ask these three questions.
Does the Network Have Bandwidth and Other Resources to Support AI Demands?
Organizations must first ensure their networks provide the necessary performance to support AI workloads. Unlike traditional applications, AI demands significantly more resources to function effectively. These applications process vast amounts of data across long distances, often with the need for speed to enable rapid decision-making. Achieving this requires adequate network bandwidth. Without it, AI applications may experience delays and interruptions.
Additionally, AI workloads can monopolize bandwidth, potentially disrupting traditional, mission-critical applications that rely on the same underlying infrastructure. Ensuring balance is critical to maintaining smooth operations across the board.
Organizations that are unprepared from a network performance level will find themselves desperate for a solution. For example, there are enterprises shipping disc storage via FedEx and UPS to their data centers across the country to help support AI data transfer demands. Of course, this is a very cumbersome and cost-ineffective approach that’s impossible to do at scale.
Are They Prepared to Mitigate AI’s Network Security Vulnerabilities?
AI introduces numerous attack surfaces across the network, exposing organizations to new and evolving risks. These vulnerabilities grow as data travels across diverse environments like multi-cloud infrastructures, adding complexity to security efforts. Organizations adopting AI must ensure their networks are safeguarded against these emerging threats.
If your security and networking teams operate in silos and rely on a patchwork of point solutions, defending against these sophisticated attacks will be nearly impossible. Such an approach may only suffice for traditional networks, but for the AI era, it is too slow and fragmented. Consider a scenario where a malicious actor injects poisoned data into AI models designed to optimize network performance. By the time the networking team identifies the issue and collaborates with the security team to address it, the damage could already be irreversible.
Do They Have Clear Visibility Into Their AI Ecosystem?
Lastly, it’s essential that organizations establish robust network visibility across the AI ecosystem, which encompasses large language models (LLMs), generative AI applications and the supporting infrastructure. This visibility is closely tied to security: Without clear insights into AI workloads, enforcing effective security measures becomes nearly impossible.
AI relies heavily on data, making it essential to identify data sources and protect them against adversarial attacks. For example, consider prompt injections targeting AI-driven network monitoring software. In such an attack, adversaries bypass AI guardrails to inject unnecessary or falsified traffic into the network. This manipulation tricks the monitoring model into overlooking anomalies, producing inaccurate predictions and leaving the network vulnerable. With comprehensive visibility, organizations can detect and thwart prompt injections and other attempts before significant damage occurs.
Performance also benefits from enhanced visibility. By gaining a clear view of network activity, companies can identify bottlenecks in their AI workflows, conduct root cause analyses and address issues efficiently to optimize operations.
Meet the Network Demands and AI Will Pay Off Big
AI adoption has the potential to unlock unprecedented efficiencies, insights and opportunities for enterprises. However, to fully harness these benefits, organizations must first address the critical networking challenges. This requires the trifecta of enhancing performance, strengthening security and ensuring robust observability within the infrastructure.