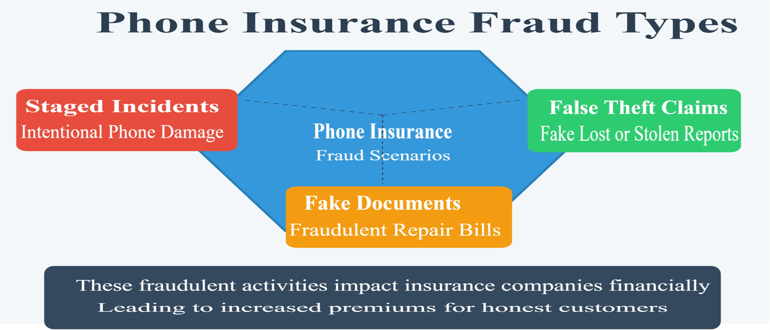
Phone insurance fraud is a growing concern among insurers worldwide. Every year, fraudulent insurance claims amount to staggering financial losses for providers, and increased premiums for customers.
Experts claim that up to 40% of device insurance claims are fraudulent. These include staged damage, false theft reports, and counterfeit devices which are extremely difficult to spot using traditional fraud detection methods. And due to high volume and complexity of theses claims, insurers struggle to detect and prevent them manually.
Addressing these challenges, artificial intelligence (AI) is emerging as a powerful tool to identify and mitigate fraud effectively.
How AI Can Tackle Phone Insurance Fraud
AI and machine learning (ML) systems can crunch through massive sets of data and find fraud trends from them. It can even detect and prevent fraud in real-time, leveraging machine learning (ML), natural language processing (NLP), and computer vision.
Here are some areas where AI can prove beneficial for fraud detection.
1. Pattern Recognition
If trained with historical claims data, AI can reliably detect suspicious patterns . For example, it can identify unusually frequent claims from the same individual, or claims filed from a geographical area different from where the policy was initially enrolled, all of which may indicate potential fraud.
2. Image and Video Analysis
Using computer vision, AI can examine photos and videos to find if they are doctored. It can identify unnatural lighting, mismatched shadows, edits, timestamps mismatch, and so on.
4. Behavioral Analytics
AI leverages behavioral analytics to monitor policyholders’ activities. So, sudden changes in location that are inconsistent with the policyholder’s normal behavior. or repeated login attempts from unfamiliar or suspicious IP addresses can be caught and flagged in real-time.Â
Using AI in Fraud Detection
AI offers significant advantages in insurance fraud detection because of its ability to improve accuracy and eliminate human errors. It is helpful for processing genuine claims while flagging suspicious ones. This can save insurers money by preventing fraudulent payouts and cutting down on the administrative costs associated with handling false claims. The other advantage is preventing unnecessary delays.Â
Challenges and Ethical Considerations
While AI offers immense potential, it is not without challenges:
- Low data quality:Â The quality of data strongly affects AI and the accuracy of machine learning algorithms.
- Bias in AI Models: Model bias can lead to discrimination against specific groups.
- Transparency: Explaining AI decisions to customers and regulators can be quite a complex thing.
Additionally, it can generate false positives or negatives which can lead to errors. Such errors are in fact quite common because the training data used for AI algorithms is rarely 100% accurate or complete, which leaves ample room for mistakes.
Another issue is that actual data may not be used in training the algorithms owing to privacy concerns Instead, dummy data based on real data is fed in, leading to inaccuracies and low performance.
To improve ML model output accuracy, they need to be retrained constantly. Insurers must also ensure accurate, complete and up-to-date data for optimal results, while ensuring fairness in the models.
The Future
As AI continues to evolve, it is expected to become more powerful in detecting and preventing complex fraud schemes in phone insurance. One promising development is the use of deep learning models that can instantly cross-reference a new insurance claim against millions of previous claims, checking for suspicious patterns such as unusual damage characteristics, repetitive claim submissions, geolocation inconsistencies, or behavioral red flags that might indicate potentially fraudulent activity.
These models do not just follow predefined rules; they learn and adapt continuously, becoming more sophisticated with each new piece of data they process. As a result they can analyze visual evidence of phone damage, compare it with extensive databases, detect potential image manipulations, and assess the likelihood of fraudulent intent with remarkable accuracy.
The integration of Internet of Things (IoT) will further bolster fraud prevention efforts by connecting multiple data sources from smartphones, wearables, and other devices. Through these, insurers will gain real-time insights into device usage, location, and potential anomalies.
Moreover, collaboration platforms are being developed to allow insurers to share anonymized fraud data, making it easier to identify repeat offenders and stay ahead of new tactics. Advancements like these will make phone insurance systems more secure and efficient, benefiting both insurers and customers.