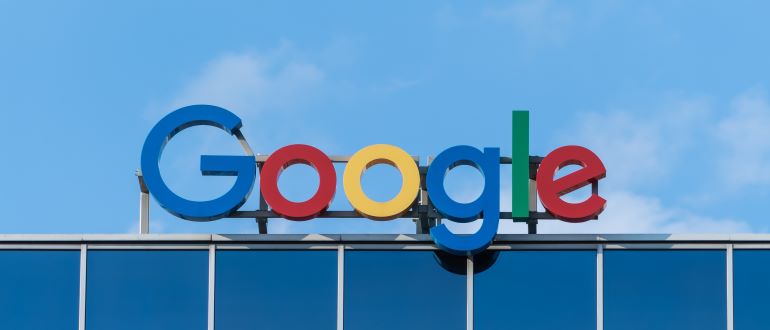
As more organizations look to operationalize generative artificial intelligence (AI), it’s become clear that many of them will need to revisit their core database strategy as they simultaneously adopt frameworks such as LangChain to expose data to large language models (LLMs). Those changing requirements are, not surprisingly, setting off a feeding frenzy among providers of database platforms.
Google, for example, this week announced it has generally made available AlloyDB AI, an extension of its Postgres database platform that makes it possible to convert data into a vector format that can then be used to expose an LLM to data beyond what it was initially trained on.
At the same time, Google is making available a public preview of vector search capabilities that have been added to its implementations of Spanner, MySQL and Redis databases.
In addition, Google is integrating its BigQuery data warehouse with Vertex AI, a machine learning operations (MLOps) platform for managing the building of AI models, to make it simple to expose text and speech to AI models. Google is also making Gemini 1.0 Pro accessible for BigQuery customers via Vertex AI.
Finally, Google is adding integrations with LangChain, a framework for developing applications powered by language models.
The overall goal is to make it simpler for IT teams to drive use cases involving analytics such as semantic search, similarity detection and retrieval-augmented generation (RAG) using data stored in multiple types of databases, says Brad Calder, general manager and vice president of engineering for Google Cloud. “Data is crucial for AI,” he notes.
It’s not clear to what degree organizations are prepared to rip and replace their existing database management platforms to operationalize AI. Many of their existing operations depend on platforms that have been in place for years and the cost of replacing them could easily be prohibitive.
On the other hand, numerous silos have emerged over the years, so AI may present IT teams with an opportunity to consolidate platforms in a way that makes it simpler to manage data at scale. Those efforts should also help reduce the total cost of IT by reducing licensing costs and, potentially, the size of the IT staff needed to manage their data.
One way or another, organizations will have to revisit the way they manage data at scale in the age of AI. Less clear is how data management teams typically led by data engineers will collaborate with the data science and DevOps teams that build and ultimately deploy AI models. Each organization will need to determine how best to structure teams that have widely divergent cultures as they seek to operationalize AI in the coming year.
In the meantime, IT teams will need to explain why investments in data management will need to be made to realize the promise of AI. That conversation may be a little uncomfortable, however, because many of those business executives tend to assume that those investments have already been made, given the amount of investment that has previously been made in IT.